本文主要是介绍长大还是大吃一惊:AI芯片初创企业面临问题吗?,希望对大家解决编程问题提供一定的参考价值,需要的开发者们随着小编来一起学习吧!
“Startups” in semiconductor chip design space had been a rarity since the dot-com crash in the early 2000s. Chip design requires massive development cost as design cycles are multi-year long with dependence on (1) expensive EDA (Electronic Design Automation) tools for design and (2) foundries for manufacturing — both of which are highly advanced technologies with very few players in the world. Long design cycles from the conception of an architecture specification to its tapeout (tapeout is when a chip design is frozen & sent to a semiconductor foundry for manufacturing) plus time it takes to develop a SW stack to program new architectures further delays the point of revenue generation for such companies. Initial high investment costs with delayed revenue and delayed improvement in gross-margin had caused major market consolidations after the 2000 dot-com crash and had made semiconductor chip startups less attractive for venture capital funding.
自从2000年代初的互联网泡沫大潮以来,半导体芯片设计领域的“初创公司”就很少见。 芯片设计需要大量的开发成本,因为设计周期长达数年之久,这取决于(1)用于设计的昂贵EDA( 电子设计自动化 )工具和(2)用于制造的代工厂-两者都是高度先进的技术,几乎没有参与者世界。 从架构规范的概念到其流水线( 流水线是指芯片设计被冻结并发送到半导体代工厂进行制造 )的漫长设计周期,再加上开发SW堆栈以对新架构进行编程所需的时间,这进一步延迟了收益点这样的公司的一代。 最初的高投资成本,延迟的收入和延迟的毛利改善导致了2000年互联网泡沫大跌之后的主要市场整合,并使半导体芯片初创公司对风险资本的吸引力下降。
However the advent of AI in the last ~8 years with its unique computational requirement has exposed newer opportunities for domain-specific ASICs to be, once again, a high-risk-high-gain proposition for venture funding.
然而,在过去的约8年中,AI的出现以其独特的计算要求为特定领域的ASIC再次提供了新的机会,使其再次成为风险投资的高风险,高收益的提议。
Introduction of Tensor Processing Unit (TPU), which is a chip designed specifically for Deep Learning (DL constitutes most of AI these days), by Google in 2017 demonstrated the possibility of building a domain-specific chip solution by a new player (new in terms of building ASICs) and cross validated the presence of a lucrative market for investors. As seen in the chart below, 190% growth in funding for AI chip startups in 2017 and the steady increase since then (the 2020 column only represents funding in the first quarter of a pandemic year) is a reflection of renewed VC confidence in semiconductor chip startups. (Note: only logic processor startups in US are included in the chart below to highlight the point. SW, IP, memory, display drivers, sensors, MEMS, RF, power management, discretes or optoelectronics & processors are excluded.)
张量处理单元(TPU),这是专为深度学习而设计的芯片的介绍(DL这些天构成最AI的 ),由谷歌在2017年展示了一个新的玩家建立一个特定领域的芯片解决方案的可能性( 新中构建ASIC的条款 )和cross验证了对投资者有利可图的市场的存在。 如下图所示,2017年AI芯片初创公司的资金增长190%,此后稳步增长( 2020栏仅代表大流行年份的第一季度的资金 ),这反映了风险投资对半导体芯片的重新信心创业公司。 (注意:下表仅列出了美国的逻辑处理器初创公司,以突出说明这一点。不包括软件,IP,内存,显示驱动器,传感器,MEMS,RF,电源管理,分立器件或光电器件和处理器。)
Interestingly, majority of the new DL chip startups (interchangeably called AI chip startups), have targeted their chip solutions for Inference instead of Training.
有趣的是,大多数新的DL芯片初创公司( 可互换地称为AI芯片初创公司 )已将其芯片解决方案的目标定位为推理而不是培训。
As a brief recap, Deep Learning consists of two phases:
简要概述一下,深度学习包含两个阶段:
Training : A deep neural network is trained to perform a task by showing a large number of data examples. For example, a neural network is trained on 1.2 million images of the ImageNet dataset to learn 1000 categories of objects (eg: peacock, cricket, buckle etc.).
训练:通过显示大量数据示例来训练深层神经网络执行任务。 例如,在ImageNet数据集的120万幅图像上训练了一个神经网络,以学习1000种类别的对象(例如:Kong雀,板球,带扣等)。
- Inference : A trained deep neural network is deployed to perform the learned task on new data. For example, above network is deployed on Pinterest to tag new images which the network has never seen before. 推论:部署了训练有素的深度神经网络,以对新数据执行学习的任务。 例如,以上网络部署在Pinterest上以标记网络从未见过的新图像。
Training is usually at least 3x more computationally intensive than Inference.
训练的计算强度通常比推理至少高3倍。
Training = forward propagation + data gradient + weight gradient
Inference = forward propagation
Training & Inference phases have significantly different computational and memory requirements and hence it is possible to have chip solutions uniquely tailored for each task.
训练和推论阶段对计算和存储的要求有很大不同,因此有可能针对每个任务专门定制芯片解决方案。
Training occurs in cloud or on giant supercomputers in premise today. Inference, dependent on the use case, can either occur in a datacenter or on edge devices closer to the point of data collection such as near IoT sensors or CCTVs for smart analytics or on autonomous robots.
今天,培训是在云端或大型超级计算机上进行的。 取决于用例,推理可以发生在数据中心或更靠近数据收集点的边缘设备上,例如在IoT传感器附近或用于智能分析的CCTV或自动机器人上。
Inference is the lower hanging fruit for a startup aspiring to target both inference and training solutions. This is proven by the fact that the first generation AI chip from new players such as Google, Graphcore, Habana (acquired by Intel), Tenstorrent among others were designed to target Inference and the subsequent generations are/will be meant for Training.
对于有志于同时针对推理和培训解决方案的初创公司而言,推理是低下的果实。 这证明了以下事实:来自Google , Graphcore , Habana (被Intel收购), Tenstorrent等新玩家的第一代AI芯片旨在针对推理,而后代将/将用于培训。
However the bulk of other AI chip startups are targeting only Edge Inference for numerous reasons, some of which are explained below.
但是,由于许多原因,其他大多数AI芯片初创公司都只将 Edge Inference作为目标,下面将对其中一些原因进行说明。
部署量 (Volume of deployment)
One of the primary reasons is the sheer volume of the Edge Inference market which can be much larger than Training. A trained model is deployed for Inference on numerous endpoints based on the use case whereas Training can be performed repeatedly on the same supercomputer on-premise or in-cloud. For example, once a model for a self-driving car has been trained on a giant cluster, it will be deployed for inference on the AI chips in millions of cars. Or for another example, once an automatic speech recognition model has been trained, it will be deployed for low latency inference across millions of smart devices like speakers, mobiles, refrigerators etc.
主要原因之一是Edge Inference市场的庞大数量,其规模可能比Training大得多。 根据用例在许多端点上部署了经过训练的模型以进行推理,而可以在内部或云端的同一台超级计算机上重复执行训练。 例如,一旦在巨型集群上训练了自动驾驶汽车的模型,就将其部署到数百万辆汽车的AI芯片上进行推理。 再举一个例子,一旦训练了自动语音识别模型,它将被部署用于在数百万个智能设备(如扬声器,手机,冰箱等)上进行低延迟推理。
多样的约束=探索更多利基 (Diverse constraints = More Niches to explore)
Based on different deployment use cases, inference chips can have different constraints or requirements.
根据不同的部署用例,推理芯片可能具有不同的约束或要求。
The compute horsepower, acceptable chip area (form-factor) and power budget of an Inference chip performing movie recommendations on Netflix in cloud is quite different from an Inference chip in an autonomous bot doing pizza delivery which in turn is quite different from an Inference chip in a smart-assistant enabled speaker or mobile phone.
在云中的Netflix上执行电影推荐的Inference芯片的计算能力,可接受的芯片面积(形状因数)和功率预算与进行披萨交付的自动机器人中的Inference芯片有很大不同,而这又与Inference芯片有很大不同在启用了智能辅助功能的扬声器或手机中。
In the past, most of AI Inference used to happen in cloud but privacy concerns regarding personal data and demand for lower latencies (higher responsiveness) have created a push for inference to be performed on edge devices directly such as on mobile devices, smart wearables, smart speakers or autonomous cars. This decentralization of inference computation from cloud to edge devices has opened up the opportunity for different players to focus specialization on different deployment tasks. The edge devices can choose to specialize only in specific endpoint applications unlike cloud devices which are required to demonstrate high efficiency performance on wider range of generic workloads running in a datacenter.
过去,大多数AI推理曾经发生在云中,但是有关个人数据和对较低延迟(更高响应度)的需求引起的隐私问题促使人们在直接在移动设备,智能可穿戴设备,智能扬声器或自动驾驶汽车。 从云到边缘设备的推理计算的分散,为不同的参与者提供了将专业化重点放在不同的部署任务上的机会。 边缘设备可以选择只专注于特定的终结点应用程序,而与云设备不同,云设备需要在数据中心中运行的更大范围的通用工作负载上展现出高效的性能。
Datacenter Training is a harder battle for startups. High compute horsepower and flexibility of performance across a diverse set of workloads requires well integrated and mature software stack with very wide user adoption — which is even harder than designing new chip architectures. Data Centers, amounting to massive infrastructure & maintenance costs, require a very high vote of confidence in the vendor which usually favors giant and old legacy providers over startups.
对于初创企业而言,数据中心培训是一场艰苦的战斗。 强大的计算能力和跨各种工作负载的性能灵活性要求具有高度集成性和成熟度的软件堆栈,并需要广泛的用户采用-这比设计新芯片架构还要难。 数据中心的基础设施和维护成本很高,需要对供应商给予很高的信任度,这通常比起初创企业更青睐大型和老式遗留提供商。
However, the proliferation of different niche edge AI use cases has created newer market segments which didn’t exist before and are ripe with opportunities for startups to capture.
但是,不同的利基边缘AI用例的激增创造了较新的细分市场,这些细分市场以前是不存在的,并且已经为初创企业提供了捕捉的机会。
Edge-based AI chipset market has been estimated to bring in 3.5x more revenue than cloud-based AI chips market by 2025 — $51.6 billion in revenue versus $14.6 billion respectively as seen in the chart below.
据估计,到2025年,基于边缘的AI芯片组市场的收入将比基于云的AI芯片市场多3.5倍-收入为516亿美元,而收入为146亿美元,如下图所示。
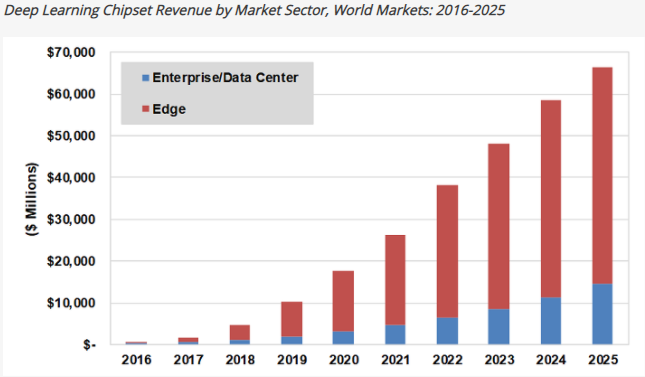
Datacenter chip space is bound to stay heavily guarded by giants such as NVIDIA, AMD and Intel who already have decades of lead in SW expertise and enterprise partnerships. To gain a slice of this pie, a startup will have to demonstrate at least 10x improvement over existing chip solutions across a diversity of workloads and establish wide SW user adoption for customers to drop existing reliable partnerships — which is incredibly hard when the monetary runway is short & when existing players with larger resources are demonstrating continued performance improvement generation-after-generation; a problem currently being faced by one of the highest funded AI chip startup Graphcore.
数据中心芯片空间必定会受到NVIDIA,AMD和Intel等巨头的严密保护,这些巨头已经在软件专业知识和企业合作伙伴方面拥有数十年的领先地位。 为了发挥这一作用,一家初创公司必须证明其在各种工作负载下比现有芯片解决方案至少提高10倍,并建立广泛的软件用户采用率,以使客户放弃现有的可靠合作伙伴关系。简短&当现有的具有较大资源的参与者展示出持续改进的一代又一代的表现时; 资金最高的AI芯片初创公司Graphcore当前面临的问题。
Edge-based AI chip market on the other hand has many new niches to explore and build partnerships with, each of which requires specialization across narrow workloads which is a more achievable goal when funding is limited. Edge-based AI chip market will continue to have high initial development cost but the promise of high volume deployment and opportunities to create & capture newer market niches would be like baking your own pie — which should be an obvious choice for hardware chip entrepreneurs & VCs.
另一方面,基于边缘的AI芯片市场有许多新的利基可供探索和建立合作伙伴关系,每个领域都需要在狭窄的工作负载上进行专业化,而在资金有限的情况下,这是一个更可实现的目标。 基于边缘的AI芯片市场将继续保持较高的初始开发成本,但是大量部署的承诺以及创建和捕捉新市场利基的机会就像烤制自己的馅饼一样-对于硬件芯片企业家和VC来说,这应该是显而易见的选择。
翻译自: https://medium.com/swlh/grow-the-pie-or-take-a-slice-question-facing-ai-chip-startups-ca8af38841ab
相关文章:
这篇关于长大还是大吃一惊:AI芯片初创企业面临问题吗?的文章就介绍到这儿,希望我们推荐的文章对编程师们有所帮助!