本文主要是介绍Elasticsearch 高级 RAG 技术第 2 部分:查询和测试,希望对大家解决编程问题提供一定的参考价值,需要的开发者们随着小编来一起学习吧!
作者:来自 Elastic Han Xiang Choong
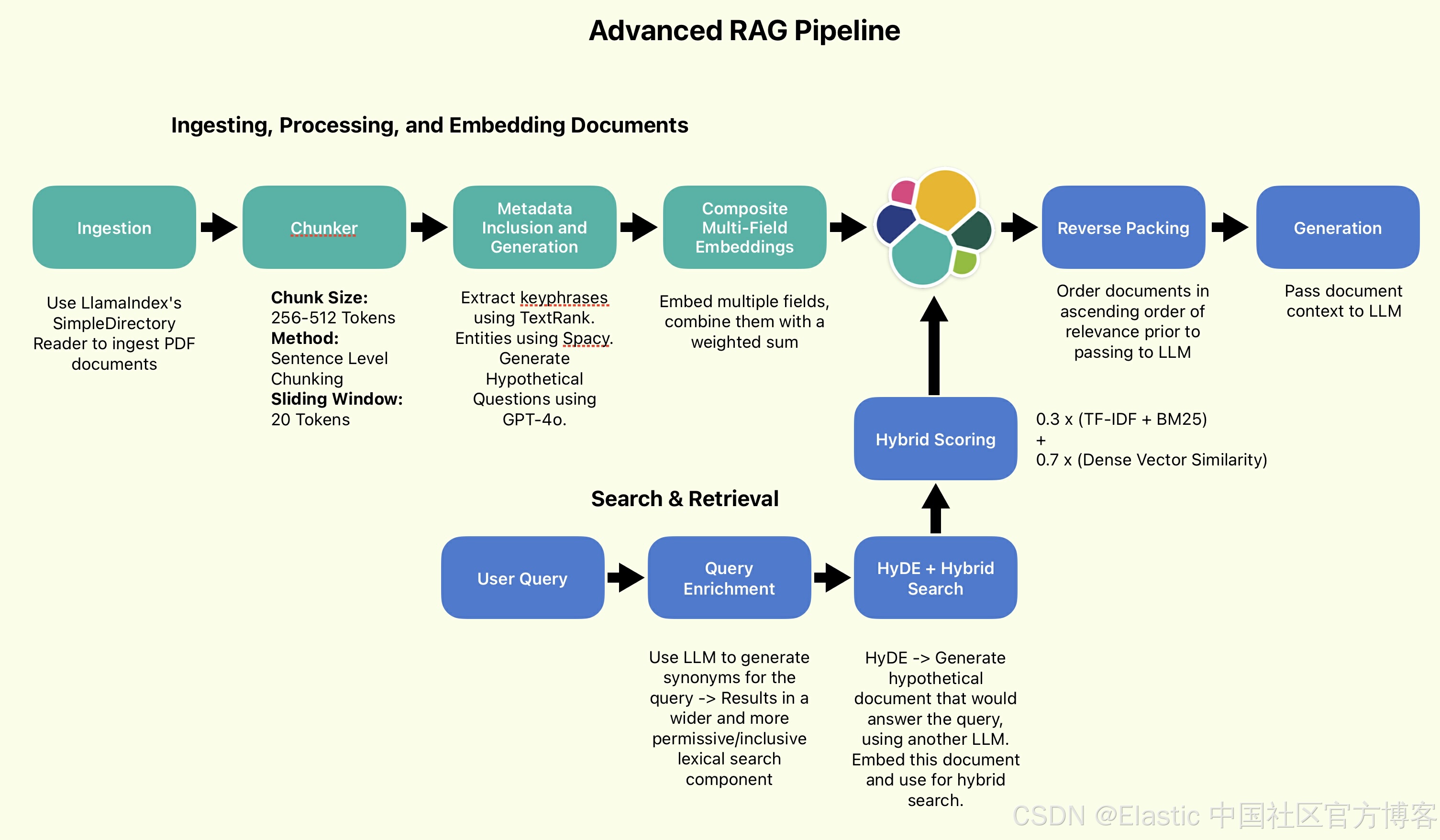
所有代码均可在 Searchlabs repo 的 advanced-rag-techniques 分支中找到。
欢迎阅读我们关于高级 RAG 技术的文章第 2 部分!在本系列的第 1 部分中,我们设置、讨论和实现了高级 RAG 管道的数据处理组件(如上所示)。
在这一部分,我们将继续查询和测试我们的实现。让我们开始吧!
搜索和检索,生成答案
让我们提出第一个查询,最好是主要在年度报告中发现的一些信息。怎么样:
Who audits Elastic?"
现在,让我们应用一些技术来增强查询。
使用同义词丰富查询
首先,让我们增强查询措辞的多样性,并将其转换为可以轻松处理为 Elasticsearch 查询的形式。我们将借助 GPT-4o 将查询转换为 OR 子句列表。让我们写下这个提示:
ELASTIC_SEARCH_QUERY_GENERATOR_PROMPT = '''
You are an AI assistant specialized in generating Elasticsearch query strings. Your task is to create the most effective query string for the given user question. This query string will be used to search for relevant documents in an Elasticsearch index.Guidelines:
1. Analyze the user's question carefully.
2. Generate ONLY a query string suitable for Elasticsearch's match query.
3. Focus on key terms and concepts from the question.
4. Include synonyms or related terms that might be in relevant documents.
5. Use simple Elasticsearch query string syntax if helpful (e.g., OR, AND).
6. Do not use advanced Elasticsearch features or syntax.
7. Do not include any explanations, comments, or additional text.
8. Provide only the query string, nothing else.For the question "What is Clickthrough Data?", we would expect a response like:
clickthrough data OR click-through data OR click through rate OR CTR OR user clicks OR ad clicks OR search engine results OR web analyticsAND operator is not allowed. Use only OR.User Question:
[The user's question will be inserted here]Generate the Elasticsearch query string:
'''
当应用于我们的查询时,GPT-4o 会生成基本查询和相关词汇的同义词。
'audits elastic OR
elasticsearch audits OR
elastic auditor OR
elasticsearch auditor OR
elastic audit firm OR
elastic audit company OR
elastic audit organization OR
elastic audit service'
在 ESQueryMaker 类中,我定义了一个函数来拆分查询:
def parse_or_query(self, query_text: str) -> List[str]:# Split the query by 'OR' and strip whitespace from each term# This converts a string like "term1 OR term2 OR term3" into a list ["term1", "term2", "term3"]return [term.strip() for term in query_text.split(' OR ')]
它的作用是将这一串 OR 子句拆分成一个术语列表,从而让我们对关键文档字段进行多重匹配:
["original_text", 'keyphrases', 'potential_questions', 'entities']
最后以这个查询结束:
'query': {'bool': {'must': [{'multi_match': {'query': 'audits Elastic Elastic auditing Elastic audit process Elastic compliance Elastic security audit Elasticsearch auditing Elasticsearch compliance Elasticsearch security audit','fields': ['original_text','keyphrases','potential_questions','entities'],'type': 'best_fields','operator': 'or'}}]
这比原始查询涵盖了更多的基础,希望可以降低因忘记同义词而错过搜索结果的风险。但我们可以做得更多。
HyDE(Hypothetical Document Embedding - 假设文档嵌入)
让我们再次使用 GPT-4o,这次是为了实现 HyDE。
HyDE 的基本前提是生成一个假设文档 - 这种文档可能包含原始查询的答案。文档的真实性或准确性不是问题。考虑到这一点,让我们写下以下提示:
HYDE_DOCUMENT_GENERATOR_PROMPT = '''
You are an AI assistant specialized in generating hypothetical documents based on user queries. Your task is to create a detailed, factual document that would likely contain the answer to the user's question. This hypothetical document will be used to enhance the retrieval process in a Retrieval-Augmented Generation (RAG) system.Guidelines:
1. Carefully analyze the user's query to understand the topic and the type of information being sought.
2. Generate a hypothetical document that:a. Is directly relevant to the queryb. Contains factual information that would answer the queryc. Includes additional context and related informationd. Uses a formal, informative tone similar to an encyclopedia or textbook entry
3. Structure the document with clear paragraphs, covering different aspects of the topic.
4. Include specific details, examples, or data points that would be relevant to the query.
5. Aim for a document length of 200-300 words.
6. Do not use citations or references, as this is a hypothetical document.
7. Avoid using phrases like "In this document" or "This text discusses" - write as if it's a real, standalone document.
8. Do not mention or refer to the original query in the generated document.
9. Ensure the content is factual and objective, avoiding opinions or speculative information.
10. Output only the generated document, without any additional explanations or meta-text.User Question:
[The user's question will be inserted here]Generate a hypothetical document that would likely contain the answer to this query:
'''
由于向量搜索通常基于余弦向量相似度进行操作,因此 HyDE 的前提是,我们可以通过将文档与文档匹配而不是将查询与文档匹配来获得更好的结果。
我们关心的是结构、流程和术语。事实性不是那么重要。GPT-4o 输出如下 HyDE 文档:
'Elastic N.V., the parent company of Elastic, the organization known for developing Elasticsearch, is subject to audits to ensure financial accuracy, regulatory compliance, and the integrity of its financial statements. The auditing of Elastic N.V. is typically conducted by an external, independent auditing firm. This is common practice for publicly traded companies to provide stakeholders with assurance regarding the company\'s financial position and operations.\n\nThe primary external auditor for Elastic is the audit firm Ernst & Young LLP (EY). Ernst & Young is one of the four largest professional services networks in the world, commonly referred to as the "Big Four" audit firms. These firms handle a substantial number of audits for major corporations around the globe, ensuring adherence to generally accepted accounting principles (GAAP) and international financial reporting standards (IFRS).\n\nThe audit process conducted by EY involves several steps. Initially, the auditors perform a risk assessment to identify areas where misstatements due to error or fraud could occur. They then design audit procedures to test the accuracy and completeness of financial statements,which include examining financial transactions, assessing internal controls, and reviewing compliance with relevant laws and regulations. Upon completion of the audit, Ernst & Young issues an audit report, which includes the auditor’s opinion on whether the financial statements are free from material misstatement and are presented fairly in accordance with the applicable financial reporting framework.\n\nIn addition to external audits by firms like Ernst & Young, Elastic may also be subject to internal audits. Internal audits are performed by the company’s own internal auditors to evaluate the effectiveness of internal controls, risk management, and governance processes.\n\nOverall, the auditing process plays a crucial role in maintaining the transparency and reliability of Elastic\'s financial information, providing confidence to investors, regulators, and other stakeholders.'
它看起来非常可信,就像是我们想要索引的文档类型的理想候选者。我们将它生成嵌入并将其用于混合搜索。
混合搜索
这是我们搜索逻辑的核心。我们的词汇搜索组件将是生成的 OR 子句字符串。我们的密集向量组件将 HyDE 文档生成嵌入(又名搜索向量)。我们使用 KNN 来有效地识别最接近我们的搜索向量的几个候选文档。我们默认使用 TF-IDF 和 BM25 来调用我们的词汇搜索组件评分。最后,词汇和密集向量分数将使用 Wang 等人推荐的 30/70 比例进行组合。
def hybrid_vector_search(self, index_name: str, query_text: str, query_vector: List[float], text_fields: List[str], vector_field: str, num_candidates: int = 100, num_results: int = 10) -> Dict:"""Perform a hybrid search combining text-based and vector-based similarity.Args:index_name (str): The name of the Elasticsearch index to search.query_text (str): The text query string, which may contain 'OR' separated terms.query_vector (List[float]): The query vector for semantic similarity search.text_fields (List[str]): List of text fields to search in the index.vector_field (str): The name of the field containing document vectors.num_candidates (int): Number of candidates to consider in the initial KNN search.num_results (int): Number of final results to return.Returns:Dict: A tuple containing the Elasticsearch response and the search body used."""try:# Parse the query_text into a list of individual search terms# This splits terms separated by 'OR' and removes any leading/trailing whitespacequery_terms = self.parse_or_query(query_text)# Construct the search body for Elasticsearchsearch_body = {# KNN search component for vector similarity"knn": {"field": vector_field, # The field containing document vectors"query_vector": query_vector, # The query vector to compare against"k": num_candidates, # Number of nearest neighbors to retrieve"num_candidates": num_candidates # Number of candidates to consider in the KNN search},"query": {"bool": {# The 'must' clause ensures that matching documents must satisfy this condition# Documents that don't match this clause are excluded from the results"must": [{# Multi-match query to search across multiple text fields"multi_match": {"query": " ".join(query_terms), # Join all query terms into a single space-separated string"fields": text_fields, # List of fields to search in"type": "best_fields", # Use the best matching field for scoring"operator": "or" # Match any of the terms (equivalent to the original OR query)}}],# The 'should' clause boosts relevance but doesn't exclude documents# It's used here to combine vector similarity with text relevance"should": [{# Custom scoring using a script to combine vector and text scores"script_score": {"query": {"match_all": {}}, # Apply this scoring to all documents that matched the 'must' clause"script": {# Script to combine vector similarity and text relevance"source": """# Calculate vector similarity (cosine similarity + 1)# Adding 1 ensures the score is always positivedouble vector_score = cosineSimilarity(params.query_vector, params.vector_field) + 1.0;# Get the text-based relevance score from the multi_match querydouble text_score = _score;# Combine scores: 70% vector similarity, 30% text relevance# This weighting can be adjusted based on the importance of semantic vs keyword matchingreturn 0.7 * vector_score + 0.3 * text_score;""",# Parameters passed to the script"params": {"query_vector": query_vector, # Query vector for similarity calculation"vector_field": vector_field # Field containing document vectors}}}}]}}}# Execute the search request against the Elasticsearch indexresponse = self.conn.search(index=index_name, body=search_body, size=num_results)# Log the successful execution of the search for monitoring and debugginglogger.info(f"Hybrid search executed on index: {index_name} with text query: {query_text}")# Return both the response and the search body (useful for debugging and result analysis)return response, search_bodyexcept Exception as e:# Log any errors that occur during the search processlogger.error(f"Error executing hybrid search on index: {index_name}. Error: {e}")# Re-raise the exception for further handling in the calling coderaise e
最后,我们可以组合一个 RAG 功能。我们的 RAG 流程将从查询到答案按照以下步骤进行:
- 将查询转换为 OR 子句。
- 生成 HyDE 文档并对其进行嵌入。
- 将二者作为输入传递给混合搜索。
- 检索前 n 个结果,并反转它们的顺序,使得得分最高的结果在 LLM 的上下文记忆中成为 “最新”(反向打包)。反向打包示例: 查询: “Elasticsearch query optimization techniques”
- 检索到的文档(按相关性排序):
- “Use bool queries to combine multiple search criteria efficiently.”
- “Implement caching strategies to improve query response times.”
- “Optimize index mappings for faster search performance.”
- LLM 上下文的反向顺序:
- “Optimize index mappings for faster search performance.”
- “Implement caching strategies to improve query response times.”
- “Use bool queries to combine multiple search criteria efficiently.”
- 检索到的文档(按相关性排序):
通过反转顺序,最相关的信息(1)在上下文中最后出现,从而在生成答案时可能会获得 LLM 更多的关注。
- 将上下文传递给 LLM 生成答案。
def get_context(index_name, match_query, text_query, fields, num_candidates=100, num_results=20, text_fields=["original_text", 'keyphrases', 'potential_questions', 'entities'], embedding_field="primary_embedding"):embedding=embedder.get_embeddings_from_text(text_query)results, search_body = es_query_maker.hybrid_vector_search(index_name=index_name,query_text=match_query,query_vector=embedding[0][0],text_fields=text_fields,vector_field=embedding_field,num_candidates=num_candidates,num_results=num_results)# Concatenates the text in each 'field' key of the search result objects into a single block of text.context_docs=['\n\n'.join([field+":\n\n"+j['_source'][field] for field in fields]) for j in results['hits']['hits']]# Reverse Packing to ensure that the highest ranking document is seen first by the LLM.context_docs.reverse()return context_docs, search_bodydef retrieval_augmented_generation(query_text):match_query= gpt4o.generate_query(query_text)fields=['original_text']hyde_document=gpt4o.generate_HyDE(query_text)context, search_body=get_context(index_name, match_query, hyde_document, fields)answer= gpt4o.basic_qa(query=query_text, context=context)return answer, match_query, hyde_document, context, search_body
让我们运行查询并返回答案:
According to the context, Elastic N.V. is audited by an independent registered public accounting firm, PricewaterhouseCoopers (PwC). This information is found in the section titled "report of independent registered public accounting firm," which states:"We have audited the accompanying consolidated balance sheets of Elastic N.V. [...] / s / pricewaterhouseco."
很好。没错。
实验
现在有一个重要的问题需要回答。在这些实现中投入如此多的精力和额外的复杂性,我们得到了什么?
让我们做一点比较。我们实现的 RAG 管道与基线混合搜索,没有任何我们所做的增强。我们将运行一系列小测试,看看是否注意到任何实质性的差异。我们将刚刚实现的 RAG 称为 AdvancedRAG,将基本管道称为 SimpleRAG。
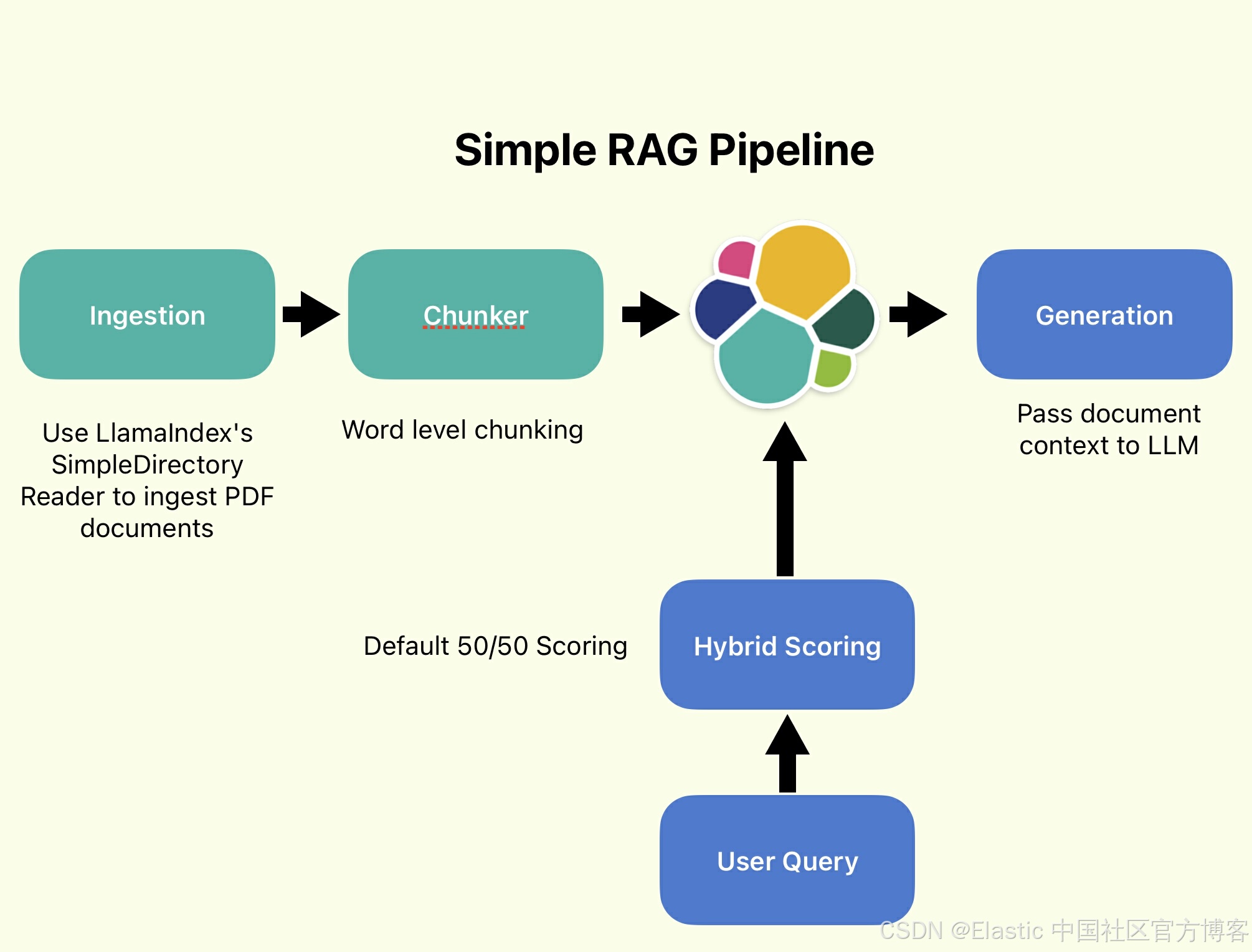
结果总结
此表总结了两个 RAG 管道的五项测试结果。我根据答案的细节和质量判断了每种方法的相对优越性,但这完全是主观判断。实际答案在此表下方重现,供你参考。话虽如此,让我们来看看他们做得怎么样!
SimpleRAG 无法回答问题 1 和 5。AdvancedRAG 还对问题 2、3 和 4 进行了更详细的说明。基于增加的细节,我更好地判断了 AdvancedRAG 答案的质量。
Test | Question | AdvancedRAG Performance | SimpleRAG Performance | AdvancedRAG Latency | SimpleRAG Latency | Winner |
---|---|---|---|---|---|---|
1 | Who audits Elastic? | Correctly identified PwC as the auditor. | Failed to identify the auditor. | 11.6s | 4.4s | AdvancedRAG |
2 | What was the total revenue in 2023? | Provided the correct revenue figure. Included additional context with revenue from previous years. | Provided the correct revenue figure. | 13.3s | 2.8s | AdvancedRAG |
3 | What product does growth primarily depend on? How much? | Correctly identified Elastic Cloud as the key driver. Included overall revenue context & greater detail. | Correctly identified Elastic Cloud as the key driver. | 14.1s | 12.8s | AdvancedRAG |
4 | Describe employee benefit plan | Gave a comprehensive description of retirement plans, health programs, and other benefits. Included specific contribution amounts for different years. | Provided a good overview of benefits, including compensation, retirement plans, work environment, and the Elastic Cares program. | 26.6s | 11.6s | AdvancedRAG |
5 | Which companies did Elastic acquire? | Correctly listed recent acquisitions mentioned in the report (CmdWatch, Build Security, Optimyze). Provided some acquisition dates and purchase prices. |
测试 1:Who audits Elastic?
AdvancedRAG
According to the context, Elastic N.V. is audited by an independent registered public accounting firm, PricewaterhouseCoopers (PwC). This information is found in the section titled "report of independent registered public accounting firm," which states: "We have audited the accompanying consolidated balance sheets of Elastic N.V. [...] / s / pricewaterhouseco."
SimpleRAG
According to the provided context, no specific auditing firm or company is mentioned as the auditor for Elastic. The context provided talks about various features, strengths, deployment options, and other details related to Elastic and its operations but does not specify which auditing firm is responsible for auditing Elastic's financial statements or internal controls.
总结:SimpleRAG 未确认 PWC 为审计师
好吧,这确实令人惊讶。这看起来像是 SimpleRAG 的搜索失败。没有检索到与审计相关的文档。让我们在下一个测试中稍微降低难度。
测试 2:total revenue 2023
AdvancedRAG
### Total Revenue for 2023According to the provided context, the total revenue for the year ended April 30, 2023, was **$1,068,989,000**. This information can be found several times in the provided text. Specifically, one part states:"The following table sets forth our results of operations for the periods presented in dollars and as a percentage of our total revenue. ... year ended April 30, 2023, ... total revenue 1,068,989".Additionally, it is mentioned here as well:"For the years ended April 30, 2023, 2022, and 2021, representing year-over-year growth of 24% for the year ended April 30, 2023... total revenue $1,068,989".Therefore, the total revenue for 2023 is $1.069 billion.
SimpleRAG
According to the context provided, the total revenue for the fiscal year ended April 30, 2023, is:$1,068,989,000 (or approximately $1.1 billion).
总结:两个 RAG 都给出了正确答案:2023 年总收入为 1,068,989,000 美元
他们俩都在这里。看起来 AdvancedRAG 可能已经获得了更广泛的文档?答案当然是更详细的,并结合了前几年的信息。考虑到我们所做的改进,这是可以预料的,但现在下结论还为时过早。
让我们增加难度。
测试 3:What product does growth primarily depend on? How much?
AdvancedRAG
### Product Growth Primarily Depends On: According to the context, "our revenue is derived primarily from subscriptions of search, observability and security built into the elastic stack." The document mentions various strategies and factors contributing to the company’s growth which include investment in "elastic cloud," "elastic stack," and the conversion of free users to paid subscribers.### Quantitative Data: - **Revenue**: The document states, "Our total revenue was $1.1 billion, $862.4 million, and $608.5 million for the years ended April 30, 2023, 2022, and 2021, respectively." - **Subscription Revenue**: "Subscriptions accounted for 92%, 93%, and 93% of our total revenue for the years ended April 30, 2023, 2022, and 2021, respectively." - **Elastic Cloud Contribution**: "For the years ended April 30, 2023, 2022, and 2021, Elastic Cloud contributed 40%, 35%, and 27% of our total revenue, respectively." - **Customer Growth**: The number of customers increased from "over 15,000 as of April 30, 2021," to "approximately 20,200 as of April 30, 2023."### Summary: Product growth for the company primarily depends on the "Elastic Stack" (which includes search, observability, and security solutions) and particularly the "Elastic Cloud." The Elastic Cloud's growing contribution to revenue highlights its significance, which grew from 27% in 2021 to 40% in 2023.
SimpleRAG
According to the provided context, growth primarily depends on **Elastic Cloud**,
which is described as the company's "family of cloud-based offerings."
The context also emphasizes the significance of Elastic Cloud in achieving
the company's long-term growth potential and its role in increasing subscription revenue.From the context:- "Increasing adoption of Elastic Cloud... is an important growth opportunity
for our business. Organizations are increasingly looking for hosted
deployment alternatives with reduced administrative burdens."
- "We believe that offering Elastic Cloud is important for achieving
our long-term growth potential, and we expect Elastic Cloud’s contribution
to our subscription revenue to continue to increase."In terms of the contribution to total revenue, here are the specific figures provided:- Elastic Cloud contributed 40% of the total revenue for the year ended April 30, 2023.
- Elastic Cloud contributed 35% of the total revenue for the year ended April 30, 2022.
- Elastic Cloud contributed 27% of the total revenue for the year ended April 30, 2021.This progression shows a clear upward trend in the importance and
contribution of Elastic Cloud to the company's overall revenue.
总结:两个 RAG 都正确地指出 Elastic Cloud 是关键的增长动力。不过,AdvancedRAG 包含更多细节,考虑到了订阅收入和客户增长,并明确提到了其他 Elastic 产品。
测试 4:Describe employee benefit plan
AdvancedRAG
### Employee Benefit Plan OverviewThe provided context outlines various elements of the employee benefit plan at Elastic, often referred to as "Elasticians." Here are the key components described in the texts:1. **401(k) Plan**:- **Coverage**: The 401(k) plan is available to substantially all U.S. employees who meet minimum age and service requirements.- **Contributions**: Elastic makes contributions to the 401(k) plan up to 6% of the participating employee’s W-2 earnings and wages.- **Expenses**: For the fiscal years ended April 30, Elastic recorded expenses of $17.9 million (2023), $15.2 million (2022), and $11.4 million (2021) related to the 401(k) plan.- **Defined-Contribution Plans in Other Countries**: Elastic has defined-contribution plans in various other countries and recorded respective expenses of $9.4 million (2023), $7.2 million (2022), and $5.1 million (2021).2. **Stock-Based Compensation**:- **Types of Awards**: Stock options, restricted stock units (RSUs), and shares under the Employee Stock Purchase Plan (ESPP).- **Fair Value Measurement**: Fair value of these stock awards is measured using models like Black-Scholes.- **Employee Stock Purchase Plan (2022 ESPP)**: - Started in 2022, it allows employees to acquire ordinary shares at a discount (85% of the market value at the beginning or end of the offering period).- Offering periods are approximately six months long.3. **Total Rewards Compensation**:- **Components**: Includes cash compensation as well as equity awards, reflecting a comprehensive interest in the well-being of the employees.- **Programs**: Market competitive medical and dental programs, mental health, and holistic well-being programs.- **Paid Time Off**: Features 16 weeks of paid leave for new parents and life-planning benefits.4. **Retirement and Income Protection Plans**:- **401(k) Match**: Elastic provides a dollar-for-dollar match up to 6% of eligible earnings up to a plan-limit maximum for U.S.-based employees.- **Similar Plans Outside the U.S.**: Competitive retirement plans available for employees based outside the United States.5. **Flexible Work Environment**: - **Distributed Workforce**: Elastic operates with a global distributed workforce model, allowing employees to work remotely and providing a broad candidate pool.6. **Learning and Development**:- **Programs**: Access to on-demand video-based learning, leadership development programs, and other specific training to develop managers and leaders.7. **Employee Engagement and Surveys**:- **Mechanisms**: Annual employee engagement survey and mid-year pulse survey.- **Objectives**: To monitor morale, develop action plans, and maintain high engagement scores.8. **Onboarding Program**:- **X-School**: An extensive new-hire orientation program to help new employees meet peers and learn about the company's products and solutions.In summary, Elastic's employee benefit plan encompasses a broad spectrum of financial, health, well-being, and professional development benefits designed to support and enhance the lives of its employees. The plan is both competitive and comprehensive, aiming to attract, retain, and engage its global workforce.
SimpleRAG
The employee benefit plan at the Company includes several key components aimed at enhancing the overall well-being and professional development of employees, referred to as "Elasticians." 1. **Compensation and Benefits:**- The Company provides market-competitive compensation packages which typically include cash compensation and equity awards.- Comprehensive medical and dental programs are offered, emphasizing mental health and holistic well-being.- Paid time off programs feature:- 16 weeks of paid leave for new parents.- Life-planning benefits.- Travel reimbursements for certain healthcare services.2. **Retirement and Income Protection Plans:**- In the United States, employees are eligible for a 401k plan with a dollar-for-dollar match by the Company up to 6% of eligible earnings, subject to a plan limit.- Similar competitive retirement and income protection plans are available for employees outside the United States.3. **Work Environment:**- The Company offers a flexible work environment, allowing most employees to work from anywhere as often as they would like.- Physical offices around the world are available for employees who prefer to work on-site or need a space for face-to-face interactions.4. **Community Involvement:**- The Elastic Cares Program supports charitable organizations that matter to employees on both local and global levels.- The program includes:- Donation matching.- Providing the Company's technology for free to certain nonprofit organizations.- Volunteer time off initiative.
总结:AdvancedRAG 进行了更深入和详细的介绍,提到了针对美国员工的 401K 计划,以及美国境外的明确供款计划。它还提到了健康和福利计划,但没有提到 SimpleRAG 提到的 Elastic Cares 计划。
测试 5:Which companies did Elastic acquire?
AdvancedRAG
Elastic acquired several companies over the years to enhance its technology and market presence. According to the provided context, the specific acquisitions include:1. **CmdWatch Security Inc.**: Acquired on September 17, 2021. The total purchase consideration was $77.8 million. 2. **Build Security Ltd.**: Acquired on September 2, 2021. The combined purchase price for Build Security Ltd. and another acquisition was $57.2 million. 3. **Optimyze Cloud Inc.**: Acquired on November 1, 2021. Part of the combined purchase price mentioned above with Build Security Ltd. 4. **Endgame, Inc.**: Although the context mentions an amended and restated stock incentive plan, it indicates that Endgame, Inc. was associated with Elastic, suggesting it could have been acquired at some point.These acquisitions are part of Elastic's strategy to enhance the technology underlying its security and observability offerings.
SimpleRAG
The provided context does not specify any companies that Elastic has acquired. Therefore, based on the context, there is no information available about the companies acquired by Elastic.
总结:SimpleRAG 未检索任何有关收购的相关信息,导致答案失败。AdvancedRAG 正确列出了 CmdWatch、Build Security 和 Optimyze,它们是报告中列出的关键收购。
结论
根据我们的测试,我们的 advanced 技术似乎增加了所呈现信息的范围和深度,从而有可能提高 RAG 答案的质量。
此外,可靠性可能会有所提高,因为 AdvancedRAG 可以正确回答措辞模糊的问题,例如 "Which companies did Elastic acquire?" 和 "Who audits Elastic",而 SimpleRAG 却不能。
但是,值得注意的是,在 5 个案例中有 3 个,基本 RAG 管道(结合了混合搜索但没有其他技术)设法产生了捕获大部分关键信息的答案。
我们应该注意,由于在数据准备和查询阶段结合了 LLMs,AdvancedRAG 的延迟通常是 SimpleRAG 的 2-5 倍。这是一笔不小的成本,可能使 AdvancedRAG 仅适用于答案质量优先于延迟的情况。
在数据准备阶段,可以使用更小、更便宜的 LLM(如 Claude Haiku 或 GPT-4o-mini)来缓解巨大的延迟成本。使用高级模型以生成答案。
这与 Wang 等人的研究结果一致。正如他们的结果所示,所做的任何改进都是相对渐进的。简而言之,简单的基线 RAG 可以让你获得不错的最终产品,同时更便宜、启动速度更快。对我来说,这是一个有趣的结论。对于速度和效率至关重要的用例,SimpleRAG 是明智的选择。对于需要挤出每一滴性能的用例,AdvancedRAG 中采用的技术可能会提供一条前进的道路。
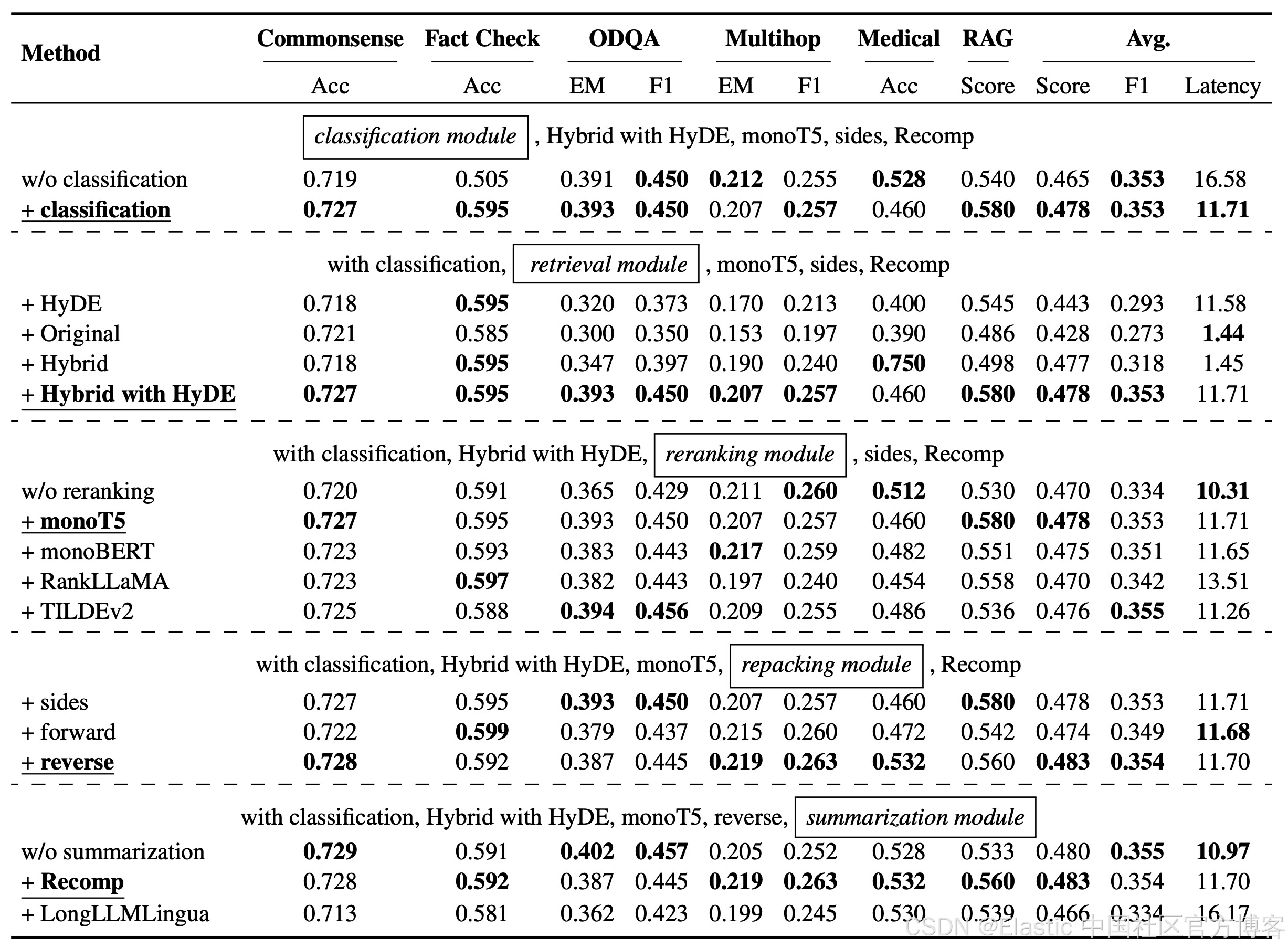
附录
提示 - prompts
RAG 问答提示
提示让 LLM 根据查询和上下文生成答案。
BASIC_RAG_PROMPT = '''
You are an AI assistant tasked with answering questions based primarily on the provided context, while also drawing on your own knowledge when appropriate. Your role is to accurately and comprehensively respond to queries, prioritizing the information given in the context but supplementing it with your own understanding when beneficial. Follow these guidelines:1. Carefully read and analyze the entire context provided.
2. Primarily focus on the information present in the context to formulate your answer.
3. If the context doesn't contain sufficient information to fully answer the query, state this clearly and then supplement with your own knowledge if possible.
4. Use your own knowledge to provide additional context, explanations, or examples that enhance the answer.
5. Clearly distinguish between information from the provided context and your own knowledge. Use phrases like "According to the context..." or "The provided information states..." for context-based information, and "Based on my knowledge..." or "Drawing from my understanding..." for your own knowledge.
6. Provide comprehensive answers that address the query specifically, balancing conciseness with thoroughness.
7. When using information from the context, cite or quote relevant parts using quotation marks.
8. Maintain objectivity and clearly identify any opinions or interpretations as such.
9. If the context contains conflicting information, acknowledge this and use your knowledge to provide clarity if possible.
10. Make reasonable inferences based on the context and your knowledge, but clearly identify these as inferences.
11. If asked about the source of information, distinguish between the provided context and your own knowledge base.
12. If the query is ambiguous, ask for clarification before attempting to answer.
13. Use your judgment to determine when additional information from your knowledge base would be helpful or necessary to provide a complete and accurate answer.Remember, your goal is to provide accurate, context-based responses, supplemented by your own knowledge when it adds value to the answer. Always prioritize the provided context, but don't hesitate to enhance it with your broader understanding when appropriate. Clearly differentiate between the two sources of information in your response.Context:
[The concatenated documents will be inserted here]Query:
[The user's question will be inserted here]Please provide your answer based on the above guidelines, the given context, and your own knowledge where appropriate, clearly distinguishing between the two:
'''
Elastic 查询生成器提示
提示使用同义词丰富查询并将其转换为 OR 格式。
ELASTIC_SEARCH_QUERY_GENERATOR_PROMPT = '''
You are an AI assistant specialized in generating Elasticsearch query strings. Your task is to create the most effective query string for the given user question. This query string will be used to search for relevant documents in an Elasticsearch index.Guidelines:
1. Analyze the user's question carefully.
2. Generate ONLY a query string suitable for Elasticsearch's match query.
3. Focus on key terms and concepts from the question.
4. Include synonyms or related terms that might be in relevant documents.
5. Use simple Elasticsearch query string syntax if helpful (e.g., OR, AND).
6. Do not use advanced Elasticsearch features or syntax.
7. Do not include any explanations, comments, or additional text.
8. Provide only the query string, nothing else.For the question "What is Clickthrough Data?", we would expect a response like:
clickthrough data OR click-through data OR click through rate OR CTR OR user clicks OR ad clicks OR search engine results OR web analyticsAND operator is not allowed. Use only OR.User Question:
[The user's question will be inserted here]Generate the Elasticsearch query string:
'''
潜在问题生成器提示
提示生成潜在问题,丰富文档元数据。
RAG_QUESTION_GENERATOR_PROMPT = '''
You are an AI assistant specialized in generating questions for Retrieval-Augmented Generation (RAG) systems. Your task is to analyze a given document and create 10 diverse questions that would effectively test a RAG system's ability to retrieve and synthesize information from this document.Guidelines:
1. Thoroughly analyze the entire document.
2. Generate exactly 10 questions that cover various aspects and levels of complexity within the document's content.
3. Create questions that specifically target:a. Key facts and informationb. Main concepts and ideasc. Relationships between different parts of the contentd. Potential applications or implications of the informatione. Comparisons or contrasts within the document
4. Ensure questions require answers of varying lengths and complexity, from simple retrieval to more complex synthesis.
5. Include questions that might require combining information from different parts of the document.
6. Frame questions to test both literal comprehension and inferential understanding.
7. Avoid yes/no questions; focus on open-ended questions that promote comprehensive answers.
8. Consider including questions that might require additional context or knowledge to fully answer, to test the RAG system's ability to combine retrieved information with broader knowledge.
9. Number the questions from 1 to 10.
10. Output only the ten questions, without any additional text, explanations, or answers.Document:
[The document content will be inserted here]Generate 10 questions optimized for testing a RAG system based on this document:
'''
HyDE 生成器提示
使用 HyDE 生成假设文档的提示
HYDE_DOCUMENT_GENERATOR_PROMPT = '''
You are an AI assistant specialized in generating hypothetical documents based on user queries. Your task is to create a detailed, factual document that would likely contain the answer to the user's question. This hypothetical document will be used to enhance the retrieval process in a Retrieval-Augmented Generation (RAG) system.Guidelines:
1. Carefully analyze the user's query to understand the topic and the type of information being sought.
2. Generate a hypothetical document that:a. Is directly relevant to the queryb. Contains factual information that would answer the queryc. Includes additional context and related informationd. Uses a formal, informative tone similar to an encyclopedia or textbook entry
3. Structure the document with clear paragraphs, covering different aspects of the topic.
4. Include specific details, examples, or data points that would be relevant to the query.
5. Aim for a document length of 200-300 words.
6. Do not use citations or references, as this is a hypothetical document.
7. Avoid using phrases like "In this document" or "This text discusses" - write as if it's a real, standalone document.
8. Do not mention or refer to the original query in the generated document.
9. Ensure the content is factual and objective, avoiding opinions or speculative information.
10. Output only the generated document, without any additional explanations or meta-text.User Question:
[The user's question will be inserted here]Generate a hypothetical document that would likely contain the answer to this query:
'''
混合搜索查询示例
{'knn': {'field': 'primary_embedding','query_vector': [0.4265527129173279,-0.1712949573993683,-0.042020395398139954,...],'k': 100,'num_candidates': 100},'query': {'bool': {'must': [{'multi_match': {'query': 'audits Elastic Elastic auditing Elastic audit process Elastic compliance Elastic security audit Elasticsearch auditing Elasticsearch compliance Elasticsearch security audit','fields': ['original_text','keyphrases','potential_questions','entities'],'type': 'best_fields','operator': 'or'}}],'should': [{'script_score': {'query': {'match_all': {}},'script': {'source': '\n double vector_score = cosineSimilarity(params.query_vector, params.vector_field) + 1.0;\n double text_score = _score;\n return 0.7 * vector_score + 0.3 * text_score;\n ','params': {'query_vector': [0.4265527129173279,-0.1712949573993683,-0.042020395398139954,...],'vector_field': 'primary_embedding'}}}}]}},'size': 10}
准备好亲自尝试了吗?开始免费试用。
Elasticsearch 集成了 LangChain、Cohere 等工具。加入我们的高级语义搜索网络研讨会,构建你的下一个 GenAI 应用程序!
原文:Advanced RAG Techniques Part 2: Querying and Testing — Search Labs
这篇关于Elasticsearch 高级 RAG 技术第 2 部分:查询和测试的文章就介绍到这儿,希望我们推荐的文章对编程师们有所帮助!