本文主要是介绍PVT(Pyramid Vision Transformer)算法整理,希望对大家解决编程问题提供一定的参考价值,需要的开发者们随着小编来一起学习吧!
整体架构
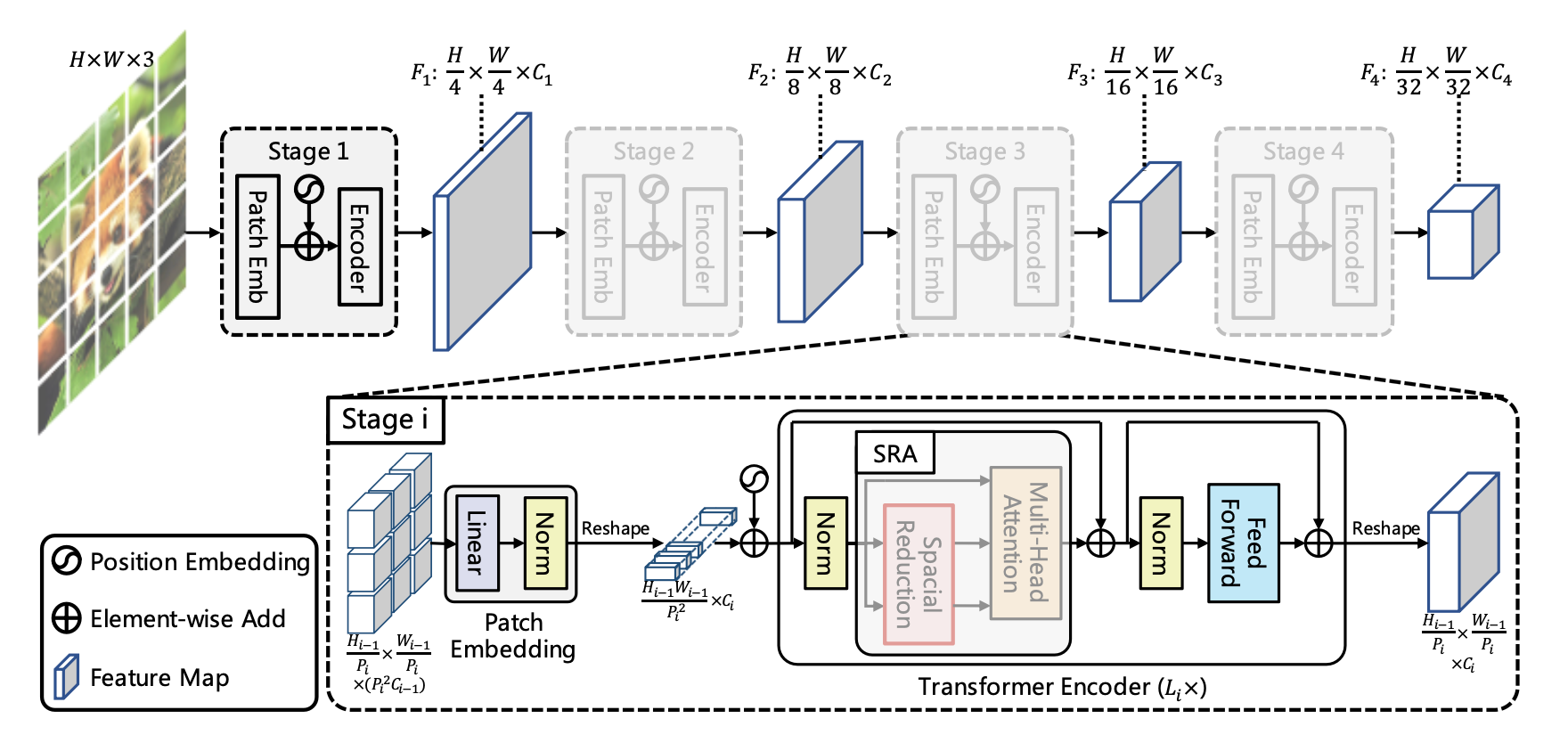
整个“金字塔”架构分为4个stage,每个stage内的基础结构是相似的,即:
- Patch Embedding:目的在于将信息分块,降低单张图的图片大小,但会增加数据的深度
- Transformer Encoder:目的在于计算图片的attention value,由于深度变大了,计算复杂度会变大,所以在这里作者使用了Special Reduction来减小计算复杂度
具体模块
Patch Embedding
Patch Embedding部分与ViT中对与图片的分块操作是一样的,即:
- 将原图切成总数为 p i × p i p_i\times p_i pi×pi的patches
具体操作:
使用卷积操作, k e r n a l _ s i z e = H p i , s t r i d e = H p i \mathrm{kernal\_size}=\frac{H}{p_i},\mathrm{stride}=\frac{H}{p_i} kernal_size=piH,stride=piH
- 将每个patch内的数据拉平,然后进行LayerNorm,此时每个patch内的数据大小为 H i − 1 W i − 1 p i 2 × C i \frac{H_{i-1}W_{i-1}}{p_i^2}\times C_i pi2Hi−1Wi−1×Ci
Transformer Encoder
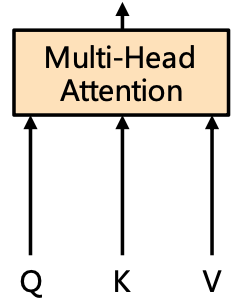
Q = W q x + b q K = W k x + b k V = W v x + b v A t t e n t i o n ( Q , K , V ) = S o f t m a x ( Q K T d h e a d ) V Q=W_qx+b_q\quad K=W_kx+b_k\quad V=W_vx+b_v\\ \mathrm{Attention}(Q,K,V)=\mathrm{Softmax}(\frac{QK^T}{\sqrt{d_{head}}})V Q=Wqx+bqK=Wkx+bkV=Wvx+bvAttention(Q,K,V)=Softmax(dheadQKT)V
假设输入( x x x)的大小为 H W × C HW\times C HW×C,则 Q . s h a p e = K . s h a p e = V . s h a p e = H W × C ′ Q.\mathrm{shape}=K.\mathrm{shape}=V.\mathrm{shape}=HW\times C' Q.shape=K.shape=V.shape=HW×C′
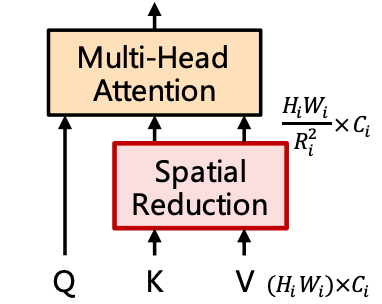
S R A ( Q , K , V ) = C o n c a t ( h e a d 0 , . . . h e a d N i ) W o , ( h e a d j = A t t e n t i o n ( Q W j Q , S R ( K ) W j K , S R ( V ) W j V ) ) S R ( x ) = N o r m ( R e s h a p e ( x , R i ) W S ) A t t e n t i o n ( Q , K , V ) = S o f t m a x ( Q K T d h e a d ) V \mathrm{SRA}(Q,K,V)=\mathrm{Concat}(head_0,...head_{N_i})W^o,\\ (head_j=\mathrm{Attention}(QW_j^Q,\mathrm{SR}(K)W_j^K,\mathrm{SR}(V)W_j^V))\\ \mathrm{SR}(x)=\mathrm{Norm(Reshape}(x,R^i)W^S)\\ \mathrm{Attention}(Q,K,V)=\mathrm{Softmax}(\frac{QK^T}{\sqrt{d_{head}}})V SRA(Q,K,V)=Concat(head0,...headNi)Wo,(headj=Attention(QWjQ,SR(K)WjK,SR(V)WjV))SR(x)=Norm(Reshape(x,Ri)WS)Attention(Q,K,V)=Softmax(dheadQKT)V
假设输入( x x x)的大小为 H W × C HW\times C HW×C,则 Q . s h a p e = H W × C ′ , K . s h a p e = V . s h a p e = H W R i 2 × C ′ Q.\mathrm{shape}=HW\times C',K.\mathrm{shape}=V.\mathrm{shape}=\frac{HW}{R_i^2}\times C' Q.shape=HW×C′,K.shape=V.shape=Ri2HW×C′,可以看出,使用这个方法之后,最终得到的结果的维度不变,但计算Attention的值时,参数的数量减少了很多
代码链接
https://github.com/whai362/PVT
这篇关于PVT(Pyramid Vision Transformer)算法整理的文章就介绍到这儿,希望我们推荐的文章对编程师们有所帮助!