本文主要是介绍[NNLM]论文实现:A Neural Probabilistic Language Model [Yoshua Bengio, Rejean Ducharme, Pascal Vincent],希望对大家解决编程问题提供一定的参考价值,需要的开发者们随着小编来一起学习吧!
A Neural Probabilistic Language Model
- 一、完整代码
- 1.1 Python 完整程序
- 二、论文解读
- 2.1 目标
- 三、过程实现
- 3.1 Tensorflow模型
- 3.2 数据准备
- 3.3 数据训练和预测
- 四、整体总结
论文:A Neural Probabilistic Language Model
作者:Yoshua Bengio; Rejean Ducharme and Pascal Vincent
时间:2000
一、完整代码
这篇文献似乎是第一篇词嵌入模型在神经网络上的文献,由于文献比较早,结构比较简单,这里简要介绍一下,并使用Tensorflow实现.
1.1 Python 完整程序
# tf.__version__ == 2.10.1
import tensorflow as tf
import numpy as np
import pandas as pd## 建立词表
s = '东胜神洲傲来国海边有一花果山,山顶一石,受日月精华,产下一个石猴,石猴勇探瀑布飞泉,发现水帘洞,被众猴奉为美猴王,猴王领群猴在山中自由自在数百载,偶闻仙、佛、神圣三者可躲过轮回,与天地山川齐寿,遂独自乘筏泛海,历南赡部洲,至西牛贺洲,终在灵台方寸山斜月三星洞,为菩提祖师收留,赐其法名孙悟空,悟空在三星洞悟彻菩提妙理,学到七十二般变化和筋斗云之术后返回花果山,一举灭妖魔混世魔王,花果山狼、虫、虎、豹等七十二洞妖王都来奉其为尊'vocabulary = list(set(list(s)))
n = 5
m = len(vocabulary)data_list = []
for i in range(len(s)-n):data_list.append([s[i:i+n], s[i+n]])## 准备数据
## [['东胜神洲傲', '来'], ['胜神洲傲来', '国'], ['神洲傲来国', '海']]x_train = np.array(data_list)[:,0]
y_train = np.array(data_list)[:,1]def get_one_hot(lst):one_hot_list = []for item in lst:one_hot = [0] * len(vocabulary)ix = vocabulary.index(item)one_hot[ix] = 1one_hot_list.append(one_hot)return one_hot_listx_train = [get_one_hot(item) for item in x_train]
y_train = [vocabulary.index(item) for item in y_train]## 建立模型
class Embedding(tf.keras.layers.Layer):def __init__(self, out_shape, **kwargs):super().__init__(**kwargs)self.out_shape = out_shapedef build(self, input_shape):self.H = self.add_weight(shape=[input_shape[-1], self.out_shape],initializer=tf.initializers.glorot_normal(),)def call(self, inputs):return tf.matmul(inputs, self.H)model = tf.keras.Sequential([tf.keras.layers.Input(shape=(n, m)),Embedding(200),tf.keras.layers.Flatten(),tf.keras.layers.Dense(200, activation='tanh'),tf.keras.layers.Dense(m, activation='softmax'),
])model.compile(loss=tf.keras.losses.SparseCategoricalCrossentropy(), metrics='accuracy')
history = model.fit(x=x_train, y=y_train, epochs=100, verbose=0)
pd.DataFrame(history.history).plot()## 预测模型
s = '边有一花果'
vocabulary[model.predict([get_one_hot(s)])[0].argmax()]
# '山'
二、论文解读
2.1 目标
这篇论文的目的是:已知一段文本序列,求文本序列下一个词出现的概率,这里我们很容易就想到一个概率公式 P ( x n ∣ x n − 1 , x n − 2 , … , x 1 ) P(x_n|x_{n-1},x_{n-2},\dots,x_1) P(xn∣xn−1,xn−2,…,x1).虽然用这个公式从现在看来有很多的毛病,但是要考虑到这是一篇2000年的论文.
三、过程实现
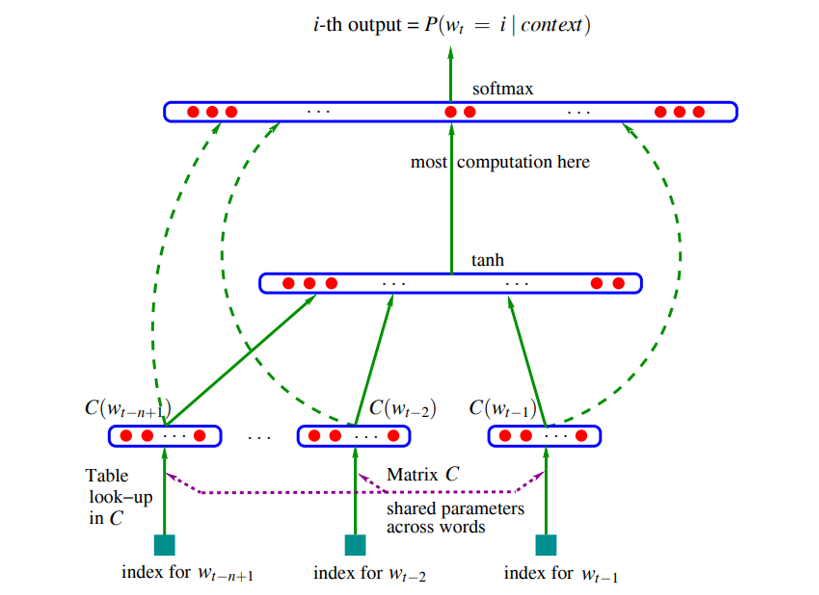
3.1 Tensorflow模型
n = 预测句子长度
m = 词表维度
class Embedding(tf.keras.layers.Layer):def __init__(self, out_shape, **kwargs):super().__init__(**kwargs)self.out_shape = out_shapedef build(self, input_shape):self.H = self.add_weight(shape=[input_shape[-1], self.out_shape],initializer=tf.initializers.glorot_normal(),)def call(self, inputs):return tf.matmul(inputs, self.H)model = tf.keras.Sequential([tf.keras.layers.Input(shape=(n, m)),Embedding(200),tf.keras.layers.Flatten(),tf.keras.layers.Dense(200, activation='tanh'),tf.keras.layers.Dense(m, activation='softmax'),
])
3.2 数据准备
从西游记里面选了一段文字,准备数据 input_shape=[n,m]
s = '东胜神洲傲来国海边有一花果山,山顶一石,受日月精华,产下一个石猴,石猴勇探瀑布飞泉,发现水帘洞,被众猴奉为美猴王,猴王领群猴在山中自由自在数百载,偶闻仙、佛、神圣三者可躲过轮回,与天地山川齐寿,遂独自乘筏泛海,历南赡部洲,至西牛贺洲,终在灵台方寸山斜月三星洞,为菩提祖师收留,赐其法名孙悟空,悟空在三星洞悟彻菩提妙理,学到七十二般变化和筋斗云之术后返回花果山,一举灭妖魔混世魔王,花果山狼、虫、虎、豹等七十二洞妖王都来奉其为尊'vocabulary = list(set(list(s)))
n = 5
m = len(vocabulary)data_list = []
for i in range(len(s)-n):data_list.append([s[i:i+n], s[i+n]])x_train = np.array(data_list)[:,0]
y_train = np.array(data_list)[:,1]def get_one_hot(lst):one_hot_list = []for item in lst:one_hot = [0] * len(vocabulary)ix = vocabulary.index(item)one_hot[ix] = 1one_hot_list.append(one_hot)return one_hot_listx_train = [get_one_hot(item) for item in x_train]
y_train = [vocabulary.index(item) for item in y_train]
3.3 数据训练和预测
model.compile(loss=tf.keras.losses.SparseCategoricalCrossentropy(), metrics='accuracy')
history = model.fit(x=x_train, y=y_train, epochs=100, verbose=0)
pd.DataFrame(history.history).plot()s = '边有一花果'
vocabulary[model.predict([get_one_hot(s)])[0].argmax()]
# 输出山
应该为山,预测结果与实际一致.
训练loss和accuracy如下:
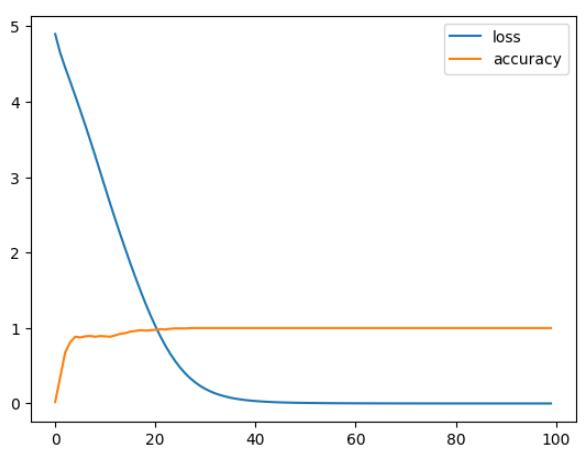
数据比较小,很好训练
四、整体总结
论文太早了,实现没难度!
这篇关于[NNLM]论文实现:A Neural Probabilistic Language Model [Yoshua Bengio, Rejean Ducharme, Pascal Vincent]的文章就介绍到这儿,希望我们推荐的文章对编程师们有所帮助!