本文主要是介绍2020-ASRM: A Semantic and Attention Spatio-temporal Recurrent Model for Next Location Prediction,希望对大家解决编程问题提供一定的参考价值,需要的开发者们随着小编来一起学习吧!
[1] Zhang X, Li B, Song C, et al. SASRM: A Semantic and Attention Spatio-temporal Recurrent Model for Next Location Prediction[C]//2020 International Joint Conference on Neural Networks (IJCNN). IEEE, 2020: 1-8.
文章目录
- `Abstract`
- `关键词`
- `1 Introduction`
- `2 Related Work`
- `3 Preliminaries`
- `4 Semantic and Attention Spatio-temporal Recurrent Model`
- `A 特征嵌入和向量生成`
- `B 递归单元`
- `C 注意力`
- `D 预测`
- `5 Experiments`
- `6 Conclusions`
Abstract
Predicting user’s next location is of great importance for a wide spectrum of location-based applications. However, most prediction methods do not take advantage of the rich semantic information contained in trajectory data. Meanwhile, the traditional LSTM-based model can not capture the spatio-temporal dependencies well. In this paper, we propose a Semantic and Attention Spatio-temporal Recurrent Model (SASRM) for next location prediction. Firstly, the SASRM put forward a method for encoding semantic vectors and concatenating vectors (location, time and semantic vectors) as input to the model. To capture the spatio-temporal dependencies, we design a variant recurrent unit based on LSTM. Further, an attention layer is used to weight hidden state to capture the influence of the historical locations on the next location prediction. We perform experiments on two real-life semantic trajectory datasets, and evaluation results demonstrate that our model outperforms several state-of-the-art models in accuracy.
- 现有问题:1. 大多数预测方法并
没有充分利用
轨迹数据中丰富的语义信息
。2. 传统的基于LSTM的模型不能很好地捕捉时空相关性
。 - 本文提出了一种语义和注意力时空循环模型(Semantic and Attention Spatio-temporal Recurrent Model,
SASRM
)用于下一个位置预测
。 - 方法(3个关键点):首先,
对语义向量进行编码
,并将向量(位置、时间和语义向量)连接起来作为模型的输入。为了捕捉时空相关性,我们设计了一个基于LSTM的变分递归单元
。在此基础上,利用注意力层
对隐藏状态进行加权,以获取历史位置对下一个位置预测的影响。 - 结果:在两个真实生活中的语义轨迹数据集上进行了实验,结果表明SASRM模型在accuracy上优于一些最先进的模型。
关键词
- Location Prediction
- Semantic Trajectory
- Attention
- LSTM
1 Introduction
- 具有丰富的时空语境信息的人的移动轨迹称为
语义轨迹
。 语义信息
: 例如位置上下文信息(例如餐馆、书店),正在进行的活动上下文信息(打篮球、跳舞、唱歌)。基于语义轨迹的位置预测
的三个挑战及本文的解决方法
:1)如何将语义信息编码到预测模型中——解决方法:语义向量
。2)如何有效地捕捉轨迹的时空相关性——解决方法:在LSTM的基础上设计变分递归单元(包含time gate和distance gate)
。3)如何将历史信息融入回归式模型训练中——解决方法:注意力机制
。
2 Related Work
介绍了传统的预测模型
和基于神经网络的模型
传统的预测模型
(HMM、MF…)基于神经网络的模型
(Liu等人提出了ST-RNN (Spatial Temporal RNN)模型,利用RNN单元的时间特异性转移矩阵和距离特异性转移矩阵来增强模型接收时空信息的能力。Zhu等人生成了Time-LSTM模型,用于学习时间间隔的依赖关系。Zhao等人在Time-LSTM的基础上提出了STGN模型。他们设计了一个基于LSTM单元的门回归网络,通过添加时间门和距离门,提高了LSTM模型捕捉时空相关性的能力。…)不足
:很少考虑语义、没有捕获到时空依赖性
3 Preliminaries
语义地点(Semantic Location)
与通常的地点表示类似,加上了文本信息。 r k u i = ( u i , l k , t k , c k ) {r_k}^{u_i} = (u_i, l_k, t_k, c_k) rkui=(ui,lk,tk,ck), 其中 u i {u_i} ui表示User ID, l k {l_k} lk表示location, t k {t_k} tk表示time, c k {c_k} ck表示由LBS或用户提供的文本
,包含了语义信息
。语义轨迹(Semantic Trajectory)
:由语义地点构成的序列LSTM知识
如下:-
LSTM是RNN的经典变体,用来解决RNN模型的梯度消失问题。与RNN相比,它能够捕捉序列信息的长期依赖性,具有良好的可扩展性。我们可以修改LSTM来提高模型在不同预测任务中的性能。
原LSTM公式见(1)-(6)。
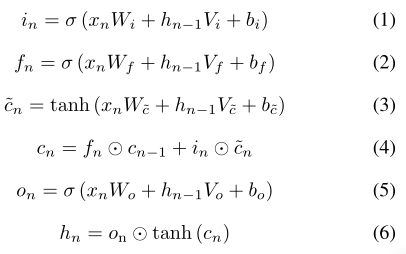
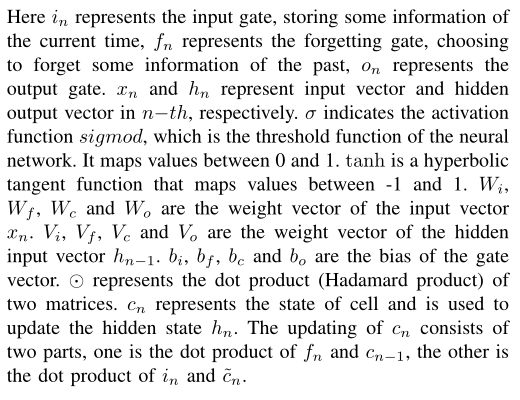
4 Semantic and Attention Spatio-temporal Recurrent Model
语义和注意力时空循环模型(Semantic and Attention Spatio-temporal Recurrent Model,
SASRM
)
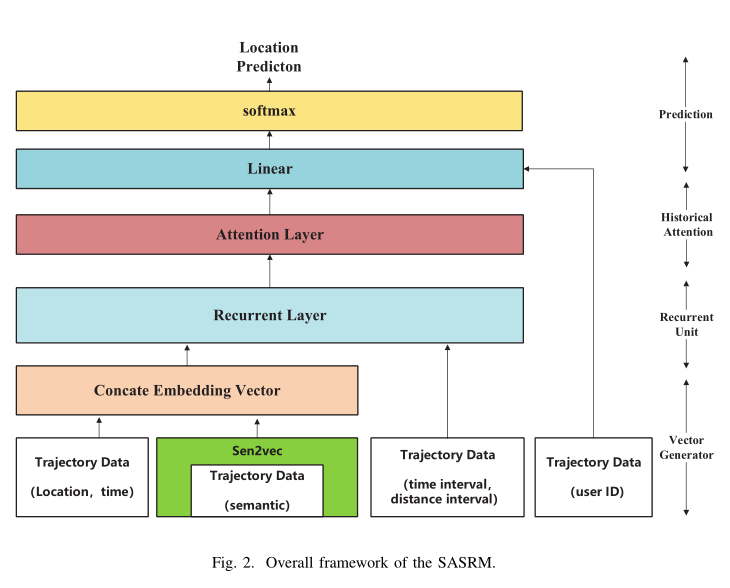
SASRM模型包括四个主要部分
:
- 特征嵌入和向量生成(feature embedding and vector generator)
- 递归单元(recurrent unit)
- 注意力(historical attention)
- 预测(prediction)
A 特征嵌入和向量生成
- 通过嵌入(
embedding
)技术生成向量 - u i {u_i} ui表示User ID, l k {l_k} lk表示location, t k {t_k} tk表示time。 u i {u_i} ui、 l k {l_k} lk、 t k {t_k} tk可以
直接编码
为嵌入向量 b u i {b_{u_i}} bui、 b l k {b_{l_k}} blk、 b t k {b_{t_k}} btk。三个嵌入权重矩阵 B i {B_i} Bi、 B k {B_k} Bk、 B k {B_k} Bk被随机初始化。初始化公式如(7)-(9).
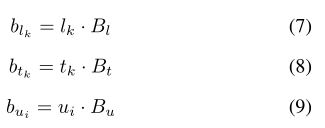
- 采用一种
语义信息向量嵌入方式sen2vec
编码语义向量
- 对于一个包含语义的文本信息 c k c_k ck,我们使用预先训练的词向量 v g k v_{g_k} vgk来表示每个词w。首先,所有词向量
这篇关于2020-ASRM: A Semantic and Attention Spatio-temporal Recurrent Model for Next Location Prediction的文章就介绍到这儿,希望我们推荐的文章对编程师们有所帮助!