本文主要是介绍ai人工智能测面相 准吗_AI会对电动蠕变尖叫吗?,希望对大家解决编程问题提供一定的参考价值,需要的开发者们随着小编来一起学习吧!
ai人工智能测面相 准吗
A look into artificial intelligence and the machine learning models that encompass the foundation of much of our user generated content
深入研究人工智能和机器学习模型,这些模型涵盖了我们许多用户生成内容的基础
When most people think of machine learning in relation to themselves, something like the auto-correct peppered throughout their texts might come to mind. But these technologies are integrated into so many industries that touch us daily. In my previous article linked below, I talk about the broad strokes of machine learning by looking into the technologies of self driving cars, healthcare, and briefly touched on the YouTube algorithm. In this article, I’ll be diving farther into that last concept by approaching three different violations of terms and services on a social media platform and the role that machine learning has in mitigating any hardships caused by these violations.
当大多数人想到与自己相关的机器学习时,可能会想到诸如整篇文章中的自动更正之类的东西。 但是,这些技术已集成到每天接触我们的众多行业中。 在下面的前一篇文章链接中,我通过研究自动驾驶汽车,医疗保健的技术来谈论机器学习的广泛动因,并简要介绍了YouTube算法。 在本文中,我将通过在社交媒体平台上处理三种不同的违反条款和服务的行为,以及机器学习在减轻这些违反行为造成的任何困难中所扮演的角色,进一步深入探讨最后一个概念。
To fully understand the decision making behavior, we must go over the basics of these algorithms. Machine learning is constructed on the foundation of three different styles of leaning: Supervised, Unsupervised and Reinforcement Learning. Supervised learning is based on working the algorithm according to a given set of features. This relies on human generated guidance, but also requires found data to learn. Secondly, unsupervised learning is based in found data, but it relies on machine created guidance. This learning style has no given features and the algorithm has to assign features to unlabeled data based on its previous decisions. Lastly we have reinforcement learning, which relies entirely on its given feedback. By rewarding the algorithm through a marriage of human and machine created guidance, systems can refine their algorithms for maximum efficiency. This is all done in a simple flow of input, then utilization of a model to illustrate the way the material is analyzed, and then to output. If the material is incorrect, the machine goes back into the model to correct itself, then again to output. This is done until the correct decision is made. Below I’ve included an amazing video by Simplilearn that visualizes these concepts wonderfully.
要完全了解决策行为,我们必须仔细研究这些算法的基础知识。 机器学习是基于三种不同的学习方式构建的:监督学习,无监督学习和强化学习。 监督学习是基于根据给定的一组功能来运行算法的。 这依赖于人工生成的指导,但是也需要找到的数据来学习。 其次,无监督学习基于发现的数据,但是它依赖于机器创建的指导。 这种学习方式没有给定的特征,算法必须根据其先前的决策将特征分配给未标记的数据。 最后,我们进行强化学习,这完全依赖于其给定的反馈。 通过结合人工指导和机器指导来对算法进行奖励,系统可以优化其算法以实现最大效率。 所有这些都通过简单的输入流程完成,然后利用模型来说明材料的分析方式,然后输出。 如果材料不正确,则机器将返回模型进行自我校正,然后再次输出。 这样做直到做出正确的决定。 下面,我包括Simplilearn的精彩视频,将这些概念完美地形象化了。
In this first violation, we look at what happens when someone uploads copyrighted content. In the current evolution of large social media domains founded on user generated content, there are many safeguards that allow from duplication of content from large media conglomerates. Take the Marvel movies for example, after each production of the box office hit, a blueprint is made of the movie to match against content being uploaded. This blueprint will flag any material that is deemed a fraudulent upload and the file will most likely not complete the upload. This is an example of supervised learning, since the algorithm knows what it is looking out for. In some cases such as lesser known media, the file could complete the upload. It could even stay up for a few hours, but eventually it will most likely be taken down either by way of machine learning algorithms or by a user flagging the content. Personally I can attest to a recent pandemic related matter where my acquaintance wanted to create a viewing party for friends. Even with choosing a certain obscure folk horror film from 1973 running only eighty-seven minutes, the viewing party lasted a brief forty-five before the algorithm flagged the content and removed it.
在第一次违规中,我们查看了当有人上传受版权保护的内容时会发生什么。 在基于用户生成的内容的大型社交媒体领域的当前发展中,有许多安全措施可防止大型媒体集团复制内容。 以Marvel电影为例,在每次制作票房成功后,都会根据电影上载的内容制作蓝图。 该蓝图将标记任何被认为是欺诈性上传的材料,并且该文件很可能不会完成上传。 这是监督学习的示例,因为该算法知道要寻找的内容。 在某些情况下(例如鲜为人知的媒体),文件可以完成上传。 它甚至可能会停留几个小时,但最终很可能会通过机器学习算法或用户标记内容将其删除。 就我个人而言,我可以证明最近发生的一次与大流行有关的事情,我的熟人想为朋友们组织一个观看聚会。 即使选择了一部从1973年开始拍摄的不起眼的民间恐怖片,它只运行了87分钟,在算法标记内容并将其删除之前,观看者仍持续了短暂的45分钟。
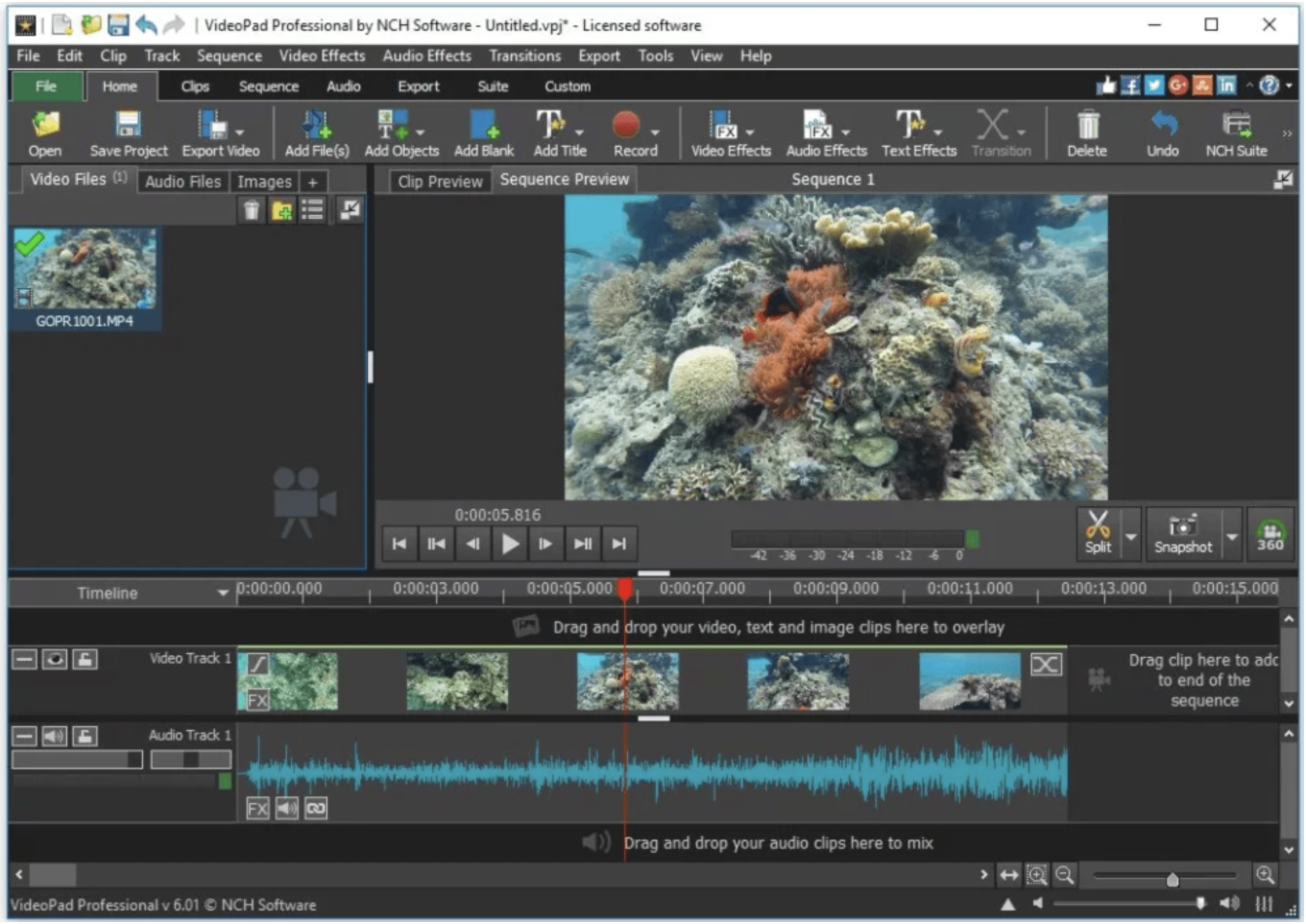
Now since these blueprints have proven themselves to work, it’s time to take a look at what goes into them. Firstly we can look at the importance of a file’s audio. When looking at content in an editing software, such as the example above, one can see the mapping of sound waves alongside its corresponding visual frames. This shows peaks and valleys, all related to its corresponding action and its visual representation of that. To simply put it, each sound has a way it is expressed in sound waves. Whether it’s the sound of someone’s coffee mug setting down on a hardwood table, or the sound of artillery firing across a field, these noises have a visual representation to coincide. This is why some bootleg copies of films can be seen with slightly distorted audio, or the visual frames cropped or vignetted with a graphic to try to trick the algorithms into overlooking this copyright violation. In the below article from TechCrunch, that exact thing is discussed. These failsafes are only viable on whole uploads in their original standing, so in the case of someone willing to edit the video, or share it in pieces, the algorithm might not be able to effectively remove the content.
现在,由于这些蓝图已经证明它们可以工作,因此该看看其中的内容了。 首先,我们可以看一下文件音频的重要性。 在编辑软件(如上述示例)中查看内容时,可以看到声波在其对应的可视帧旁边的映射。 这显示了峰和谷,所有峰和谷都与其相应的动作及其视觉表示有关。 简而言之,每种声音都有一种在声波中表达的方式。 不管是有人将咖啡杯放在硬木桌上的声音,还是大炮在田野上开火的声音,这些声音都具有视觉上的重合。 这就是为什么可以看到一些电影的盗版复制品,其音频略有失真,或者视觉画面被图形裁剪或渐晕,以试图诱使算法忽略这种侵犯版权的行为。 在TechCrunch的以下文章中,讨论了确切的内容。 这些故障保护功能仅能以原始状态在整个上传过程中使用,因此,如果有人愿意编辑视频或分段共享视频,则该算法可能无法有效删除内容。
Now in the scenario of re-uploading content from a media conglomerate, repercussions can be varied. Mostly people will receive a notice, maybe get a tick on their account, but in the case of a repeat offender, one could face legal ramifications. One thing is worth noting though, and that is the pervasive issue of the theft of individuals media, whether it be malicious or “for the views.” In this scenario, an individual chooses to re-upload content to their social media but they don’t tag the original poster. Now some might try to go through the report through the app function, others may reach out through email or social media, but the running theme in this scenario is that the outcome is usually the same: nothing. With the current way some platforms are structured, their machine learning algorithms and human intervention are coming under fire. Some criticize that these algorithms aren’t for the people, but actually to uplift corporations and sponsored posts. But what would this even look like in the machine learning mindset? Well this is where the “learning” part comes in. In the previous example, the violation was found by the blueprint given to the system. In this, maybe a violation of sorts was detected, but maybe the content flagged is gaining a lot of traction to the site. One could argue that by way of human intervention, the algorithms can begin to favor the corporate funded media significantly over the user with 1,000 subscribers that you’re actually subscribed to yourself. Although shown here in a somewhat negative view, this is an example of reinforcement learning. By human intervention guiding the way the machine learns, those previously validated or corrected choices now determine the way the algorithm reacts to similar content uploaded onto the platform.
现在,在从媒体集团重新上传内容的场景中,影响可能会有所不同。 通常情况下,人们会收到通知,也许会在帐户上打勾,但如果屡犯,则可能面临法律后果。 值得一提的是,这是个人媒体失窃的普遍问题,无论是恶意的还是“出于观点”。 在这种情况下,个人选择将内容重新上传到他们的社交媒体,但是他们没有标记原始海报。 现在,有些人可能会尝试通过应用程序功能查看报告,其他人可能会通过电子邮件或社交媒体进行查询,但是这种情况下的运行主题是结果通常是相同的:没有。 以当前的方式构建某些平台,其机器学习算法和人工干预正受到抨击。 有人批评说这些算法不是针对人民的,而是实际上是为了提升公司和赞助职位。 但是,这在机器学习心态中会是什么样呢? 好的,这就是“学习”部分的所在。在前面的示例中,违规是通过给系统的蓝图找到的。 在这种情况下,可能检测到某种形式的违规行为,但是可能所标记的内容正对该站点产生很大的吸引力。 有人可能会争辩说,通过人为干预,这些算法可能开始比起实际上由您自己订阅的拥有1,000个订阅者的用户来更偏爱由企业资助的媒体。 尽管此处显示的是负面图,但这是强化学习的示例。 通过人工干预指导机器学习的方式,那些先前经过验证或更正的选择现在可以确定算法对上传到平台上的相似内容的React方式。
Now with this mindset of a heavily favored algorithm, the problem can become even darker when the issue of the theft and distribution of malicious media comes into play. In this scenario, something is uploaded to bully or harass a user. By its very nature, it most likely falls against the community terms of service. Although people often don’t get the media taken down, some do, and if the issue is widespread enough, a blueprint could be made of that particular media to screen against re-uploads. But the issue in this scenario is usually how long something takes to be flagged, even when a blueprint is created. In some of the worst cases media can be up for days, even years, garnering thousands of views and downloads alike, all while at the disappointment and anxiety of the person who went through all the trouble to try and avoid these re-uploads. This can result in a myriad of negative situations, pushing people to their wits end. As seen below with a simple Google search of “remove negative online content” the results and resources are staggering. From a momentary glance, one can see just how dangerous some things can get for individuals being targeted in such a heinous way.
现在,有了这种广受青睐的算法的思维定势,当盗窃和分发恶意媒体的问题开始发挥作用时,问题可能会变得更加严峻。 在这种情况下,某些东西会被上传到欺凌者或骚扰用户。 就其本质而言,它很可能违反社区服务条款。 尽管人们常常不让媒体失望,但有些人会这么做,如果问题足够普遍,则可以针对特定媒体制作蓝图,以防止重新上传。 但是这种情况下的问题通常是,即使创建了蓝图,也需要花费多长时间来标记某些内容。 在某些最坏的情况下,媒体可能需要几天甚至几年的时间,才能获得成千上万的观看次数和下载量,而与此同时,经历了所有麻烦的人都感到失望和焦虑,他们试图避免这些重新上传。 这可能导致无数负面情况,使人们无所适从。 如下所示,通过简单的Google搜索来“删除负面的在线内容”,结果和资源令人震惊。 从片刻的眼神中,可以看出以这种令人发指的方式,某些事情对于成为被攻击目标的人来说是多么危险。
Lastly in a much more lighthearted scenario, we look at plain old user error. Maybe someone accidentally posts a scandalous photo or they don’t realize something is in the background, the algorithm can use previous decisions to assign a label to something and deem it against terms of service. In this example we take a look at the odd but existing problem of people somehow posting their personal information online. In one example, a credit card is in plain sight, in another a medical ID bracelet. All of these are ways someone could seriously harm someone or themselves, but posted completely accidentally, sandwiched in between a string of normal posts. The algorithm could see these key factors such as the syntax of 16 digits organized in chunks of four and recognize it as someones personal information and remove the post. In another scenario, the scandalous photo accidentally posted goes through that same checking system. By checking against basic mathematics of the photograph and previously made choices, the algorithm categorizes the contents and determines whether or not its deemed appropriate. In these scenarios, unsupervised learning is most likely what would be used. This is because the content is being checked without a blueprint of what to expect. The algorithm is what is responsible for finding a category for everything based on its own machine based learning.
最后,在更为轻松的情况下,我们来看普通的旧用户错误。 也许有人不小心发布了一张丑陋的照片,或者他们没有意识到背景中有东西,该算法可以使用先前的决定为某物分配标签,并根据服务条款认为该标签。 在此示例中,我们看一下人们以某种方式在线发布其个人信息的奇怪但存在的问题。 在一个示例中,信用卡清晰可见,在另一个中则是医疗ID手镯。 所有这些都是某人可能严重伤害某人或自己的方式,但完全意外地将其张贴在一系列正常职位之间。 该算法可以看到这些关键因素,例如以四个为一组的16位数字的语法,并将其识别为某人的个人信息,并删除该帖子。 在另一种情况下,意外张贴的丑闻照片将通过同一检查系统。 通过对照照片的基本数学和先前做出的选择,该算法对内容进行分类并确定其是否合适。 在这些情况下,最有可能使用无监督学习。 这是因为在检查内容时没有预期内容的蓝图。 该算法负责根据自己的基于机器的学习为所有事物找到类别。
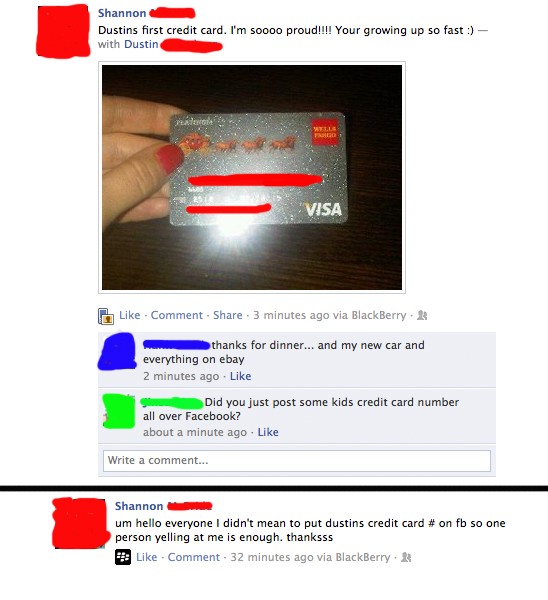
No matter what the scenario, a simple flow of information is happening. From input, the material goes into the given model, then to output. This deceptively simple flow if information only begins to encompass the possibilities machine learning offers. In such a fast growing world, it is hard to even predict what our technologies of the future will even look like. One thing can be certain though, and that is the rise of the use of machine learning in almost all industries. These new breakthroughs in efficiency are based on the choices happening in our algorithms today. If humans hone these capabilities sooner and create more successful, unbiased algorithms, the future of social media and technology as a whole could be changed for the better for decades to come.
无论发生什么情况,都将发生简单的信息流。 从输入开始,材料进入给定模型,然后输出。 如果信息仅开始包含机器学习提供的可能性,那么这种看似简单的流程。 在如此快速发展的世界中,甚至无法预测我们未来的技术将是什么样。 但可以肯定的是,几乎所有行业都在使用机器学习。 这些新的效率突破是基于当今算法中发生的选择。 如果人类能够早日磨练这些功能并创建更成功,更公正的算法,那么整个社会媒体和技术的未来可能会在未来几十年变得更好。
— — — Written by Kathleen McKiernan for Holberton New Haven — — —
— —由凯瑟琳·麦克基南(Kathleen McKiernan)为霍尔伯顿·纽黑文(Herberton New Haven)撰写— — —
翻译自: https://medium.com/swlh/does-ai-scream-at-electric-creeps-a420fc0e7f05
ai人工智能测面相 准吗
相关文章:
这篇关于ai人工智能测面相 准吗_AI会对电动蠕变尖叫吗?的文章就介绍到这儿,希望我们推荐的文章对编程师们有所帮助!