本文主要是介绍deepseek-coder模型量化,希望对大家解决编程问题提供一定的参考价值,需要的开发者们随着小编来一起学习吧!
1 简介
DeepSeek-Coder在多种编程语言和各种基准测试中取得了开源代码模型中最先进的性能。
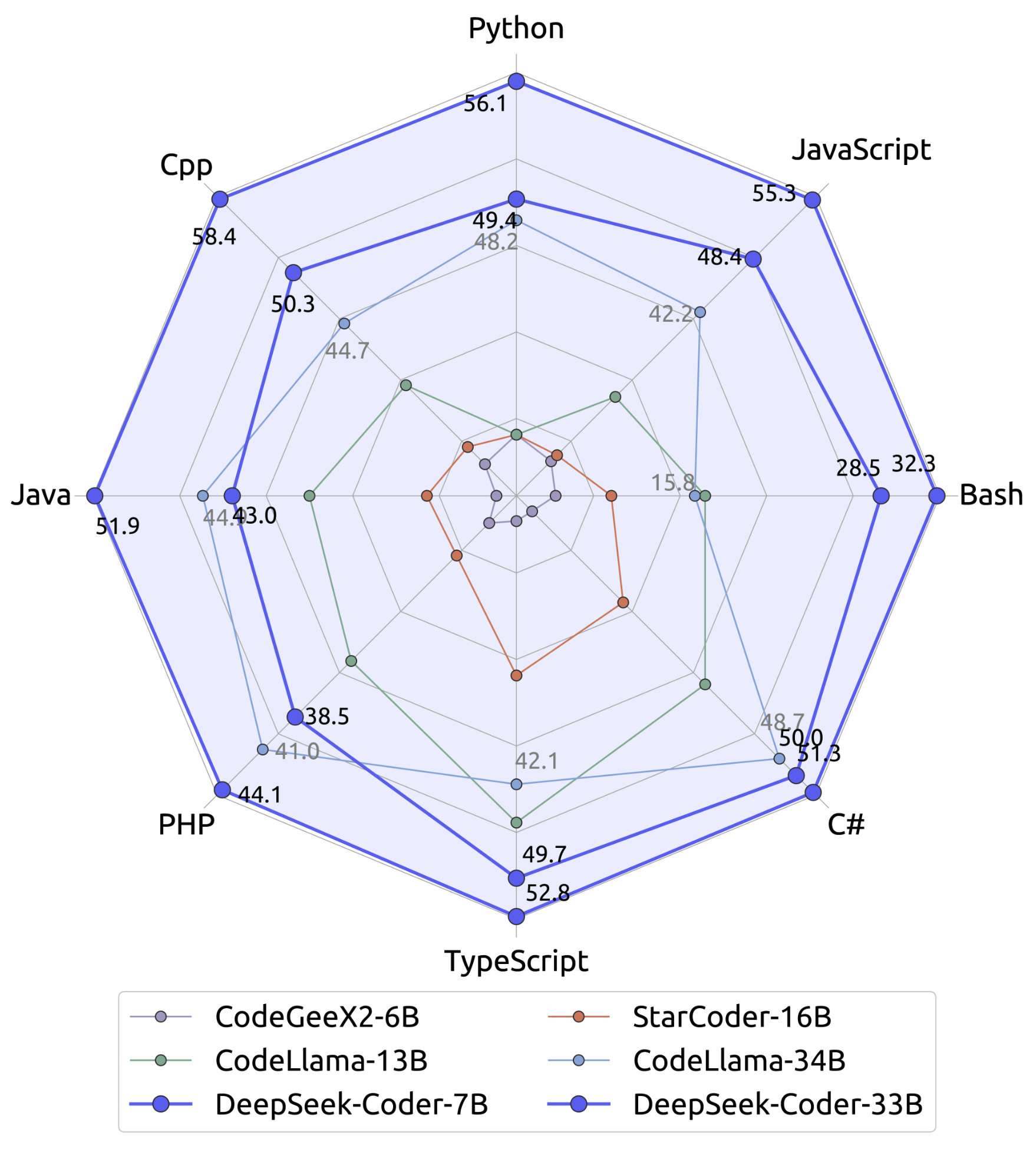
为尝试在开发板进行部署,首先利用llama.cpp对其进行量化。
2 llama.cpp安装
git clone
之后进入文件夹make
即可,再将依赖补全pip install -r requirements.txt
3 量化
按照GitHub上DeepSeek和llama.cpp官方的信息,后者对deepseek模型的量化目前的支持(进度)还不是很完善。
下面记录一下目前量化出现的问题。
3.1 DeepSeek官方tutorial
依照官方md
git clone https://github.com/DOGEwbx/llama.cpp.git
cd llama.cpp
git checkout regex_gpt2_preprocess
出现error: pathspec 'regex_gpt2_preprocess' did not match any file(s) known to git
# set up the environment according to README
make
python3 -m pip install -r requirements.txt
# generate GGUF model
python convert-hf-to-gguf.py <MODEL_PATH> --outfile <GGUF_PATH> --model-name deepseekcoder
出现convert-hf-to-gguf.py: error: unrecognized arguments: --model-name deepseekcoder
去掉--model-name
参数,出现NotImplementedError: Architecture 'LlamaForCausalLM' not supported!
,解释。
3.2 convert.py转换
参考这个comment和这个comment,使用convert.py
进行转换。
看起来这个修改已经被合并了,浅浅试一下。
python convert.py <MODEL_PATH> --outfile <GGUF_PATH>
出现错误: Exception: Vocab size mismatch (model has 32256, but ../DeepSeek-Coder/models/deepseek-coder-1.3b-instruct has 32022). Add the --pad-vocab option and try again.
详细的log如下
Loading model file ../DeepSeek-Coder/models/deepseek-coder-1.3b-instruct/model.safetensors
params = Params(n_vocab=32256, n_embd=2048, n_layer=24, n_ctx=16384, n_ff=5504, n_head=16, n_head_kv=16, n_experts=None, n_experts_used=None, f_norm_eps=1e-06, rope_scaling_type=<RopeScalingType.LINEAR: 'linear'>, f_rope_freq_base=100000, f_rope_scale=4.0, n_orig_ctx=None, rope_finetuned=None, ftype=None, path_model=PosixPath('../DeepSeek-Coder/models/deepseek-coder-1.3b-instruct'))
Found vocab files: {'spm': None, 'bpe': None, 'hfft': PosixPath('../DeepSeek-Coder/models/deepseek-coder-1.3b-instruct/tokenizer.json')}
Loading vocab file PosixPath('../DeepSeek-Coder/models/deepseek-coder-1.3b-instruct/tokenizer.json'), type 'hfft'
fname_tokenizer: ../DeepSeek-Coder/models/deepseek-coder-1.3b-instruct
Special tokens have been added in the vocabulary, make sure the associated word embeddings are fine-tuned or trained.
Vocab info: <HfVocab with 32000 base tokens and 22 added tokens>
Special vocab info: <SpecialVocab with 0 merges, special tokens {'bos': 32013, 'eos': 32021, 'pad': 32014}, add special tokens {'bos': True, 'eos': False}>
Permuting layer 0
Permuting layer 1
Permuting layer 2
...省略部分
Permuting layer 22
Permuting layer 23
lm_head.weight -> output.weight | BF16 | [32256, 2048]
model.embed_tokens.weight -> token_embd.weight | BF16 | [32256, 2048]
model.layers.0.input_layernorm.weight -> blk.0.attn_norm.weight | BF16 | [2048]
model.layers.0.mlp.down_proj.weight -> blk.0.ffn_down.weight | BF16 | [2048, 5504]
model.layers.0.mlp.gate_proj.weight -> blk.0.ffn_gate.weight | BF16 | [5504, 2048]
...
model.layers.18.self_attn.v_proj.weight -> blk.18.attn_v.weight | BF16 | [2048, 2048]
model.layers.19.input_layernorm.weight -> blk.19.attn_norm.weight | BF16 | [2048]
...
model.layers.9.input_layernorm.weight -> blk.9.attn_norm.weight | BF16 | [2048]
model.layers.9.mlp.down_proj.weight -> blk.9.ffn_down.weight | BF16 | [2048, 5504]
model.layers.9.mlp.gate_proj.weight -> blk.9.ffn_gate.weight | BF16 | [5504, 2048]
model.layers.9.mlp.up_proj.weight -> blk.9.ffn_up.weight | BF16 | [5504, 2048]
model.layers.9.post_attention_layernorm.weight -> blk.9.ffn_norm.weight | BF16 | [2048]
model.layers.9.self_attn.k_proj.weight -> blk.9.attn_k.weight | BF16 | [2048, 2048]
model.layers.9.self_attn.o_proj.weight -> blk.9.attn_output.weight | BF16 | [2048, 2048]
model.layers.9.self_attn.q_proj.weight -> blk.9.attn_q.weight | BF16 | [2048, 2048]
model.layers.9.self_attn.v_proj.weight -> blk.9.attn_v.weight | BF16 | [2048, 2048]
model.norm.weight -> output_norm.weight | BF16 | [2048]
Writing ../DeepSeek-Coder/models/1.3b.gguf, format 1
Traceback (most recent call last):File "/home/stlinpeiyang/lpy22/LLM/llama.cpp/convert.py", line 1479, in <module>main()File "/home/stlinpeiyang/lpy22/LLM/llama.cpp/convert.py", line 1473, in mainOutputFile.write_all(outfile, ftype, params, model, vocab, special_vocab,File "/home/stlinpeiyang/lpy22/LLM/llama.cpp/convert.py", line 1117, in write_allcheck_vocab_size(params, vocab, pad_vocab=pad_vocab)File "/home/stlinpeiyang/lpy22/LLM/llama.cpp/convert.py", line 963, in check_vocab_sizeraise Exception(msg)
Exception: Vocab size mismatch (model has 32256, but ../DeepSeek-Coder/models/deepseek-coder-1.3b-instruct has 32022). Add the --pad-vocab option and try again.
3.2.1 添加--pad-vocab
首先,显然提示添加参数,根据提示加上--pad-vocab
参数后,成功运行并可以成功量化,但是在测试时,会出现以下错误
terminate called after throwing an instance of 'std::out_of_range'what(): _Map_base::at
Aborted (core dumped)
这种情况有相关的issue comment&这个。
从llama.cpp
的pull request和issue来看,应该是还没处理好。菜鸡只能嗷嗷待哺了
😥。不知道TheBloke大佬是怎么处理的👍。
(表情网站)
3.2.2 修改vocab_size
其次,根据错误的前半段的model has 32256, but ... has 32022
,有类似的issue.
根据comment,对vocal_size
进行修改。相应地,打开deepseek-coder-1.3b-instruct中的config.json
文件,试将"vocab_size": 32256
修改为"vocal_size": 32022
。再次运行
python convert.py <MODEL_PATH> --outfile <GGUF_PATH>
输出的log如下
Loading model file ../DeepSeek-Coder/models/deepseek-coder-1.3b-instruct/model.safetensors
params = Params(n_vocab=32022, n_embd=2048, n_layer=24, n_ctx=16384, n_ff=5504, n_head=16, n_head_kv=16, n_experts=None, n_experts_used=None, f_norm_eps=1e-06, rope_scaling_type=<RopeScalingType.LINEAR: 'linear'>, f_rope_freq_base=100000, f_rope_scale=4.0, n_orig_ctx=None, rope_finetuned=None, ftype=None, path_model=PosixPath('../DeepSeek-Coder/models/deepseek-coder-1.3b-instruct'))
Found vocab files: {'spm': None, 'bpe': None, 'hfft': PosixPath('../DeepSeek-Coder/models/deepseek-coder-1.3b-instruct/tokenizer.json')}
Loading vocab file PosixPath('../DeepSeek-Coder/models/deepseek-coder-1.3b-instruct/tokenizer.json'), type 'hfft'
fname_tokenizer: ../DeepSeek-Coder/models/deepseek-coder-1.3b-instruct
Special tokens have been added in the vocabulary, make sure the associated word embeddings are fine-tuned or trained.
Vocab info: <HfVocab with 32000 base tokens and 22 added tokens>
Special vocab info: <SpecialVocab with 0 merges, special tokens {'bos': 32013, 'eos': 32021, 'pad': 32014}, add special tokens {'bos': True, 'eos': False}>
Permuting layer 0
Permuting layer 1
Permuting layer 2
...省略部分
lm_head.weight -> output.weight | BF16 | [32256, 2048]
model.embed_tokens.weight -> token_embd.weight | BF16 | [32256, 2048]
model.layers.0.input_layernorm.weight -> blk.0.attn_norm.weight | BF16 | [2048]
model.layers.0.mlp.down_proj.weight -> blk.0.ffn_down.weight | BF16 | [2048, 5504]
model.layers.0.mlp.gate_proj.weight -> blk.0.ffn_gate.weight | BF16 | [5504, 2048]
model.layers.0.mlp.up_proj.weight -> blk.0.ffn_up.weight | BF16 | [5504, 2048]
model.layers.0.post_attention_layernorm.weight -> blk.0.ffn_norm.weight | BF16 | [2048]
model.layers.0.self_attn.k_proj.weight -> blk.0.attn_k.weight | BF16 | [2048, 2048]
model.layers.0.self_attn.o_proj.weight -> blk.0.attn_output.weight | BF16 | [2048, 2048]
model.layers.0.self_attn.q_proj.weight -> blk.0.attn_q.weight | BF16 | [2048, 2048]
model.layers.0.self_attn.v_proj.weight -> blk.0.attn_v.weight
...省略部分
model.layers.9.self_attn.q_proj.weight -> blk.9.attn_q.weight | BF16 | [2048, 2048]
model.layers.9.self_attn.v_proj.weight -> blk.9.attn_v.weight | BF16 | [2048, 2048]
model.norm.weight -> output_norm.weight | BF16 | [2048]
Writing ../DeepSeek-Coder/models/1.3b.gguf, format 1
Ignoring added_tokens.json since model matches vocab size without it.
gguf: This GGUF file is for Little Endian only
gguf: Setting special token type bos to 32013
gguf: Setting special token type eos to 32021
gguf: Setting special token type pad to 32014
gguf: Setting add_bos_token to True
gguf: Setting add_eos_token to False
gguf: Setting chat_template to {% if not add_generation_prompt is defined %}
{% set add_generation_prompt = false %}
{% endif %}
{%- set ns = namespace(found=false) -%}
{%- for message in messages -%}{%- if message['role'] == 'system' -%}{%- set ns.found = true -%}{%- endif -%}
{%- endfor -%}
{{bos_token}}{%- if not ns.found -%}
{{'You are an AI programming assistant, utilizing the Deepseek Coder model, developed by Deepseek Company, and you only answer questions related to computer science. For politically sensitive questions, security and privacy issues, and other non-computer science questions, you will refuse to answer\n'}}
{%- endif %}
{%- for message in messages %}{%- if message['role'] == 'system' %}
{{ message['content'] }}{%- else %}{%- if message['role'] == 'user' %}
{{'### Instruction:\n' + message['content'] + '\n'}}{%- else %}
{{'### Response:\n' + message['content'] + '\n<|EOT|>\n'}}{%- endif %}{%- endif %}
{%- endfor %}
{% if add_generation_prompt %}
{{'### Response:'}}
{% endif %}
[ 1/219] Writing tensor output.weight | size 32256 x 2048 | type F16 | T+ 0
[ 2/219] Writing tensor token_embd.weight | size 32256 x 2048 | type F16 | T+ 0
...省略部分
[216/219] Writing tensor blk.9.attn_output.weight | size 2048 x 2048 | type F16 | T+ 2
[217/219] Writing tensor blk.9.attn_q.weight | size 2048 x 2048 | type F16 | T+ 2
[218/219] Writing tensor blk.9.attn_v.weight | size 2048 x 2048 | type F16 | T+ 2
[219/219] Writing tensor output_norm.weight | size 2048 | type F32 | T+ 2
Wrote ../DeepSeek-Coder/models/1.3b.gguf
成功生成gguf
文件。下一步进行量化
./quantize ${out_model.gguf} ${out_model-q5_0.gguf} q5_0
输出log如下
main: build = 1 (231ae28)
main: built with cc (Ubuntu 9.4.0-1ubuntu1~20.04.2) 9.4.0 for x86_64-linux-gnu
main: quantizing '../DeepSeek-Coder/models/1.3b.gguf' to '../DeepSeek-Coder/models/1.3b-q5_0.gguf' as Q5_0
llama_model_loader: loaded meta data with 24 key-value pairs and 219 tensors from ../DeepSeek-Coder/models/1.3b.gguf (version GGUF V3 (latest))
llama_model_loader: Dumping metadata keys/values. Note: KV overrides do not apply in this output.
llama_model_loader: - kv 0: general.architecture str = llama
llama_model_loader: - kv 1: general.name str = models
llama_model_loader: - kv 2: llama.context_length u32 = 16384
llama_model_loader: - kv 3: llama.embedding_length u32 = 2048
llama_model_loader: - kv 4: llama.block_count u32 = 24
llama_model_loader: - kv 5: llama.feed_forward_length u32 = 5504
llama_model_loader: - kv 6: llama.rope.dimension_count u32 = 128
llama_model_loader: - kv 7: llama.attention.head_count u32 = 16
llama_model_loader: - kv 8: llama.attention.head_count_kv u32 = 16
llama_model_loader: - kv 9: llama.attention.layer_norm_rms_epsilon f32 = 0.000001
llama_model_loader: - kv 10: llama.rope.freq_base f32 = 100000.000000
llama_model_loader: - kv 11: llama.rope.scaling.type str = linear
llama_model_loader: - kv 12: llama.rope.scaling.factor f32 = 4.000000
llama_model_loader: - kv 13: general.file_type u32 = 1
llama_model_loader: - kv 14: tokenizer.ggml.model str = llama
llama_model_loader: - kv 15: tokenizer.ggml.tokens arr[str,32022] = ["!", "\"", "#", "$", "%", "&", "'", ...
llama_model_loader: - kv 16: tokenizer.ggml.scores arr[f32,32022] = [-1000.000000, -1000.000000, -1000.00...
llama_model_loader: - kv 17: tokenizer.ggml.token_type arr[i32,32022] = [1, 1, 1, 1, 1, 1, 1, 1, 1, 1, 1, 1, ...
llama_model_loader: - kv 18: tokenizer.ggml.bos_token_id u32 = 32013
llama_model_loader: - kv 19: tokenizer.ggml.eos_token_id u32 = 32021
llama_model_loader: - kv 20: tokenizer.ggml.padding_token_id u32 = 32014
llama_model_loader: - kv 21: tokenizer.ggml.add_bos_token bool = true
llama_model_loader: - kv 22: tokenizer.ggml.add_eos_token bool = false
llama_model_loader: - kv 23: tokenizer.chat_template str = {% if not add_generation_prompt is de...
llama_model_loader: - type f32: 49 tensors
llama_model_loader: - type f16: 170 tensors
llama_model_quantize_internal: meta size = 767616 bytes
[ 1/ 219] output.weight - [ 2048, 32256, 1, 1], type = f16, quantizing to q6_K .. size = 126.00 MiB -> 51.68 MiB
[ 2/ 219] token_embd.weight - [ 2048, 32256, 1, 1], type = f16, quantizing to q5_0 .. size = 126.00 MiB -> 43.31 MiB | hist: 0.040 0.018 0.028 0.043 0.061 0.082 0.101 0.114 0.117 0.109 0.092 0.072 0.052 0.035 0.022 0.016
...
[ 218/ 219] blk.9.attn_v.weight - [ 2048, 2048, 1, 1], type = f16, quantizing to q5_0 .. size = 8.00 MiB -> 2.75 MiB | hist: 0.040 0.017 0.028 0.042 0.060 0.081 0.101 0.116 0.121 0.109 0.091 0.071 0.051 0.034 0.022 0.016
[ 219/ 219] output_norm.weight - [ 2048, 1, 1, 1], type = f32, size = 0.008 MB
llama_model_quantize_internal: model size = 2568.38 MB
llama_model_quantize_internal: quant size = 891.50 MB
llama_model_quantize_internal: hist: 0.040 0.017 0.028 0.043 0.061 0.082 0.101 0.114 0.118 0.109 0.092 0.071 0.051 0.035 0.022 0.016main: quantize time = 9300.54 ms
main: total time = 9300.54 ms
进行测试
./main -m ../DeepSeek-Coder/models/1.3b-q5_0.gguf -n 256 -t 18 --repeat_penalty 1.0 --color -i -r "User:" -f ./prompts/chat-with-bob.txt -ngl 20
加载模型失败.
warning: not compiled with GPU offload support, --n-gpu-layers option will be ignored
warning: see main README.md for information on enabling GPU BLAS support
Log start
main: build = 1 (231ae28)
main: built with cc (Ubuntu 9.4.0-1ubuntu1~20.04.2) 9.4.0 for x86_64-linux-gnu
main: seed = 1710571501
llama_model_loader: loaded meta data with 25 key-value pairs and 219 tensors from ../DeepSeek-Coder/models/1.3b-q5_0.gguf (version GGUF V3 (latest))
llama_model_loader: Dumping metadata keys/values. Note: KV overrides do not apply in this output.
llama_model_loader: - kv 0: general.architecture str = llama
llama_model_loader: - kv 1: general.name str = models
llama_model_loader: - kv 2: llama.context_length u32 = 16384
llama_model_loader: - kv 3: llama.embedding_length u32 = 2048
llama_model_loader: - kv 4: llama.block_count u32 = 24
llama_model_loader: - kv 5: llama.feed_forward_length u32 = 5504
llama_model_loader: - kv 6: llama.rope.dimension_count u32 = 128
llama_model_loader: - kv 7: llama.attention.head_count u32 = 16
llama_model_loader: - kv 8: llama.attention.head_count_kv u32 = 16
llama_model_loader: - kv 9: llama.attention.layer_norm_rms_epsilon f32 = 0.000001
llama_model_loader: - kv 10: llama.rope.freq_base f32 = 100000.000000
llama_model_loader: - kv 11: llama.rope.scaling.type str = linear
llama_model_loader: - kv 12: llama.rope.scaling.factor f32 = 4.000000
llama_model_loader: - kv 13: general.file_type u32 = 8
llama_model_loader: - kv 14: tokenizer.ggml.model str = llama
llama_model_loader: - kv 15: tokenizer.ggml.tokens arr[str,32022] = ["!", "\"", "#", "$", "%", "&", "'", ...
llama_model_loader: - kv 16: tokenizer.ggml.scores arr[f32,32022] = [-1000.000000, -1000.000000, -1000.00...
llama_model_loader: - kv 17: tokenizer.ggml.token_type arr[i32,32022] = [1, 1, 1, 1, 1, 1, 1, 1, 1, 1, 1, 1, ...
llama_model_loader: - kv 18: tokenizer.ggml.bos_token_id u32 = 32013
llama_model_loader: - kv 19: tokenizer.ggml.eos_token_id u32 = 32021
llama_model_loader: - kv 20: tokenizer.ggml.padding_token_id u32 = 32014
llama_model_loader: - kv 21: tokenizer.ggml.add_bos_token bool = true
llama_model_loader: - kv 22: tokenizer.ggml.add_eos_token bool = false
llama_model_loader: - kv 23: tokenizer.chat_template str = {% if not add_generation_prompt is de...
llama_model_loader: - kv 24: general.quantization_version u32 = 2
llama_model_loader: - type f32: 49 tensors
llama_model_loader: - type q5_0: 169 tensors
llama_model_loader: - type q6_K: 1 tensors
llm_load_vocab: SPM vocabulary, but newline token not found: _Map_base::at! Using special_pad_id instead.llm_load_vocab: mismatch in special tokens definition ( 9/32022 vs 22/32022 ).
llm_load_print_meta: format = GGUF V3 (latest)
llm_load_print_meta: arch = llama
llm_load_print_meta: vocab type = SPM
llm_load_print_meta: n_vocab = 32022
llm_load_print_meta: n_merges = 0
llm_load_print_meta: n_ctx_train = 16384
llm_load_print_meta: n_embd = 2048
llm_load_print_meta: n_head = 16
llm_load_print_meta: n_head_kv = 16
llm_load_print_meta: n_layer = 24
llm_load_print_meta: n_rot = 128
llm_load_print_meta: n_embd_head_k = 128
llm_load_print_meta: n_embd_head_v = 128
llm_load_print_meta: n_gqa = 1
llm_load_print_meta: n_embd_k_gqa = 2048
llm_load_print_meta: n_embd_v_gqa = 2048
llm_load_print_meta: f_norm_eps = 0.0e+00
llm_load_print_meta: f_norm_rms_eps = 1.0e-06
llm_load_print_meta: f_clamp_kqv = 0.0e+00
llm_load_print_meta: f_max_alibi_bias = 0.0e+00
llm_load_print_meta: n_ff = 5504
llm_load_print_meta: n_expert = 0
llm_load_print_meta: n_expert_used = 0
llm_load_print_meta: pooling type = 0
llm_load_print_meta: rope type = 0
llm_load_print_meta: rope scaling = linear
llm_load_print_meta: freq_base_train = 100000.0
llm_load_print_meta: freq_scale_train = 0.25
llm_load_print_meta: n_yarn_orig_ctx = 16384
llm_load_print_meta: rope_finetuned = unknown
llm_load_print_meta: model type = ?B
llm_load_print_meta: model ftype = Q5_0
llm_load_print_meta: model params = 1.35 B
llm_load_print_meta: model size = 891.50 MiB (5.55 BPW)
llm_load_print_meta: general.name = models
llm_load_print_meta: BOS token = 32013 '<|begin▁of▁sentence|>'
llm_load_print_meta: EOS token = 32021 '<|EOT|>'
llm_load_print_meta: UNK token = 0 '!'
llm_load_print_meta: PAD token = 32014 '<|end▁of▁sentence|>'
llm_load_tensors: ggml ctx size = 0.08 MiB
llama_model_load: error loading model: create_tensor: tensor 'token_embd.weight' has wrong shape; expected 2048, 32022, got 2048, 32256, 1, 1
llama_load_model_from_file: failed to load model
llama_init_from_gpt_params: error: failed to load model '../DeepSeek-Coder/models/1.3b-q5_0.gguf'
main: error: unable to load model
看错误llama_model_load: error loading model: create_tensor: tensor 'token_embd.weight' has wrong shape; expected 2048, 32022, got 2048, 32256, 1, 1
应该是跟前面修改的vocab-size
有关。
这篇关于deepseek-coder模型量化的文章就介绍到这儿,希望我们推荐的文章对编程师们有所帮助!