本文主要是介绍GoodPoint: unsupervised learning of keypoint detection and description∗,希望对大家解决编程问题提供一定的参考价值,需要的开发者们随着小编来一起学习吧!
主要提供了一种无监督的deep feature的提取方式
good point应该满足:
- they should be distributed more or less evenly throughout the image;
- have good repeatability between different view- points;
- be recognizable and distinguishable with descrip- tors;
- should not lie too densely.
可以认为是在superpoint上的改进
网络架构:
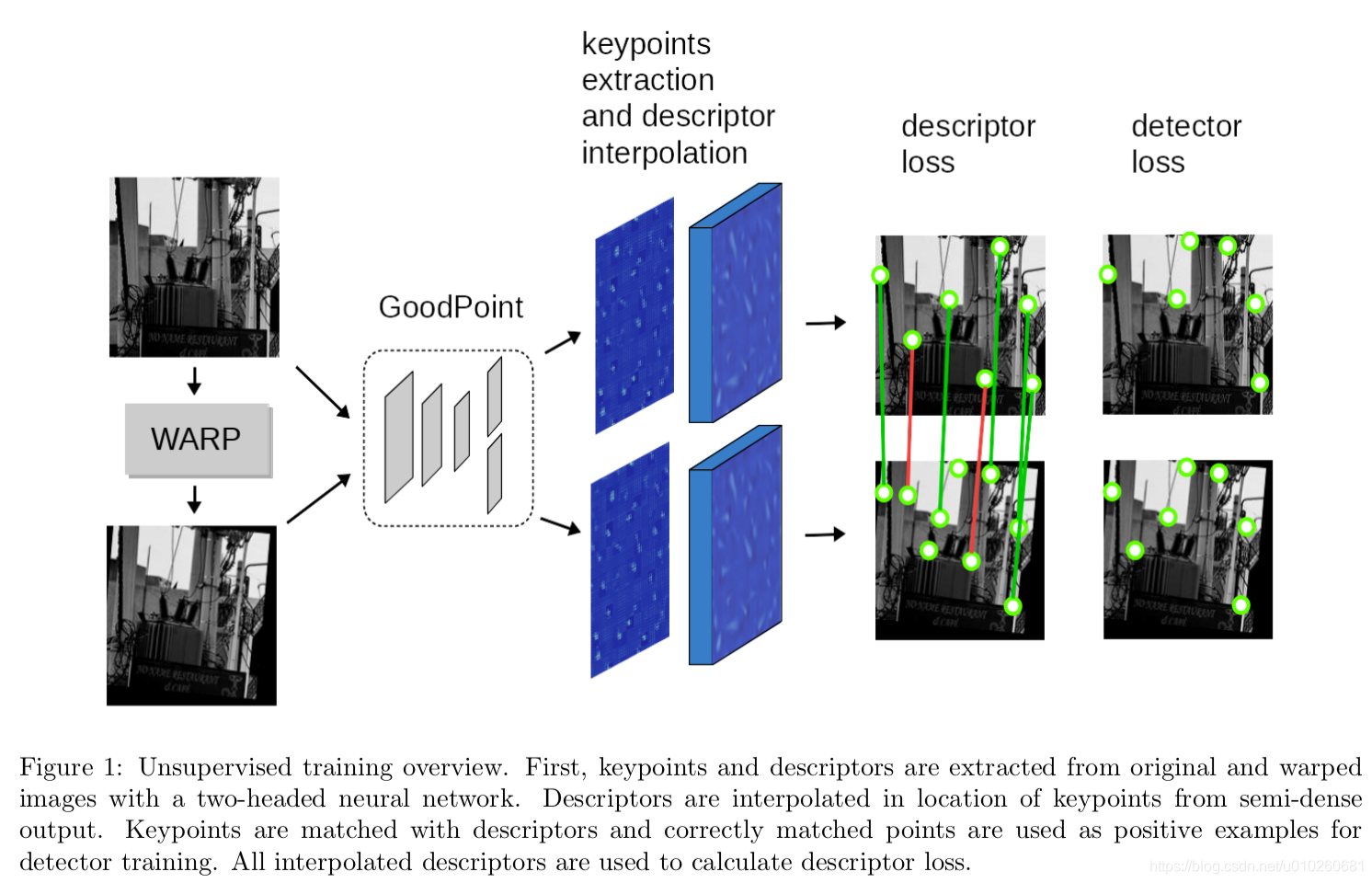
train:
loss:
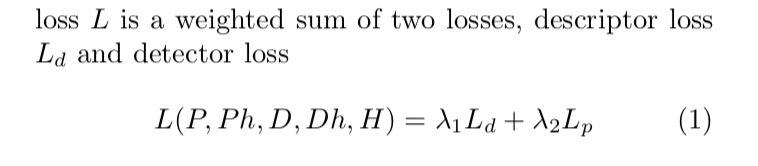

首先构造gt(使用随机homograph+随机噪声派生出图像)
4.1 Keypoints loss
为32×32或16×16大小的每个区域选择一个关键点是基于这样的假设:关键点应该在整个图像中均匀分布,但不要太密集。
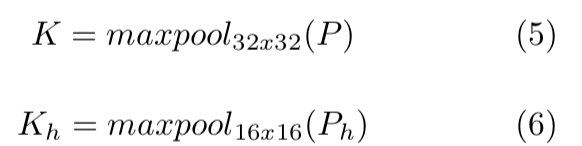
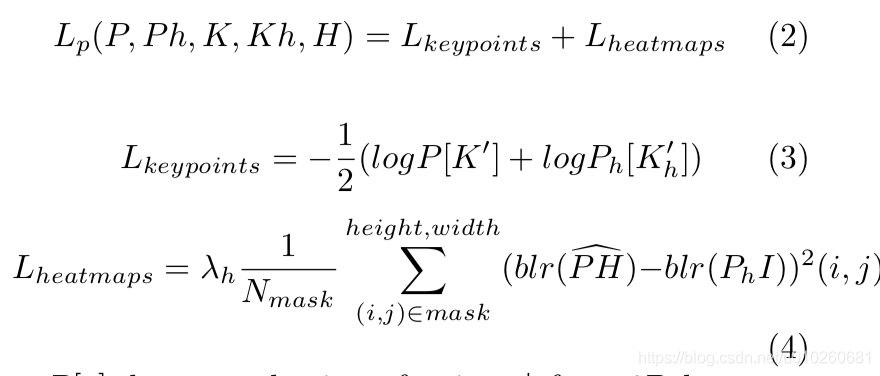
其中Lkeypoints 的loss 是这样计算:
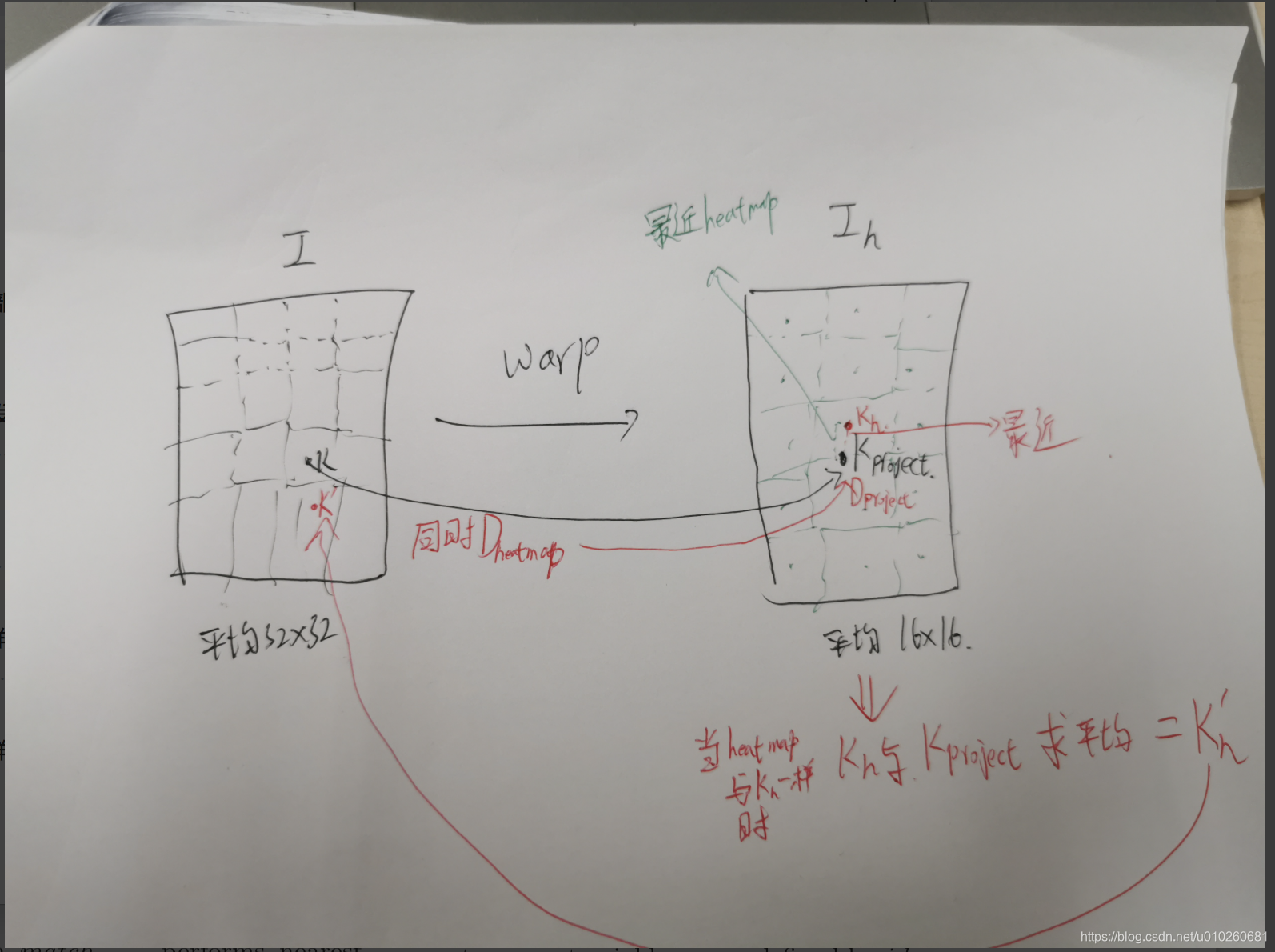
如此自适应的解决detector问题( 但是只是经过homograph没有办法解决金字塔呀?除非train数据中存在scale的大量的变化)
4.2 Descriptor loss
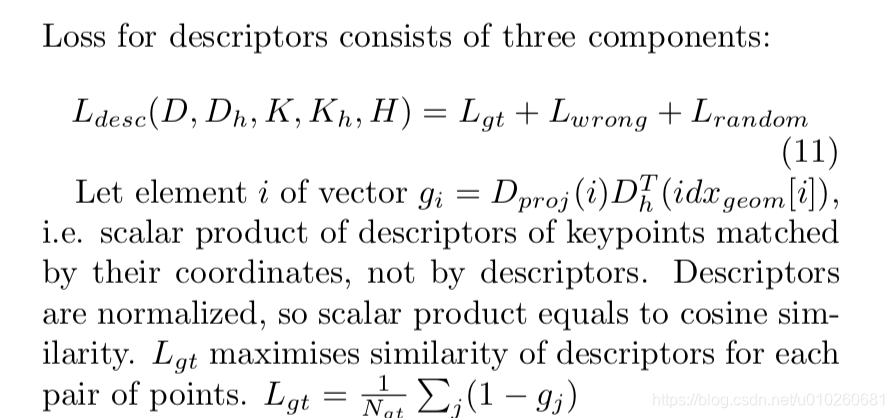
所以Lgt表示的是detector层的heatmap的差异。
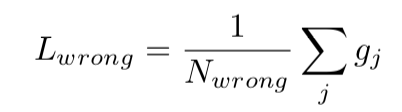

结果:
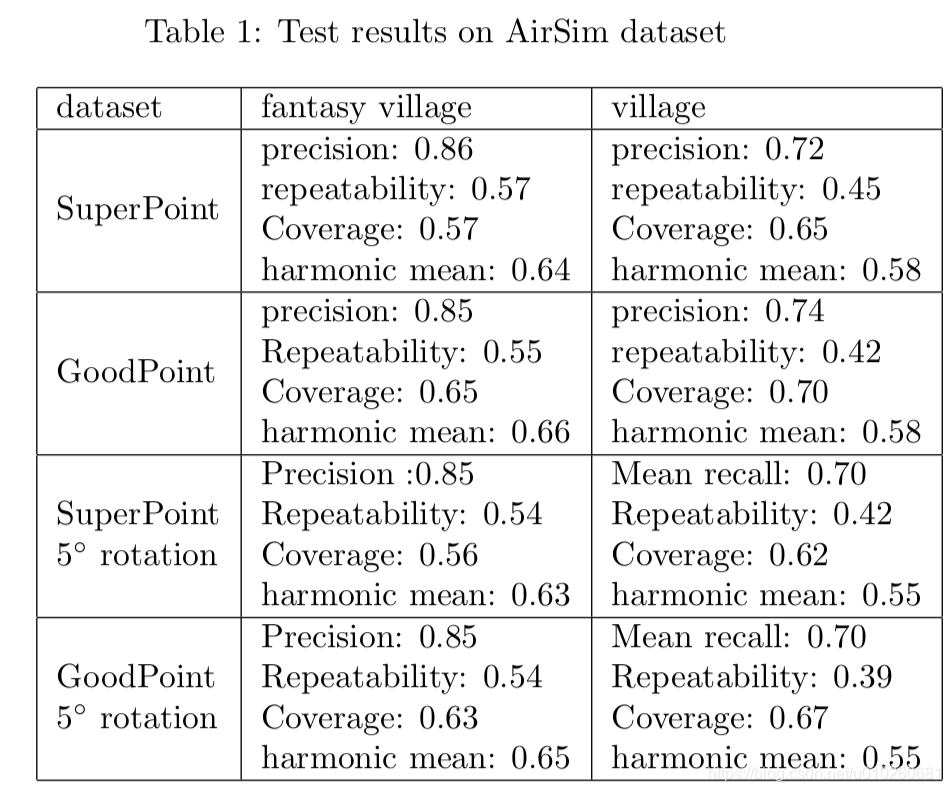
比较superpoint提升不大 可能是比较新颖的不用标注数据吧
持续关注视觉定位相关论文,感兴趣➕关注
这篇关于GoodPoint: unsupervised learning of keypoint detection and description∗的文章就介绍到这儿,希望我们推荐的文章对编程师们有所帮助!