本文主要是介绍PP-LCNet: A Lightweight CPU Convolutional Neural Network,希望对大家解决编程问题提供一定的参考价值,需要的开发者们随着小编来一起学习吧!
轻量级Trick的优化组合。
论文名称:PP-LCNet: A Lightweight CPU Convolutional Neural Network
作者:Cheng Cui, Tingquan Gao, Shengyu Wei,Yuning Du…
Code:https://github.com/PaddlePaddle/PaddleClas
摘要
- 总结了一些在延迟(latency)几乎不变的情况下精度提高的技术;
- 提出了一种基于MKLDNN加速策略的轻量级CPU网络,即PP-LCNet。

介绍
目前的轻量级网络在启用MKLDNN的Intel CPU上速度并不理想,考虑了一下三个基本问题:
- 如何促使网络学习到更强的特征,但不增加延迟?
- 在CPU上提高轻量级模型精度的要素是什么?
- 如何有效地结合不同的策略来设计CPU上的轻量级模型?
Method
PP-LCNet使用深度可分离卷积作为基础结构,构建了一个类似MobileNetV1的BaseNet,并在其基础上结合现有的技术,从而得到了PP-LCNet
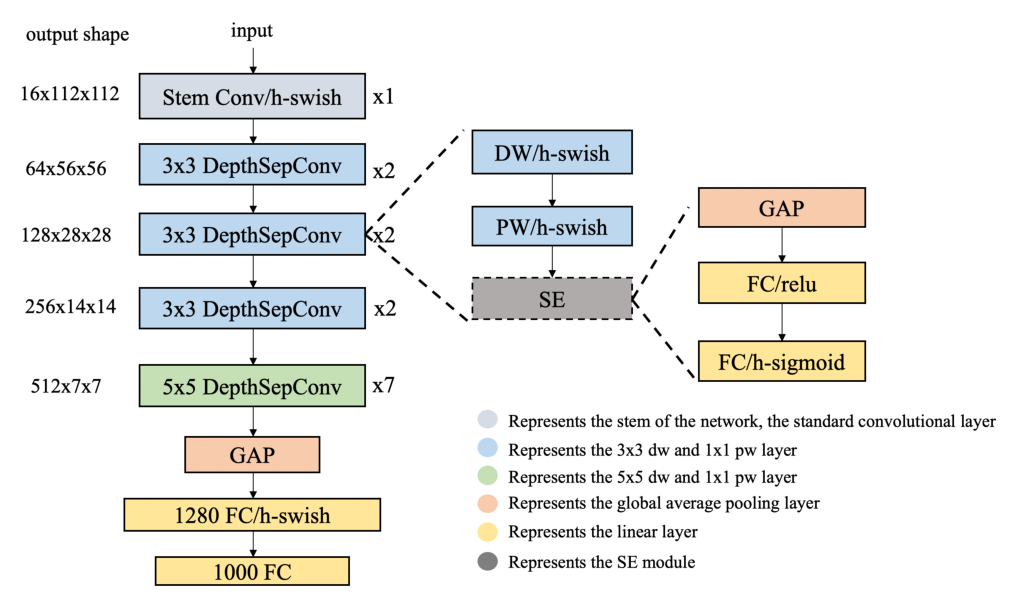
参数配置:
Better activation function
激活函数是神经网络中非线性的来源,因此其质量一定程度上决定着网络的表达能力。
当激活函数由Sigmoid变为ReLU时,网络的性能得到了很大的提升,近来出现了很多超越ReLU的激活函数,如EfficientNet的Swish,MobileNetV3中将其升级为HSwish,避免了大量的指数运算;因此本网络中使用HSwish激活函数代替ReLU。
首先让我们看一下ReLU函数的近似推导:
KaTeX parse error: No such environment: align at position 8: \begin{̲a̲l̲i̲g̲n̲}̲ f(x)&=\sum_{i=…
出于计算量的考虑和实验验证选择了ReLU
ReLU6:
增加了上界

Swish:
f ( x ) = x ⋅ s i g m o i d ( β x ) f(x)=x\cdot sigmoid(\beta x) f(x)=x⋅sigmoid(βx)
β \beta β是个常数或可训练的参数。Swish 具备无上界有下界、平滑、非单调的特性。
Swish 在深层模型上的效果略优于 ReLU。仅仅使用 Swish 单元替换 ReLU 就能把 Mobile NASNetA 在 ImageNet 上的 top-1 分类准确率提高 0.9%,Inception-ResNet-v 的分类准确率提高 0.6%。
导数:

当 β = 0 β = 0 β=0时,Swish变为线性函数 f ( x ) = x 2 f(x)=\frac x 2 f(x)=2x
当 β → ∞ β → ∞ β→∞, σ ( x ) = 1 1 + e − x \sigma(x)=\frac{1}{1+e^{−x}} σ(x)=1+e−x1为0或1,这时Swish变为 R e L U ( x ) = m a x ( 0 , x ) ReLU(x)=max(0,x) ReLU(x)=max(0,x)
因此Swish函数可以看做是介于线性函数与ReLU函数之间的平滑函数.
HSwish:
Swish函数的计算量是很大的,因此提出了HSwish,H表示Hard,意味着超过某个范围,激活值为常数
对ReLU6除以6再向左平移三个单位可以得到HSigmoid:

HSwish的近似公式为 x ⋅ h σ ( x ) = r e l u 6 ( x + 3 ) 6 x\cdot h\sigma(x)=\frac{relu6(x+3)}{6} x⋅hσ(x)=6relu6(x+3),图像如下:
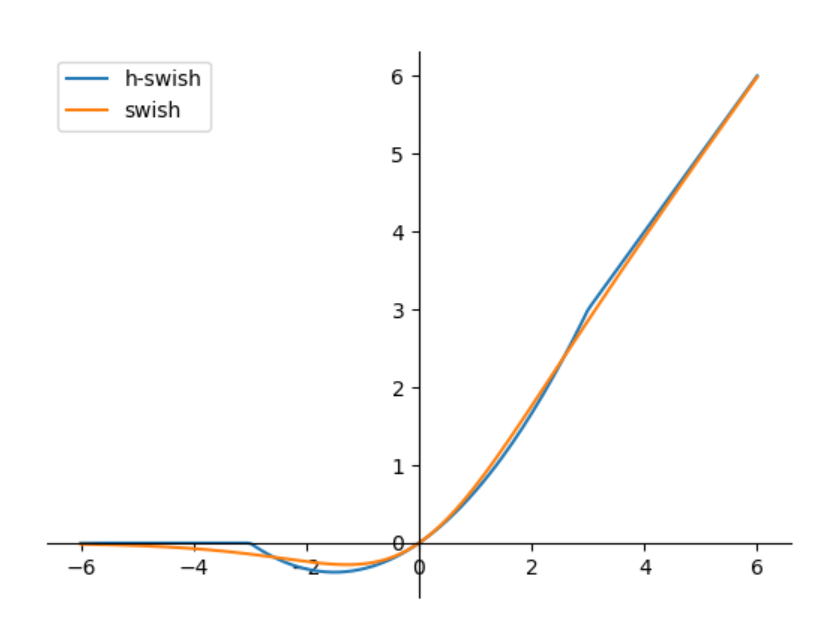
SE modules at appropriate positions
注意力模块无疑是轻量级网络完美的选择,本文探究了SE模块放置的位置,发现在网络深层的效果较好。
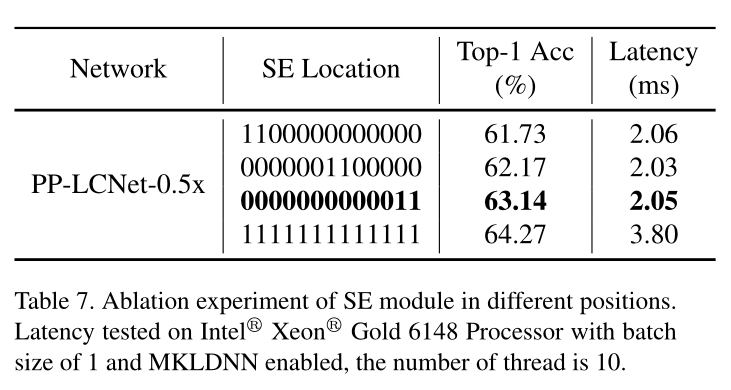
Larger convolution kernels
使用更大的卷积核尺寸,发现在网络深层效果较好
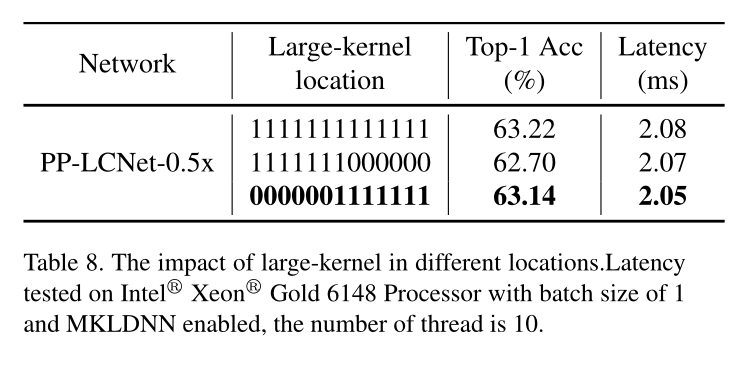
Larger dimensional1×1conv layer after GAP
在网络最后的GAP之后使用Pointwise卷积进行升维,以此提高网络的性能
Drop out
实验发现drop out可以提高性能
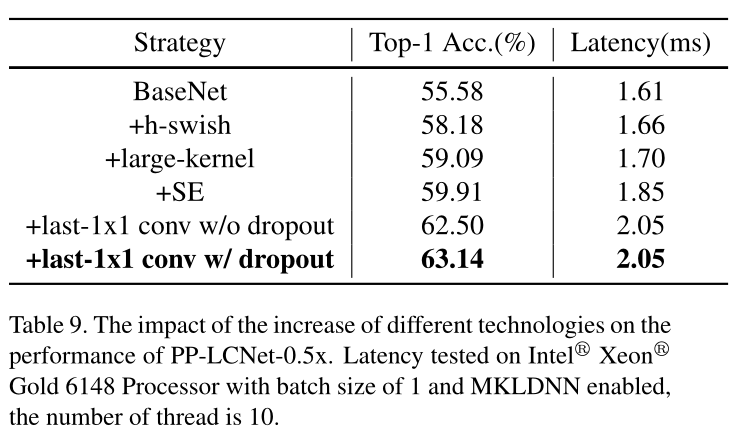
实验结果
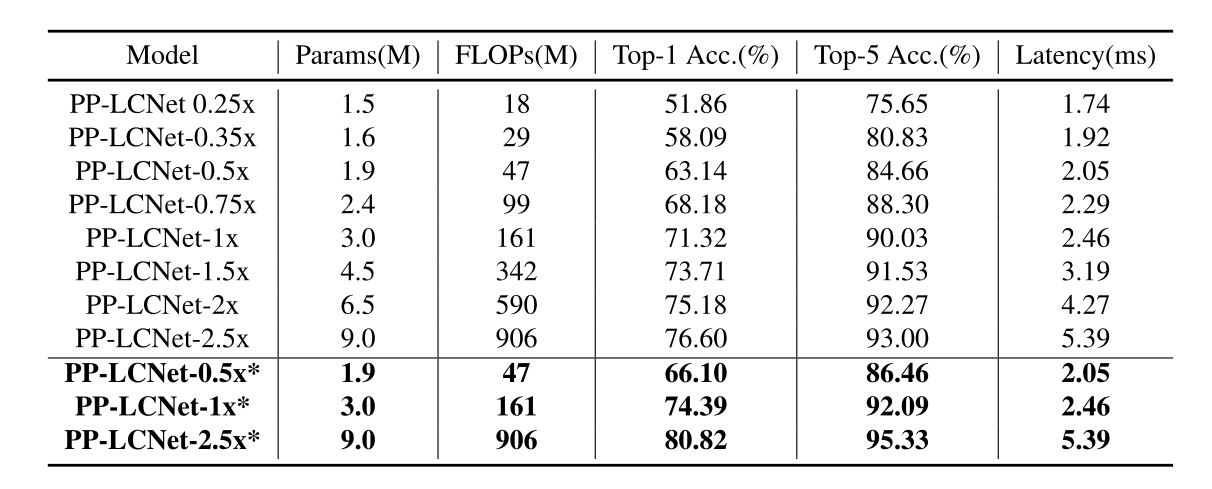
与其他网络进行对比

这篇关于PP-LCNet: A Lightweight CPU Convolutional Neural Network的文章就介绍到这儿,希望我们推荐的文章对编程师们有所帮助!