本文主要是介绍使用DMAD(Learning Efficient GANs using Differentiable Masks and co-Attention Distillation)训练并测试自己的数据,希望对大家解决编程问题提供一定的参考价值,需要的开发者们随着小编来一起学习吧!
论文:Learning Efficient GANs using Differentiable Masks and co-Attention Distillation
代码:DMAD
最近在做毕设,翻GitHub时看到原作者的repo,就尝试拿来跑一下自己的数据。结果一上来就报错(除了一些通用性比较高的repo外,很多都会遇到这种问题),解决了半天的环境问题,遇到下面这个错误:
/home/wlw19/miniconda3/lib/python3.8/site-packages/torchvision/transforms/transforms.py:257: UserWarning: Argument interpolation should be of type InterpolationMode instead of int. Please, use InterpolationMode enum.warnings.warn(
/home/wlw19/miniconda3/lib/python3.8/site-packages/torchvision/models/inception.py:80: FutureWarning: The default weight initialization of inception_v3 will be changed in future releases of torchvision. If you wish to keep the old behavior (which leads to long initialization times due to scipy/scipy#11299), please set init_weights=True.warnings.warn('The default weight initialization of inception_v3 will be changed in future releases of '
Traceback (most recent call last):File "train.py", line 278, in <module>test(model, opt, logger, total_iters, best_AtoB_fid, best_BtoA_fid, best_AtoB_epoch,File "train.py", line 173, in testfid = test_pix2pix_fid(model, copy.copy(opt))File "train.py", line 92, in test_pix2pix_fidfid = get_fid(list(fake_B.values()), inception_model, npz, model.device, opt.batch_size)File "/media/wlw19/Elements/415_experiments/WH/code/DMAD-master/metric/__init__.py", line 9, in get_fidm1, s1 = npz['mu'], npz['sigma']File "/home/wlw19/.local/lib/python3.8/site-packages/numpy/lib/npyio.py", line 259, in __getitem__raise KeyError("%s is not a file in the archive" % key)
KeyError: 'mu is not a file in the archive'
刚开始我没有看源码,对这个错误不明所以,看了后才知道:原作者是一边训练数据、训练一次后就测试FID,并把FID值最低的模型权重的epoch数、FID值都保存下来,报错代码的m2, s1就是用来测试FID的。但我用自己的数据所生成的npz文件没有这两个标签,所以报错。(我写了个脚本,测了一下自己数据生成的 npz ,只有一个vol标签)
反正,报错信息是与计算FID有关的,那我换种方式计算FID就行了。正好源码的 metric 文件夹下 fid_score.py就是用来计算FID的,而且提供了图片路径作为参数计算FID的函数 calculate_fid_given_paths。那么,直接把train.py相应的代码注释掉,换成calculate_fid_given_paths来计算FID就可以了。
训练、测试跑完后,发现这个repo的pix2pix并不适合我的数据,弃用。如图1所示,Attention 1和 Attention 2是我自己的方法。
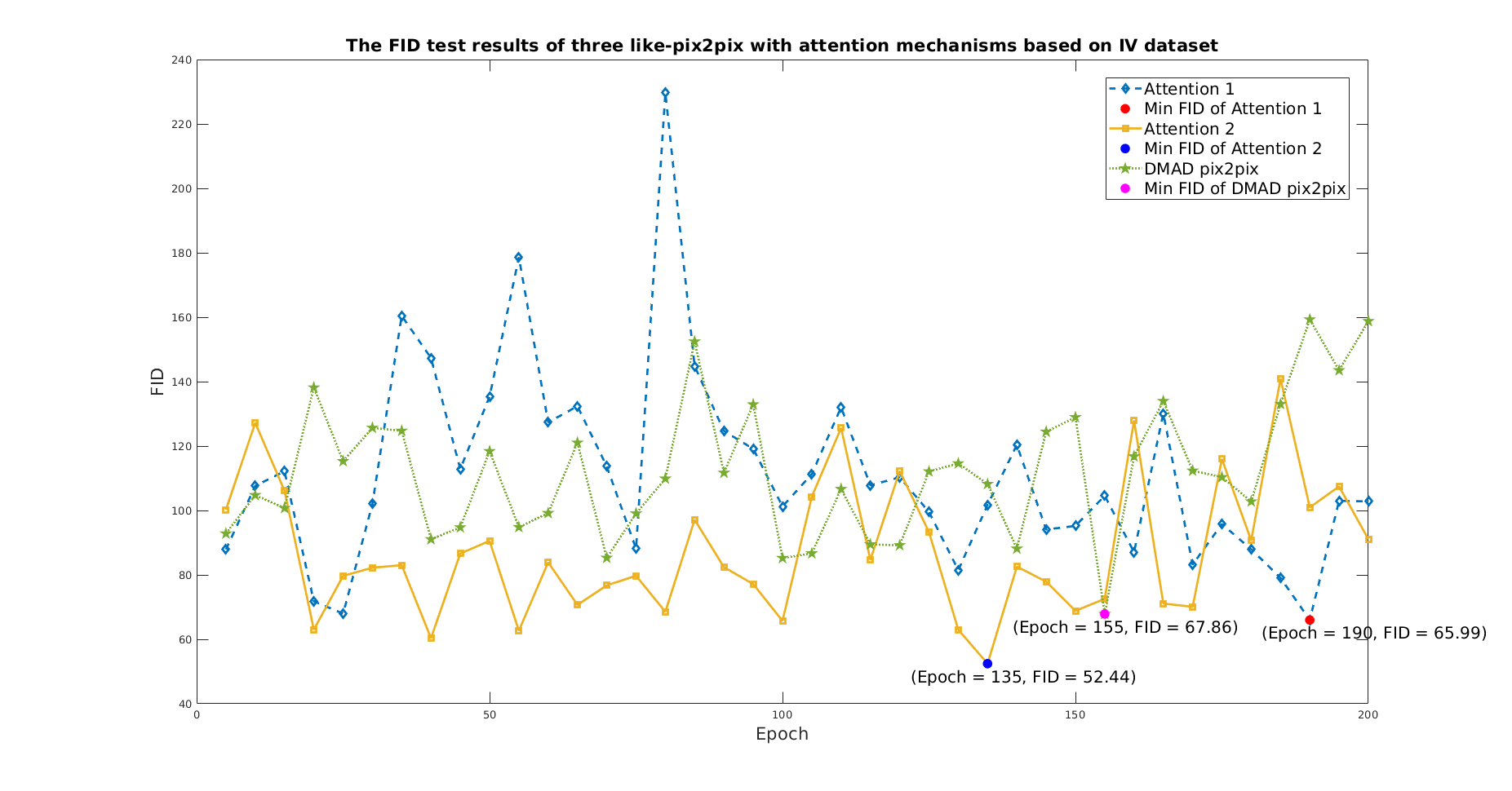
数据集的结构与pix2pix的一样,如图2所示。
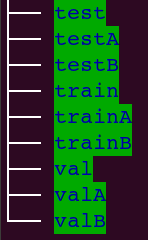
简单修改使之可以跑自己的数据:GitHub链接
这篇关于使用DMAD(Learning Efficient GANs using Differentiable Masks and co-Attention Distillation)训练并测试自己的数据的文章就介绍到这儿,希望我们推荐的文章对编程师们有所帮助!