本文主要是介绍探索模块化神经网络在现代人工智能中的功效和应用,希望对大家解决编程问题提供一定的参考价值,需要的开发者们随着小编来一起学习吧!
一、介绍
在快速发展的人工智能领域,模块化神经网络 (MNN) 已成为一项关键创新。与遵循整体方法的传统神经网络架构不同,MNN 采用分散式结构。本文深入探讨了 MNN 的基础知识、它们的优势、应用以及它们带来的挑战。
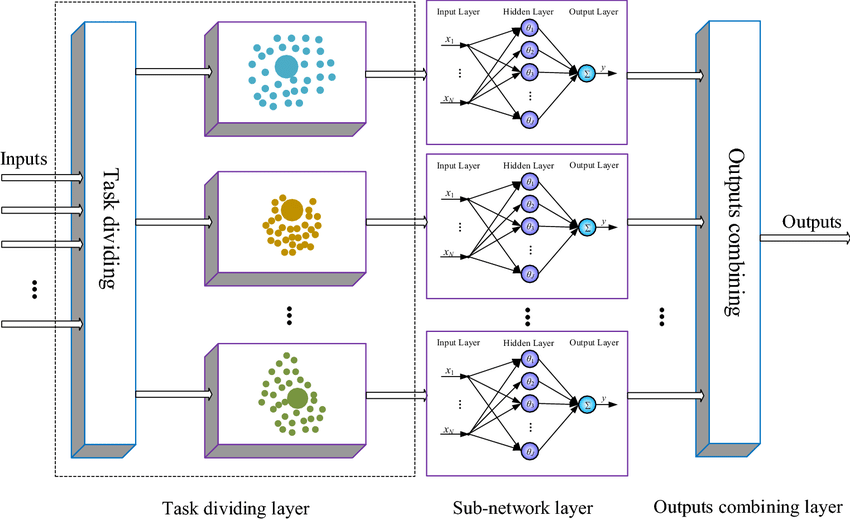
在人工智能领域,模块化神经网络证明了协作智能的力量,体现了整体大于部分之和的原则。
二、了解模块化神经网络
模块化神经网络代表了神经网络设计的范式转变。核心思想是将复杂问题分解为更小的、可管理的子任务,每个子任务由专用模块处理。这些模块本质上是单独的神经网络,经过训练专门研究整个任务的特定方面。然后整合这些模块的输出以制定全面的解决方案。
在 MNN 中,每个模块都单独训练,从而实现专业化。这种分散式训练方法与传统网络形成鲜明对比,在传统网络中,单一模型针对任务的各个方面进行训练。训练后,这些模块通过分层结构或网络进行协作,其中某些模块的输出可作为其他模块的输入。
2.1 模块化神经网络的优点
- 专业化和效率:MNN 的划分性质允许专业化,从而提高解决复杂任务的效率和有效性。每个模块都成为其特定领域的专家,使网络擅长处理多方面的问题。
- 可扩展性和灵活性:MNN 提供卓越的可扩展性和灵活性。可以添加新模块或更新现有模块,而无需重新训练整个网络。这种模块化架构使得 MNN 特别适合不断变化的任务和环境。
- 并行处理和速度:分散的结构有利于并行处理,显着加快计算速度。由于模块可以独立运行,MNN 非常适合分布式计算环境。
2.2 模块化神经网络的应用
- 机器人和自主系统:在机器人技术中,MNN 可以控制机器人的不同部分或功能。例如,单独的模块可以处理感官处理、运动协调和决策,从而形成更高效、适应性更强的机器人系统。
- 复杂问题解决:MNN 擅长解决可以分解为更小部分的复杂问题。这包括自然语言处理等领域,其中不同的模块可以处理语法、语义和上下文。
- 个性化和自适应系统:在推荐系统和个性化内容交付中,MNN 可以通过调整特定模块来适应个人用户的偏好和行为,而无需彻底检修整个系统。
2.3 挑战和未来方向
- 集成和协调: MNN 的主要挑战之一是模块的集成和协调。确保模块之间的无缝通信和协作对于网络的有效性至关重要。
- 设计和维护的复杂性:MNN 的设计和维护可能很复杂。确定模块的最佳数量、它们的具体角色和整体架构需要仔细的规划和专业知识。
- 未来展望: MNN 的未来研究可能会集中在自动化模块集成、模块间通信的高级训练算法以及探索更多样化领域的应用。
三、代码
使用 Python 创建模块化神经网络 (MNN) 的完整代码示例涉及几个步骤:生成合成数据集、为网络设计单独的模块、训练这些模块,最后集成它们。出于演示目的,我将创建一个简化的 MNN,使用合成数据集解决分类问题。我们将使用诸如numpy
数据操作以及tensorflow
构建和训练神经网络之类的库。
确保您安装了 TensorFlow 和其他必需的库。您可以使用 pip 安装它们:
pip install numpy tensorflow matplotlib sklearn
让我们开始编写 Python 代码:
import numpy as np
import tensorflow as tf
from sklearn.datasets import make_classification
from sklearn.model_selection import train_test_split
from sklearn.metrics import accuracy_score
import matplotlib.pyplot as plt# Step 2: Generate Synthetic Dataset
X, y = make_classification(n_samples=1000, n_features=20, n_informative=15, n_redundant=5, random_state=42)
X_train, X_test, y_train, y_test = train_test_split(X, y, test_size=0.2, random_state=42)# Split features for two modules
X_train_mod1 = X_train[:, :10]
X_train_mod2 = X_train[:, 10:]
X_test_mod1 = X_test[:, :10]
X_test_mod2 = X_test[:, 10:]# Step 3: Designing Modular Neural Networks
def create_module(input_shape):model = tf.keras.models.Sequential([tf.keras.layers.Dense(64, activation='relu', input_shape=input_shape),tf.keras.layers.Dense(32, activation='relu'),tf.keras.layers.Dense(16, activation='relu')])return modelmodule1 = create_module((10,))
module2 = create_module((10,))# Step 4: Training the Modules
module1.compile(optimizer='adam', loss='sparse_categorical_crossentropy', metrics=['accuracy'])
module2.compile(optimizer='adam', loss='sparse_categorical_crossentropy', metrics=['accuracy'])module1.fit(X_train_mod1, y_train, epochs=10, batch_size=32, verbose=0)
module2.fit(X_train_mod2, y_train, epochs=10, batch_size=32, verbose=0)# Step 5: Integration and Final Classification
combined_input = tf.keras.layers.concatenate([module1.output, module2.output])
final_output = tf.keras.layers.Dense(2, activation='softmax')(combined_input)
final_model = tf.keras.models.Model(inputs=[module1.input, module2.input], outputs=final_output)final_model.compile(optimizer='adam', loss='sparse_categorical_crossentropy', metrics=['accuracy'])
final_model.fit([X_train_mod1, X_train_mod2], y_train, epochs=10, batch_size=32, verbose=0)# Evaluation
y_pred = np.argmax(final_model.predict([X_test_mod1, X_test_mod2]), axis=1)
accuracy = accuracy_score(y_test, y_pred)
print(f'Accuracy: {accuracy}')# Step 6: Plotting the Results
# Here you can add any specific plots you want, like loss curves or accuracy over epochs.
import matplotlib.pyplot as plt# Modifying the training process to store history
history1 = module1.fit(X_train_mod1, y_train, epochs=10, batch_size=32, verbose=0, validation_split=0.2)
history2 = module2.fit(X_train_mod2, y_train, epochs=10, batch_size=32, verbose=0, validation_split=0.2)
final_history = final_model.fit([X_train_mod1, X_train_mod2], y_train, epochs=10, batch_size=32, verbose=0, validation_split=0.2)# Plotting
plt.figure(figsize=(12, 6))# Plot training & validation accuracy values for Module 1
plt.subplot(2, 3, 1)
plt.plot(history1.history['accuracy'])
plt.plot(history1.history['val_accuracy'])
plt.title('Module 1 Accuracy')
plt.ylabel('Accuracy')
plt.xlabel('Epoch')
plt.legend(['Train', 'Test'], loc='upper left')# Plot training & validation loss values for Module 1
plt.subplot(2, 3, 2)
plt.plot(history1.history['loss'])
plt.plot(history1.history['val_loss'])
plt.title('Module 1 Loss')
plt.ylabel('Loss')
plt.xlabel('Epoch')
plt.legend(['Train', 'Test'], loc='upper left')# Plot training & validation accuracy values for Module 2
plt.subplot(2, 3, 3)
plt.plot(history2.history['accuracy'])
plt.plot(history2.history['val_accuracy'])
plt.title('Module 2 Accuracy')
plt.ylabel('Accuracy')
plt.xlabel('Epoch')
plt.legend(['Train', 'Test'], loc='upper left')# Plot training & validation loss values for Module 2
plt.subplot(2, 3, 4)
plt.plot(history2.history['loss'])
plt.plot(history2.history['val_loss'])
plt.title('Module 2 Loss')
plt.ylabel('Loss')
plt.xlabel('Epoch')
plt.legend(['Train', 'Test'], loc='upper left')# Plot training & validation accuracy values for Final Model
plt.subplot(2, 3, 5)
plt.plot(final_history.history['accuracy'])
plt.plot(final_history.history['val_accuracy'])
plt.title('Final Model Accuracy')
plt.ylabel('Accuracy')
plt.xlabel('Epoch')
plt.legend(['Train', 'Test'], loc='upper left')# Plot training & validation loss values for Final Model
plt.subplot(2, 3, 6)
plt.plot(final_history.history['loss'])
plt.plot(final_history.history['val_loss'])
plt.title('Final Model Loss')
plt.ylabel('Loss')
plt.xlabel('Epoch')
plt.legend(['Train', 'Test'], loc='upper left')plt.tight_layout()
plt.show()
该脚本演示了模块化神经网络的基本实现。根据您的具体问题,架构、模块数量及其集成方式可能会有很大差异。另外,请记住根据任务的复杂性调整纪元、批量大小和网络层。
四、结论
模块化神经网络标志着人工智能领域的重大进步,提供了灵活、高效且可扩展的问题解决方法。它们处理复杂、多方面任务的能力使它们成为各种应用中的宝贵工具。虽然它们带来了一定的挑战,但正在进行的研究和开发有望进一步增强它们的能力,巩固它们在人工智能未来的作用。
这篇关于探索模块化神经网络在现代人工智能中的功效和应用的文章就介绍到这儿,希望我们推荐的文章对编程师们有所帮助!