本文主要是介绍Facial Micro-Expressions:An Overview 阅读笔记,希望对大家解决编程问题提供一定的参考价值,需要的开发者们随着小编来一起学习吧!
Proceedings of the IEEE上一篇微表情相关的综述,写的很详细。从心理学与计算机两个领域阐述了微表情生成的原因与相关算法,值得仔细研读。
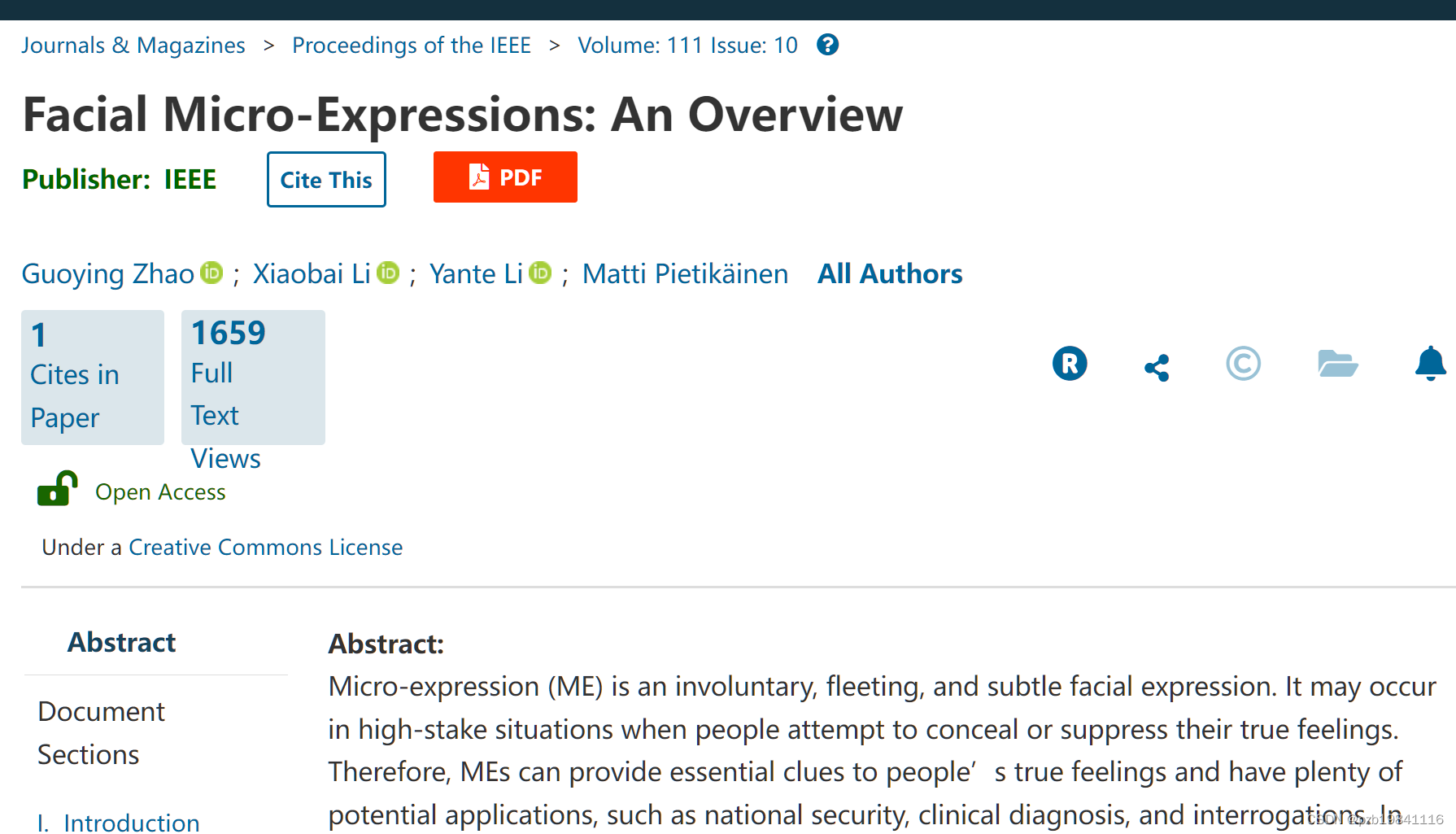
摘要:
Four main tasks in ME analysis arespecifically discussed,including ME spotting,ME recognition,ME action unit detection,and ME generation in terms of theapproaches,advance developments,and challenges.
综述着重讨论ME分析的四个主要任务,分别是ME的检测、识别、动作单元检测和生成,涵盖了方法、进展和挑战。
1.简介
Affective computingaims to endow computers the human-like capabilities toobserve,understand,and interpret human affects,refer-ring to feeling,emotion,and mood
情感计算的目标是赋予计算机类似于人类的能力,观察、理解和解释人类的情感,涉及到情感、情绪和心境。
However,people may try to conceal their true feelings under certainconditions when people want to avoid losses or gain bene-fits
面部微表情(MEs)在某些条件下可能会出现,因为人们在想要避免损失或获得利益时可能会试图掩饰其真实感情。
We startfrom the discovery of the ME phenomenon and explo-rations in psychological studies and then track the studiesin cognitive neuroscience about the neural mechanismbeneath the behavioral phenomenon.After that,we intro-duce the technological studies of ME in the computervision field,from the early attempts to advanced machinelearning methods for recognition,spotting,related AUdetection tasks,and ME synthesis or generation.
从ME现象的发现和心理学研究开始,追踪认知神经科学研究中关于行为现象背后神经机制的研究。然后,介绍了在计算机视觉领域的技术研究,从早期尝试到用于识别、检测相关动作单元任务以及ME合成或生成的先进机器学习方法。
2.心理学中的微表情研究
The research of MEs can be traced back to 1966 whenHaggard and Isaacs[22]first reported finding one kindof short-lived facial behavior in psychotherapy that istoo fast to be observed with the naked eyes.
微表情(MEs)的研究可以追溯到1966年,Haggard和Isaacs首次报告在心理治疗中发现了一种短暂的面部行为,肉眼观察太快。
This phenomenonwas also found by Ekman and Friesen[23]one year afterand named it micro-facial expression.
Ekman和Friesen在一年后发现了相同的现象,并将其命名为"微表情"。
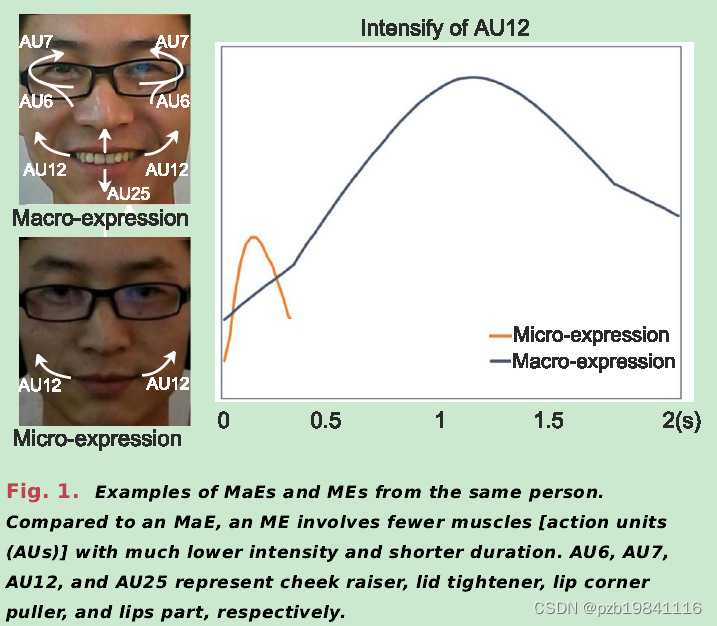
FACS is a comprehensive tool to measure facial movementsobjectively[32].FACS encodes and taxonomizes eachvisually discernible facial muscle movement according tohuman face anatomical structure,which is called AU.
FACS是一个全面的工具,用于客观测量面部运动。它根据人类面部解剖结构对每个可视辨别的面部肌肉运动进行编码和分类,称为动作单元(AU)。
The neural mechanisms of MEs are also explored andexplained.Two neural pathways[34]originating fromdifferent brain areas are involved to mediate facial expres-sions.One pathway originated from the subcortical areas(i.e.,the amygdala),which drives involuntary emotionalexpression,including facial expressions and other bodilyresponses,while the other pathway is originated from thecortical motor strip,which drives voluntary facial actions.
ME的神经机制涉及两个起源于不同脑区的神经通路,其中一个来自亚皮质区域(如杏仁核),驱动不自主的情感表达,而另一个来自皮质运动区,驱动自愿的面部动作。
when peopleare feeling strong emotions but try to control/suppresstheir expressions in high-stake situations,the two path-ways meet in the middle and engage in a neural“tugof war”over the control of the face,which may lead tofleeting leakage of MEs.
当人们感受强烈情绪但试图在高风险情境中控制/抑制表达时,这两个通路在中间相遇,进行神经上的“拉锯战”,可能导致ME的瞬时泄漏。
3.计算机视觉的早起尝试
The ME spotting task aims to detect and locate MEoccurrences from context clips,and the recognition taskaims to classify MEs into emotional categories.
ME检测任务旨在从上下文剪辑中检测和定位ME事件,而识别任务旨在将ME分类为情感类别。
posed ME datasets
姿态ME数据集是指一些早期的微表情(ME)研究中所使用的数据集,其中参与者被要求通过模仿或表演某种面部表情,而这种表情并非在真实、自然的情境下产生,而是被设计或要求的。
the posed MEs are different from real,naturallyoccurred MEs on their appearances in both spatial andtemporal domains
即姿态ME与实际自然发生的ME在空间和时间领域的外观上存在差异
4.数据集
常用数据集
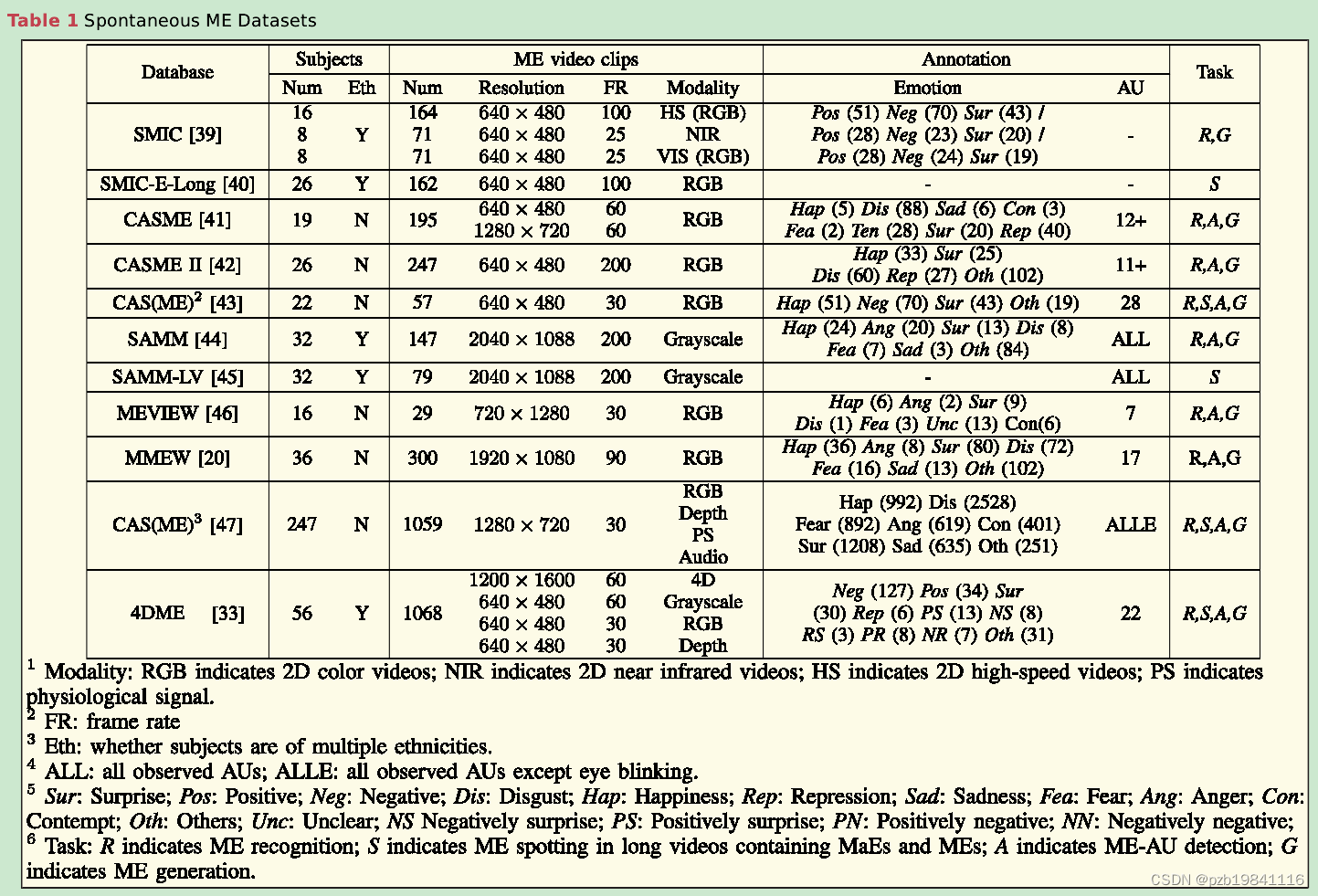
One common way to induce spontaneous MEs is to usemovie clips with strong emotional clips.
诱导自发微表情的一种常见方法是使用带有强烈情感内容的电影片段。
Recently,inspired by the paradigm of mockcrime in psychology
受心理学中模拟犯罪范式的启发
Earlier spontaneous ME datasets only con-tain frontal 2-D videos as they were relatively easy to col-lect and analyze,which leads to the fact that most existingME methods can only analyze frontal faces and are inca-pable of dealing with challenges in real-world applications,such as illumination variation,occlusion,and pose vari-ations.
早期的自发微表情(ME)数据集主要包含前方的2D视频,因为这相对容易收集和分析,导致大多数现有的ME方法只能分析前方面部,并无法处理现实世界应用中的挑战,如光照变化、遮挡和姿态变化。
Currently,the fast technological developmentof 3-D scanning makes recording and reconstructing high-quality 3-D facial videos possible.
由于3D扫描技术的快速发展,目前录制和重建高质量的3D面部视频已经成为可能。
各种2D与3D的微表情数据集样本
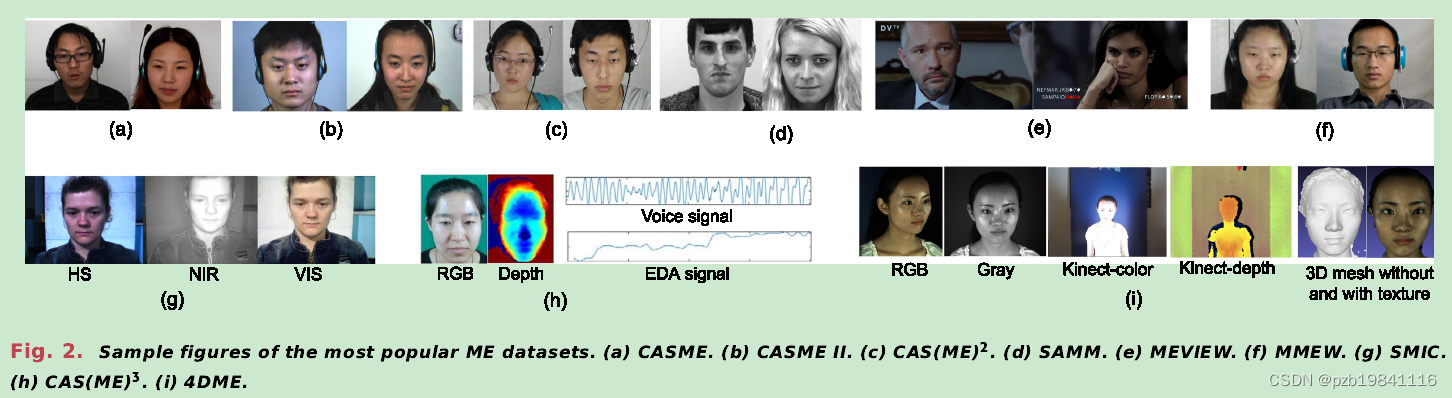
For researching ME spotting,some ME datasetshave been extended by including non-micro-frames beforeand after the annotated ME samples to generate longervideos,
针对微表情定位任务,一些数据集通过在标注的ME样本之前和之后包含非微观帧来生成更长的视频
5.微表情分析的计算方法
Given raw collected videos,preprocessing is usually thefirst step to be performed,and then,the ME clips can bedetected and located through ME spotting.After that,MiXand ME-AU detection can be carried out as separate or jointtasks.ME generation can synthesize either long videos,including mixed MEs and MaEs or short clips with justMEs,which is expected to benefit different ME analyses.
给定原始采集的视频,预处理通常是首要执行的步骤,然后可以通过ME定位来检测和定位ME剪辑。之后,MiX和ME-AU检测可以作为独立或联合任务进行。ME生成可以合成包含混合ME和MaE或仅有ME的短剪辑,有望有助于不同的ME分析。
微表情分析的流程
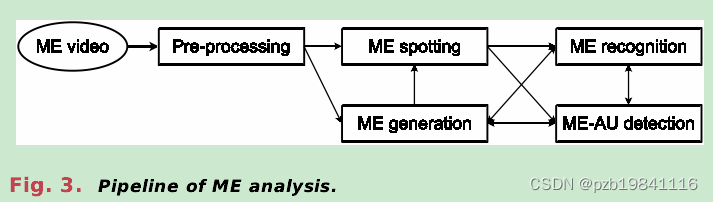
A.预处理
Face detection is the first step inan ME analysis system.Face detection finds the face
人脸检测是ME分析系统中的第一步,它找到脸部位置并去除背景。
Face registration aims to align each detected face to a refer-ence face according to key facial landmarks so that to alle-viate pose variation and head movement problems.
由于MEs非常微妙,容易受到头部姿态变化的影响,因此人脸注册旨在将每个检测到的脸与参考脸相对齐,以减轻姿态变化和头部运动问题。
Motion magnification aims toenhance the intensity level of subtle motions in videos,e.g.,the invisible trembling of a working machine.It wasfound to be helpful for ME analysis tasks and employed asa special preprocessing step.
运动放大旨在增强视频中微小运动的强度,对于ME分析任务非常有帮助。
Besides low intensity,anotherchallenge of ME analysis is that ME clips are very short andwith varying lengths,which is not good for clip-based anal-ysis,especially when recording MEs with a relatively low-speed camera.Temporal interpolation solves this issue byinterpolating sequences into a designated length.
由于ME剪辑非常短且长度变化,不利于基于剪辑的分析,尤其是在使用相对低速摄像头记录MEs时。时间插值通过将序列插值到指定长度来解决这个问题,有助于提取稳定的时空特征。
B.输入
微表情分析中,输入数据的各种格式
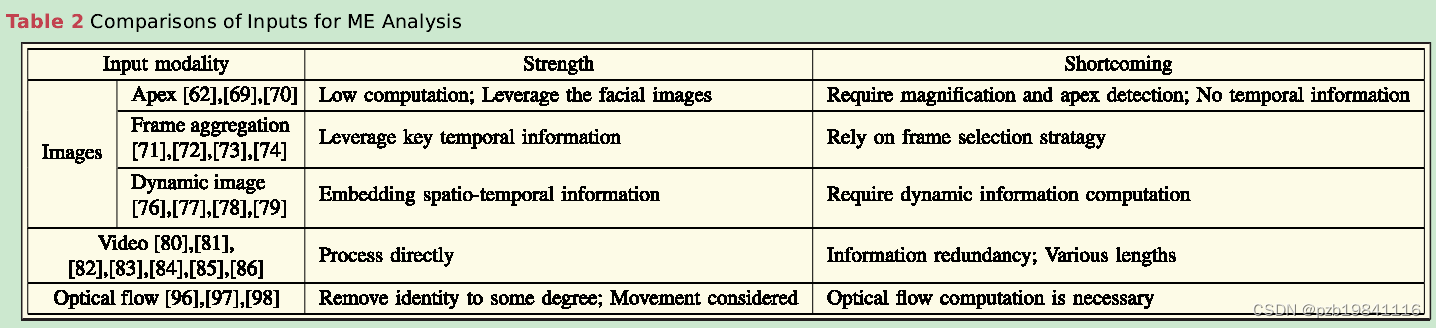
A large number of facial expression recog-nition studies are based on static images because of theconvenience of image processing and the availability ofmassive amounts of facial images.
静态图像是面部表情识别(MER)研究的常见输入形式,因为图像处理方便且大量面部图像易获取。
Some works[71],[72]proposed touse multiple key ME frames as inputs.
为了维持时空信息,有研究提出使用多个关键ME帧作为输入。
Considering thatthe dynamic image can summarize appearance and subtledynamics into an image,multiple MER methods[76],[77],[78],[79]employed dynamic image as input andachieved promising performance.The dynamic image cansimultaneously consider spatial and temporal information,and keep computational efficiency by processing only oneimage.
动态图像能够将外观和微妙的动态信息汇总到一幅图像中,一些MER方法采用动态图像作为输入,取得了良好的性能。动态图像可以同时考虑空间和时间信息,通过处理单个图像保持计算效率。
It considers spatial and continuous temporal infor-mation simultaneously and can be processed directly with-out extra operations.
ME视频剪辑是MER研究中常见的输入形式,同时考虑了空间和连续的时间信息。
Optical flow estimates the local movement betweenimages by computing the direction and magnitude ofpixel movement in image sequences
光流是另一种广泛使用的输入形式,通过计算图像序列中像素移动的方向和幅度来估计局部运动。
C.微表情检测
ME spotting is one of the maintasks in automatic ME analysis,which identifies temporallocations(sometimes also the spatial places in faces)ofMEs in video clips.
ME Spoting是自动ME分析中的主要任务之一,其目标是识别视频剪辑中ME的时间位置(有时也包括面部的空间位置)
The onset is the first frame in which the MEmotion is first discriminable.The frame with the highestmotion intensity in the ME clip is the apex frame.The offsetis the frame marking the end of the motion.
起始帧是首次可以辨别ME运动的帧,顶点帧是ME剪辑中运动强度最高的帧,结束帧标志着运动的结束。
Traditional algorithms are usu-ally training-free,heuristic,and spot MEs by comparingfeature differences in a sliding window with fixed-lengthtime[61],[62],[111].The location of MEs can bedetermined by a thresholding method.
传统算法通常是无需训练、基于启发式的,通过在固定长度时间的滑动窗口中比较特征差异来识别MEs。ME的位置可以通过阈值方法确定。
LBP[61],[111],HOG[61],and optical flow[103],[112],[113],as shownin Fig.4,are the commonly used features for ME spotting,
LBP、HOG和光流是ME Spoting中常用的特征,这些特征在实际应用中具有重要作用。
Main directional max-imal difference analysis(MDMD)was proposed[104],[113]to spot MEs based on the magnitude of maximaldifference in the main direction of optical flow.
主方向最大差异分析(MDMD)[104],[113]是一种基于光流主方向上最大差异幅度的ME定位方法,使用了滑动窗口和基于块的划分。
frequency-based ME spotting methods[62],[69]werepresented to spot the apex frame in the ME sequence byexploiting information in the frequency domain,whichcan reflect the rate of facial changes.
为了在长视频中区分MaEs和MEs,提出了基于频率的ME定位方法,通过利用频率域中的信息来定位ME序列中的顶点帧,这可以反映面部变化的速率。
The strength of feature difference-based approaches isthat the approaches consider the temporal characteristicaccording to the size of the sliding window,and the spot-ting results can be simply obtained by setting thresholds.
特征差异方法的优势: 这些方法考虑了时间特性,通过设置阈值就可以简单地获得定位结果。
as they are heuristic,mainly based on practicalexperience regarding,e.g.,a threshold in the feature dif-ference value,the spotting results can be easily influencedby the other facial movements with similar intensity orduration,such as eye blinks.Thus,it is hard to distinguishMEs from other similar facial movements with feature-difference-based ME spotting methods.
特征差异方法的劣势: 由于这些方法主要是基于实际经验的启发式方法,因此定位结果很容易受到其他面部运动的影响,这些运动具有相似的强度或持续时间,例如眨眼。因此,通过特征差异进行ME Spoting的方法很难将MEs与具有相似特征的其他面部运动区分开。
machine learning-basedmethods were developed to tell apart different facial move-ments,which regards ME spotting as a binary classificationof non-ME and ME frames.In general,these methodsfirst extract features of each ME frame,and a classifier isutilized to recognize the ME frames.
基于机器学习的方法,将ME定位视为非ME和ME帧的二元分类问题。这些方法通常首先提取每个ME帧的特征,然后使用分类器识别ME帧。
Inspired by the successful application of deep learning
深度学习也用到了MESpot中
the heuristic ME spottingmethods are mostly based on thresholds to classify MEsand non-MEs,which are weak to distinguish the MEsfrom other facial movements,such as eye blinks.
基于启发式的ME定位方法主要依赖于阈值来对ME和非ME进行分类。这些方法较难区分ME和其他面部运动,如眨眼等。
Themachine learning-based methods can recognize differentfacial movements by training classifiers.However,the per-formance of ME spotting is restricted by the small-scale MEdatasets and unbalanced ME and non-ME samples.
机器学习方法可以通过训练分类器来识别不同的面部运动,从而实现ME定位。然而,ME定位的性能受到小规模ME数据集和ME与非ME样本不平衡的限制。
With the increase in ME spotting research,variousevaluation protocols have been proposed,using differenttraining and testing sets,and various metrics,such as thearea under the curve(AUC),the receiver operating charac-teristic(ROC)curve,mean absolute error(MAE),accuracy,recall,and F1-score[18]
随着ME定位研究的增加,提出了各种评估协议,使用不同的训练和测试集以及各种度量标准,如AUC、ROC曲线、MAE、准确性、召回率和F1分数。
In addi-tion,multiple studies attempted to explore spotting MEsand MaEs simultaneously in long videos.Due to the pres-ence of noise,irrelevant movements,and mixed MEs andMaEs,it is very challenging to learn discriminative featureson limited datasets and accurately locate the various MEsand MaEs that should be further studied.
一些研究尝试在长视频中同时定位ME和MaE。由于存在噪声、无关的运动以及混合的ME和MaE,对于有限的数据集学习具有区分性特征并准确定位各种ME和MaE是非常具有挑战性的,需要进一步研究。
D.微表情识别
Due to most MEdatasets with limited samples,handcrafted features arewidely researched in MER.
由于大多数ME数据集的样本有限,MER领域广泛研究采用手工设计的特征。
传统方法的效果
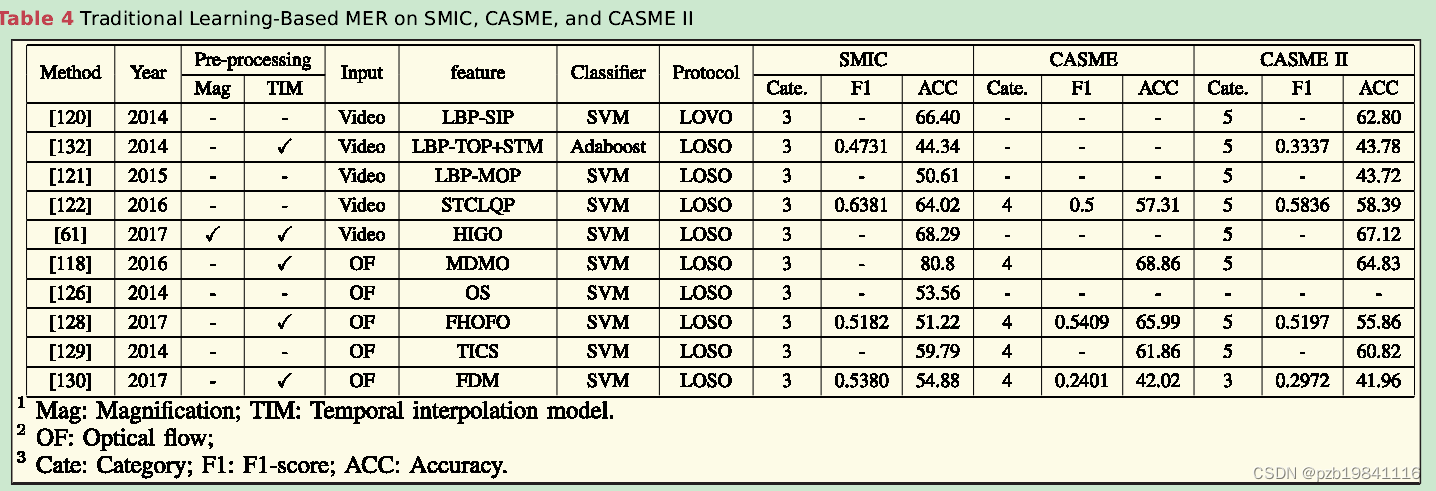
LBP-TOP is a texture descriptor thresh-olding the neighbors of each pixel with a binary codein spatial and temporal dimensions.Most of the MERresearch employs the LBP-TOP as their baseline due toits computational simplicity
LBP-TOP是一种纹理描述符,在时空维度上用二进制代码阈值化每个像素的邻域。由于计算简单,大多数MER研究采用LBP-TOP作为其基准。
The histogram of gradients(HOG)is one ofthe most widely used features for its ability to describethe edges in an image with geometric invariance[123],
梯度是另一种广泛用于MER的特征。梯度直方图(HOG)是其中最常用的特征之一,因为它能够以几何不变性描述图像中的边缘。
several works designed feature descriptorsbased on optical flow for MER.
一些研究基于光流设计特征描述符用于MER。
there are descriptors rep-resenting MEs in other views,such as color[129]andfacial geometry[119],[130]
还有一些使用其他视图表示ME的描述符,如颜色和面部几何。这些方法采用不同的颜色空间或面部几何结构,以增强MER的准确性。
以下是几种常用的手工特征的可视化效果
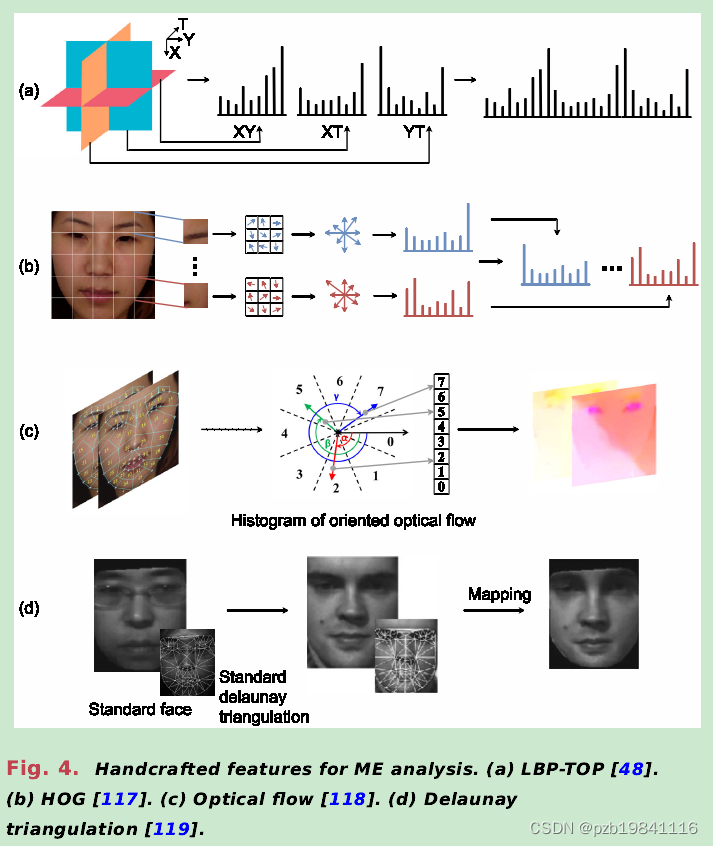
SVM is the most widely used classifierbecause of its robustness,accuracy,and effectiveness espe-cially when the training samples are limited.
SVM是最广泛使用的分类器之一,尤其在训练样本有限的情况下表现出色。
MEs have small-scale datasets and lowintensity,which makes MER based on deep learning hard.
近年来,研究者尝试使用深度学习方法进行ME分析,但由于ME数据集规模较小、强度较低,这使得基于深度学习的MER面临困难。
Fine-tuning deep networkspretrained on large datasets can effectively avoid the over-fitting problem caused by small-scale ME datasets
Fine-tuning预训练模型,避免小规模数据集引起的过拟合问题。
multiple works adopted multistream net-work structures to extract multiview features from var-ious inputs.
多视图学习则采用多流网络结构,从不同输入中提取多视图特征。
To further explore the temporalinformation of MEs,several works[88],[153],[154]cascaded CNN and RNN or LSTM to extract features fromindividual frames of ME sequence and capture the facialevolution of MEs.
采用CNN和RNN或LSTM级联结构,从ME序列的各帧提取特征,捕捉ME的面部演变。该方法旨在更有效地利用ME的时序信息。
multiple research developed multitask learning for bet-ter MER by leveraging different side tasks
利用多任务学习改善MER性能,整合不同辅助任务的信息。
Other methods leverage the knowledge ofother tasks through transfer learning.Directly fine-tuningon a pretrained model is the simplest.Besides fine-tuning,knowledge distillation is also widely applied to MER[70].
通过迁移学习和领域自适应等方法,利用其他任务的知识来提升MER性能。直接微调和知识蒸馏是常用方法。
Besides utilizing more data,many studies designedeffective shallow networks for MER to avoid overfitting
除了利用更多的数据外,许多研究为MER设计了有效的浅层网络以避免过拟合
In order to emphasize learningon RoIs and reduce the influence of information unre-lated to MEs,multiple works introduced attention mod-ules
为了强调对RoIs的学习,减少与MEs无关信息的影响,许多工作引入了注意力模块
The graph convolutional network(GCN)has beenverified to effectively model the semantic relationships.
图卷积网络( GCN )已经被验证可以有效地对语义关系进行建模。
以下是集中常用的基于深度学习的微表情识别模型
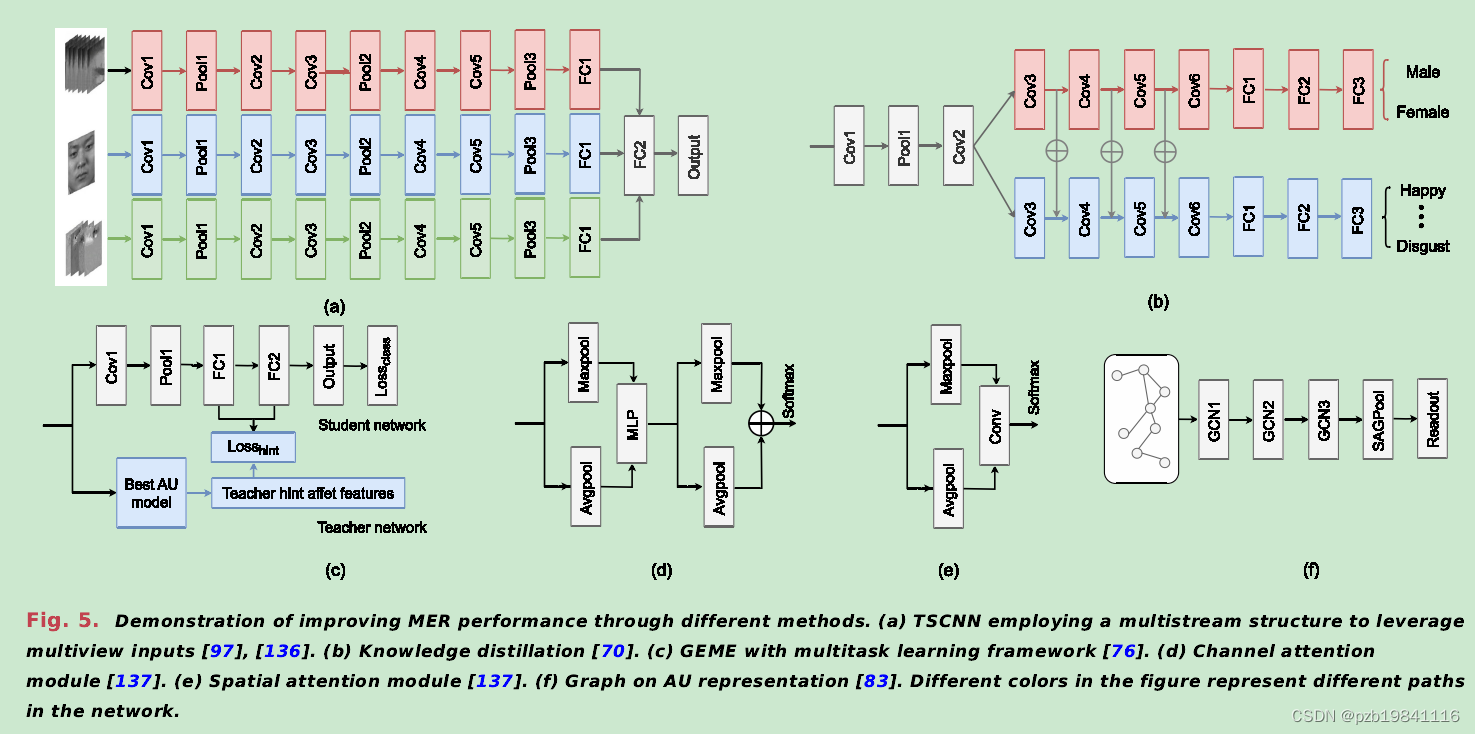
Contrastive loss[179],triplet loss,and center loss[180]were introduced to MER to increase intraclass compactnessand interclass separability of MEs[69],[160].
采用不同的损失函数,如对比损失、三元损失、中心损失、聚类损失等,以提高ME表示的紧凑性和可分离性。
Thefocal loss was employed to alleviate the issue by focusingon misclassified and hard samples
由于某些ME很难触发,导致数据集分布不均衡,采用聚焦损失等方法来缓解这一问题。
The main challenge for robust MER ishow to effectively extract discriminative representations.
鲜明的MER挑战在于如何有效提取具有区分性的表示。
Handcrafted features are low-level representations thatare able to effectively describe the texture,color,and soon while being weak in extracting high-level semanticinformation.In contrast,deep learning-based features areabstract high-level representations.
传统的手工特征适用于低级别的纹理、颜色等描述,而深度学习特征则提供抽象的高级表示。
The performancesof MER are influenced by various factors,such as prepro-cessing,features,and network structure.
MER性能受到多个因素的影响,包括预处理、特征和网络结构。
以下是深度学习在微表情识别上的效果
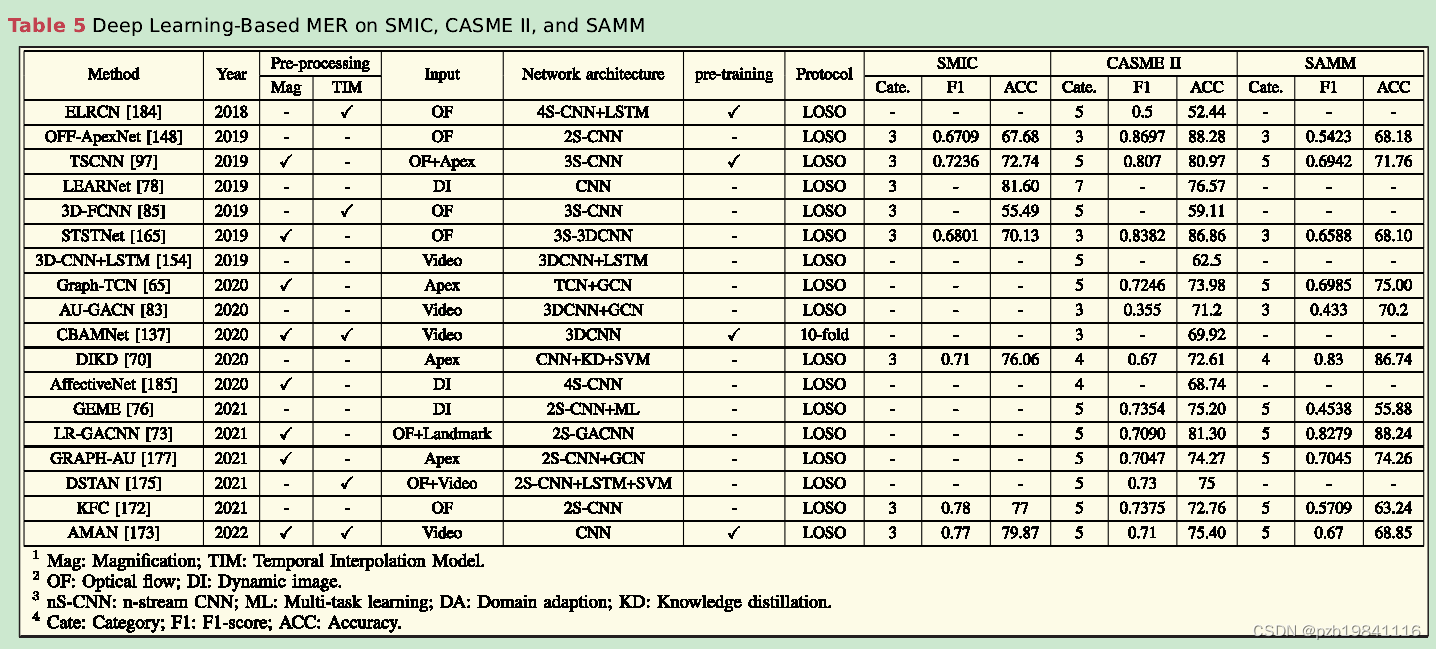
In general,the preprocessing step could benefit the MERfor both traditional learning and deep learning-based MERapproaches.
实验结果显示,预处理对传统学习和深度学习的MER方法都有益处。
下图是时序插帧与运动增强对微表情识别结果的影响
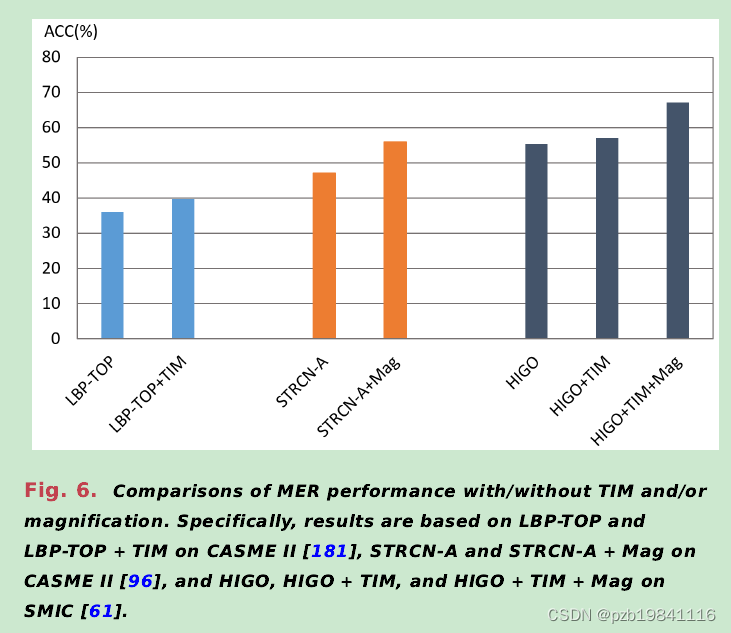
magnification and TIM can benefit MER.However,the suitable magnification and temporal interpo-lation factor should be further studied.
TIM(Temporal Interpolation Method)和放大对MER性能有益。然而,需要进一步研究适当的放大和时间插值因子。
Current MER research designed shallow networks orleveraged massive MaE images to solve the data limitationproblem.
解决数据限制问题的方法包括设计浅层网络或利用大量MaE图像。
the perfor-mance is still far from satisfying for real-world applica-tions.
当前方法在小规模ME数据集上的性能仍不足以满足实际应用需求。
more effective blocks andstructures should be developed to learn discriminative MEfeatures with fewer parameters in the future.
对于使用浅层网络的方法,未来需要开发更有效的模块和结构,以用更少的参数学到具有区分性的ME特征。
considering the appearance difference betweenMEs and MaEs,transfer learning methods should be fur-ther studied to solve the domain shift problem.Lever-aging information from other related tasks,such as ageestimation and identity classification,could be considered.
对于利用大量MaE图像的迁移学习方法,需要进一步研究解决领域转移问题的方法,可以考虑利用其他相关任务的信息,如年龄估计和身份分类。
unsupervised learning and semisupervisedlearning[182][183]are promising future directions forMER,as they could leverage the massive unlabeled images.
无监督学习和半监督学习是未来MER研究的有前途的方向,因为它们可以充分利用大量未标记的图像。
E.ME-AU检测
The study of facial expression recog-nition indicates that AU detection is able to facilitatecomplex facial expression analysis,and developing facialexpression recognition with AU analysis simultaneouslycould boost the facial expression recognition performance
面部表情识别的研究表明,动作单元(AU)的检测有助于复杂的面部表情分析,同时开展AU分析的面部表情识别研究能够提升性能。
Common facial AU datasets containa large number of facial samples and identity diver-sity
AU检测是一种复杂的细粒度面部分析,通常包含大量面部样本和身份多样性
Exist-ing ME-AU detection research proposed to utilize theMaEs[194]or specific ME characteristics,such as subtlelocal facial movements[66],[195],[196],which are dis-cussed in more detail in the following,to overcome theseissues.
为克服ME数据不足,一些研究采用MaEs或特定ME特征(如微妙的局部面部运动)进行ME-AU检测。
An intra-contrastive and intercontrastive learning method was pro-posed to enlarge and utilize the contrastive informationbetween the onset and apex frames to obtain the dis-criminative representation for low-intensity ME-AU detec-tion
针对ME特征的细微AU建模,提出了一种基于对比学习的方法,以扩大和利用起始和顶点帧之间的对比信息,获得低强度ME-AU检测的判别性表示。
To effectively learn local facial movement andleverage relationship information between different facialregions to enhance the robustness of ME-AU detection,a spatial and channel attention module was designedto capture subtle ME-AUs by exploring high-order statis-tics
另一方法设计了空间和通道关注模块,通过探索高阶统计信息,有效地学习局部面部运动,并增强ME-AU检测的鲁棒性。
AU检测的准确率
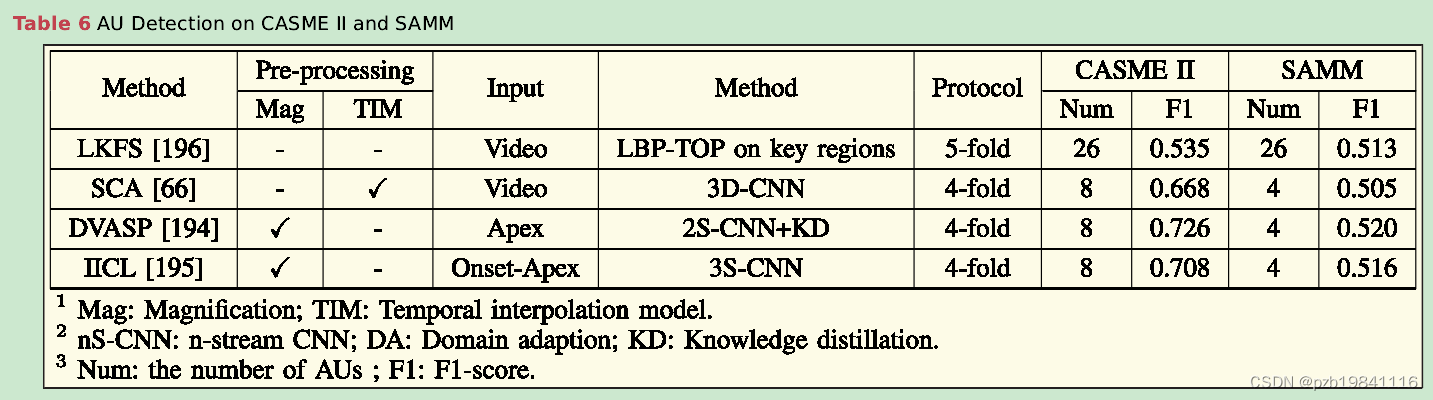
the ME-AU detec-tion suffers from small-scale and extremely unbalanceddatasets as some AUs coexist and the occurrence of someAUs is very low.
目前ME-AU检测的研究工作和性能受到限制,主要表现在数据规模小、分布极不均衡、AUs相互关联等方面。
F.ME生成
annotating MEs needs certified FACS codersto check videos frame by frame several times,which istime-consuming and labor-intensive.
标注ME需要经过认证的FACS编码员逐帧检查视频多次,这既耗时又劳动密集,导致ME分析中样本有限且分布不均衡。
utilizing GAN to generate facial images.However,theMEs are subtle and rapid,and straightforwardly utilizingGANs cannot generate satisfying MEs.
为解决ME分析中的问题,研究人员开始探索使用生成对抗网络(GAN)生成面部图像。然而,由于ME是微妙且迅速的,直接使用GAN难以生成令人满意的ME。
CurrentME generation methods mainly leverage AUs or facial keypoints.
近期的ME生成方法主要利用动作单元(AU)或面部关键点。
Since facial expressions are constituted by AUs[209],[210],AU-ICGAN[83],FAMGAN[211],and MiE-X[199]introduced GANs based on AUs to generate MEs.
一些方法,如AU-ICGAN、FAMGAN和MiE-X,基于AUs生成MEs。
ME生成对网络预训练的影响如下所示
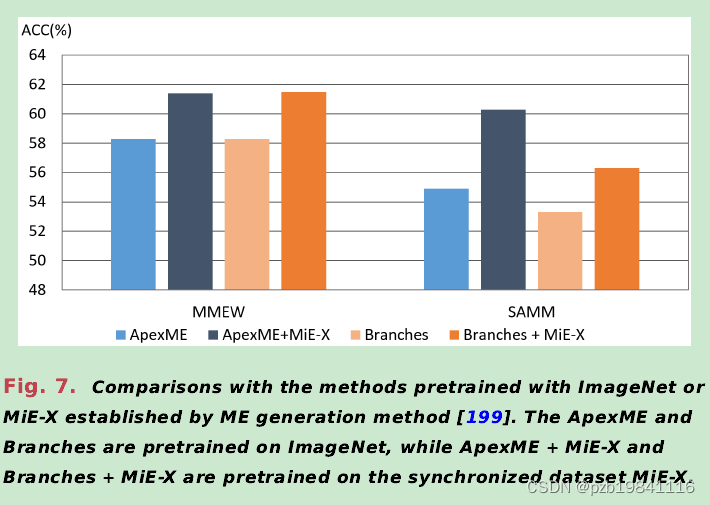
Other works estimated facial motion using key pointsto generate ME images
其他方法使用面部关键点估计面部运动生成ME图像
Currently,the quality of the generated MEs is not realisticenough.However,with further investigation,it is expectedthat they could be not only helpful in other ME analyses,such as MER,ME spotting,and ME AU detection,but alsoin ME synthesis for augmented reality,HCI,and so on.
ME生成是ME分析的新方向,尽管当前生成的ME质量尚不够逼真,但进一步的研究预计将在其他ME分析方面发挥作用,如MER、ME检测和ME AU检测,同时也可用于增强现实、人机交互等领域。
6.公开挑战与未来方向
Computational methodsdeveloped for ME analysis,including spotting,recognition,and generation,could help in multiple use cases e.g.,convert emotion understanding and expert training.
微表情分析的计算方法,包括检测、识别和生成,可以在多种情境下帮助,例如转化情感理解和专家培训。
A.技术层面的挑战
Data annotationis one key issue that hinders the development of large-scaleME datasets as it requires certified expertise and is verytime-consuming.Moreover,some emotion,such as fear,is difficult to be evoked,which causes data imbalanceissues.
数据标注是限制大规模ME数据集发展的一个关键问题,因为它需要经过认证的专业知识,并且非常耗时。此外,某些情感(如恐惧)很难唤起,这导致数据不平衡问题。
show that people canproduce mixed expressions in their daily life when two ormore“basic”emotions are felt.
人们在日常生活中可以产生混合表情,即在感受到两种或更多“基本”情感时。
Compound MEscould be rare and more challenging to study,but,as theyreflect the specific emotional states that practically exist,they should be considered and not ignored in future MEstudies.
对于复合ME的研究较少,但由于它们反映了实际存在的特定情感状态,因此在未来的ME研究中应该予以考虑。
Leveraging complementary information frommultiple modalities can also enhance ME analysis for abetter understanding of human’s covert emotions.
利用来自多个模态的互补信息也可以增强ME分析,更好地理解人类的隐蔽情感。
it is natural and often that MaEsand MEs coexist and even overlap[23]with each other.
在实际应用中,MaEs和MEs自然而然地可能共存甚至重叠。
Future studies should dig deeper to exploremore challenging situations where MaEs and MEs overlapwith each other,which would be a substantial step towardthe accurate understanding of human emotion in realisticsituations.
未来的研究应更深入地探讨MaEs和MEs相互叠加的更具挑战性的情境,这将是朝向准确理解真实情境中人类情感的重要一步。
ME analysis methods builton the basis of constrained settings might not generalizewell to wild environments.Effective algorithms for analyz-ing MEs in unconstrained settings with pose changes andillumination variations must be developed in the future.
在受限设置的基础上构建的ME分析方法可能不太适用于在带有姿势变化和光照变化的非受限环境中。未来必须开发在不受约束的环境中分析ME的有效算法。
B.伦理问题
ME data contain facial videosthat are sensitive data that must be considered for privacyprotection to avoid potential leakage of the participants’personal information.
ME数据包含面部视频,这是一种敏感数据,必须考虑隐私保护,以避免潜在的参与者个人信息泄露。
Newtechnology should consider its fairness and validity amongthe general population with diverse ages,gender,culture,ethnicity,and so on.For ME studies,this issue needs to beaddressed from four aspects.
新技术应考虑在年龄、性别、文化、种族等方面在总体人口中的公平性和有效性。对于ME研究,这个问题需要从四个方面解决。
Legislation should be furtherdeveloped to define specific rules to regulate the use ofME data and technologies.
应进一步通过立法明确具体规则,规范ME数据和技术的使用。
7.总结
This article offers a comprehen-sive review of the development of micro-expressions withinthe realm of computer vision.Instead of solely focusingon the introduction of machine-learning techniques formicro-expression detection and recognition,this overviewencompasses the exploration of micro-expression analy-sis from its roots in psychology and early endeavors incomputer vision to the diverse range of contemporarycomputational methods.
本文全面回顾了计算机视觉领域中微表情的发展。与仅仅关注介绍微表情检测和识别的机器学习技术不同,这一概述包括了微表情分析的探索,从其起源于心理学的根基和早期的计算机视觉尝试,到当代计算方法的多样范围。
这篇关于Facial Micro-Expressions:An Overview 阅读笔记的文章就介绍到这儿,希望我们推荐的文章对编程师们有所帮助!