本文主要是介绍FATE —— 二.1.1 Homo-NN二进制分类任务,希望对大家解决编程问题提供一定的参考价值,需要的开发者们随着小编来一起学习吧!
说明
FATE版本为1.10.0单机部署版,win10+centos7
本教程允许您快速开始使用Homo NN。默认情况下,您可以在与其他FATE算法组件相同的过程中使用Homo NN组件:使用FATE附带的读取器和转换器接口输入表数据并转换数据格式,然后将数据输入算法组件。然后NN组件将使用您定义的模型、优化器和损失函数进行训练和模型聚合。
在FATE-1.10中,正在开发中的Homo NN增加了对pytorch的支持。您可以遵循pytorch Sequential的用法,使用pytorch的内置层来定义Sequentical模型并提交模型。同时,您可以使用Pytorch附带的损失函数和优化器。
下面是一个基本的二进制分类任务Homo NN任务。有两个客户端的参与方ID分别为10000和9999,10000被指定为服务器端聚合模型。
开始
上载表格数据
一开始,我们将数据上传到FATE。我们可以使用管道直接上传数据。在这里,我们上载两个文件:来宾的brest_homo_guest.csv和主机的brest_homo_host.csv。请注意,在本教程中,我们使用的是独立版本,如果您使用的是集群版本,则需要在每台计算机上上载相应的数据。
from pipeline.backend.pipeline import PipeLine # pipeline Class# [9999(guest), 10000(host)] as client
# [10000(arbiter)] as serverguest = 9999
host = 10000
arbiter = 10000
pipeline_upload = PipeLine().set_initiator(role='guest', party_id=guest).set_roles(guest=guest, host=host, arbiter=arbiter)partition = 4# upload a dataset
path_to_fate_project = '../../../../'
guest_data = {"name": "breast_homo_guest", "namespace": "experiment"}
host_data = {"name": "breast_homo_host", "namespace": "experiment"}
pipeline_upload.add_upload_data(file="examples/data/breast_homo_guest.csv", # file in the example/datatable_name=guest_data["name"], # table namenamespace=guest_data["namespace"], # namespacehead=1, partition=partition) # data info
pipeline_upload.add_upload_data(file="examples/data/breast_homo_host.csv", # file in the example/datatable_name=host_data["name"], # table namenamespace=host_data["namespace"], # namespacehead=1, partition=partition) # data infopipeline_upload.upload(drop=1)
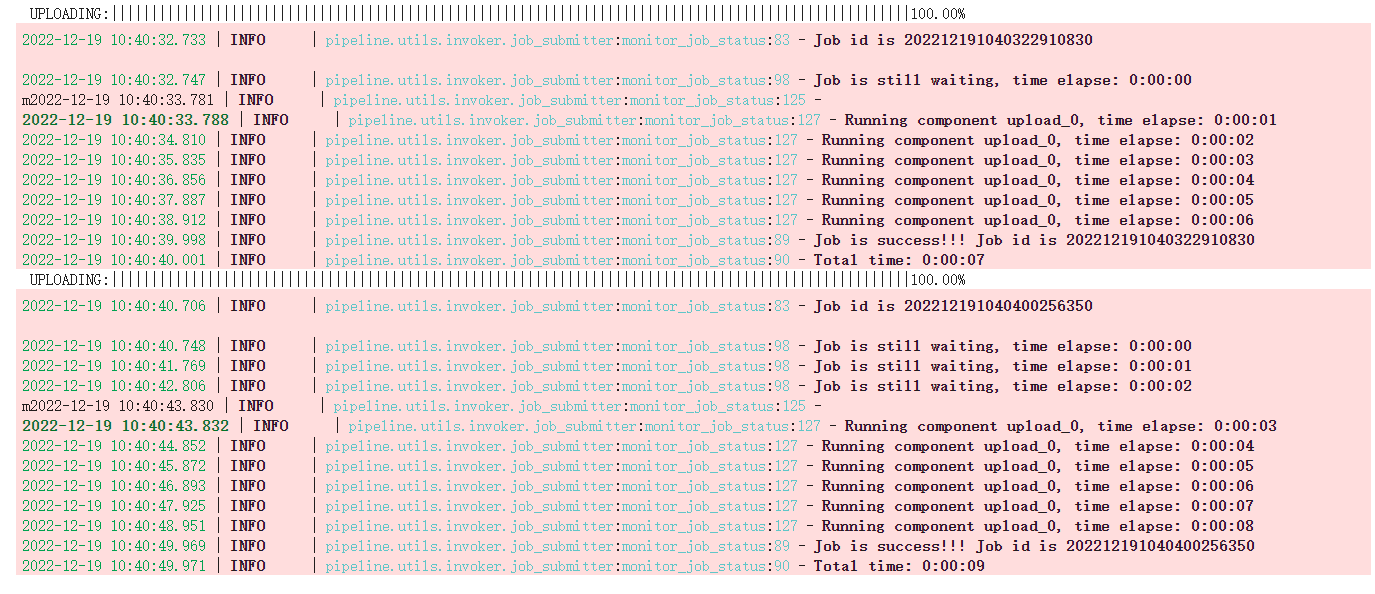
乳房数据集是一个具有30个特征的二进制数据集:
import pandas as pd
df = pd.read_csv('../examples/data/breast_homo_guest.csv') # 这里根据文件夹位置自行调整
df
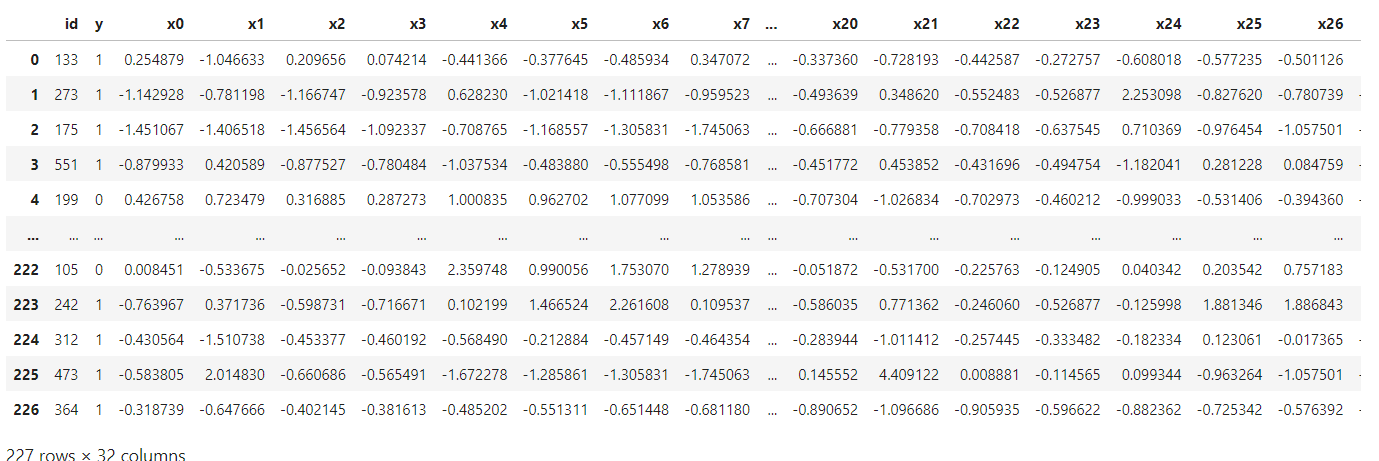
编写并执行Pipeline脚本
上传完成后,我们可以开始编写管道脚本以提交FATE任务。
导入管道组件
# torch
import torch as t
from torch import nn# pipeline
from pipeline.component.homo_nn import HomoNN, TrainerParam # HomoNN Component, TrainerParam for setting trainer parameter
from pipeline.backend.pipeline import PipeLine # pipeline class
from pipeline.component import Reader, DataTransform, Evaluation # Data I/O and Evaluation
from pipeline.interface import Data # Data Interaces for defining data flow
我们可以检查Homo NN组件的参数:
print(HomoNN.__doc__)
Parameters----------name, name of this componenttrainer, trainer paramdataset, dataset paramtorch_seed, global random seedloss, loss function from fate_torchoptimizer, optimizer from fate_torchmodel, a fate torch sequential defining the model structure
fate_torch_hook
请确保执行以下fate_torch_hook函数,该函数可以修改某些torch类,以便可以通过管道解析和提交您在脚本中定义的torch层、顺序、优化器和损失函数。
from pipeline import fate_torch_hook
t = fate_torch_hook(t)
pipeline
# create a pipeline to submitting the job
guest = 9999
host = 10000
arbiter = 10000
pipeline = PipeLine().set_initiator(role='guest', party_id=guest).set_roles(guest=guest, host=host, arbiter=arbiter)# read uploaded dataset
train_data_0 = {"name": "breast_homo_guest", "namespace": "experiment"}
train_data_1 = {"name": "breast_homo_host", "namespace": "experiment"}
reader_0 = Reader(name="reader_0")
reader_0.get_party_instance(role='guest', party_id=guest).component_param(table=train_data_0)
reader_0.get_party_instance(role='host', party_id=host).component_param(table=train_data_1)# The transform component converts the uploaded data to the DATE standard format
data_transform_0 = DataTransform(name='data_transform_0')
data_transform_0.get_party_instance(role='guest', party_id=guest).component_param(with_label=True, output_format="dense")
data_transform_0.get_party_instance(role='host', party_id=host).component_param(with_label=True, output_format="dense")"""
Define Pytorch model/ optimizer and loss
"""
model = nn.Sequential(nn.Linear(30, 1),nn.Sigmoid()
)
loss = nn.BCELoss()
optimizer = t.optim.Adam(model.parameters(), lr=0.01)"""
Create Homo-NN Component
"""
nn_component = HomoNN(name='nn_0',model=model, # set modelloss=loss, # set lossoptimizer=optimizer, # set optimizer# Here we use fedavg trainer# TrainerParam passes parameters to fedavg_trainer, see below for details about Trainertrainer=TrainerParam(trainer_name='fedavg_trainer', epochs=3, batch_size=128, validation_freqs=1),torch_seed=100 # random seed)# define work flow
pipeline.add_component(reader_0)
pipeline.add_component(data_transform_0, data=Data(data=reader_0.output.data))
pipeline.add_component(nn_component, data=Data(train_data=data_transform_0.output.data))
pipeline.add_component(Evaluation(name='eval_0'), data=Data(data=nn_component.output.data))pipeline.compile()
pipeline.fit()
打开可视化工具可以看到进程。
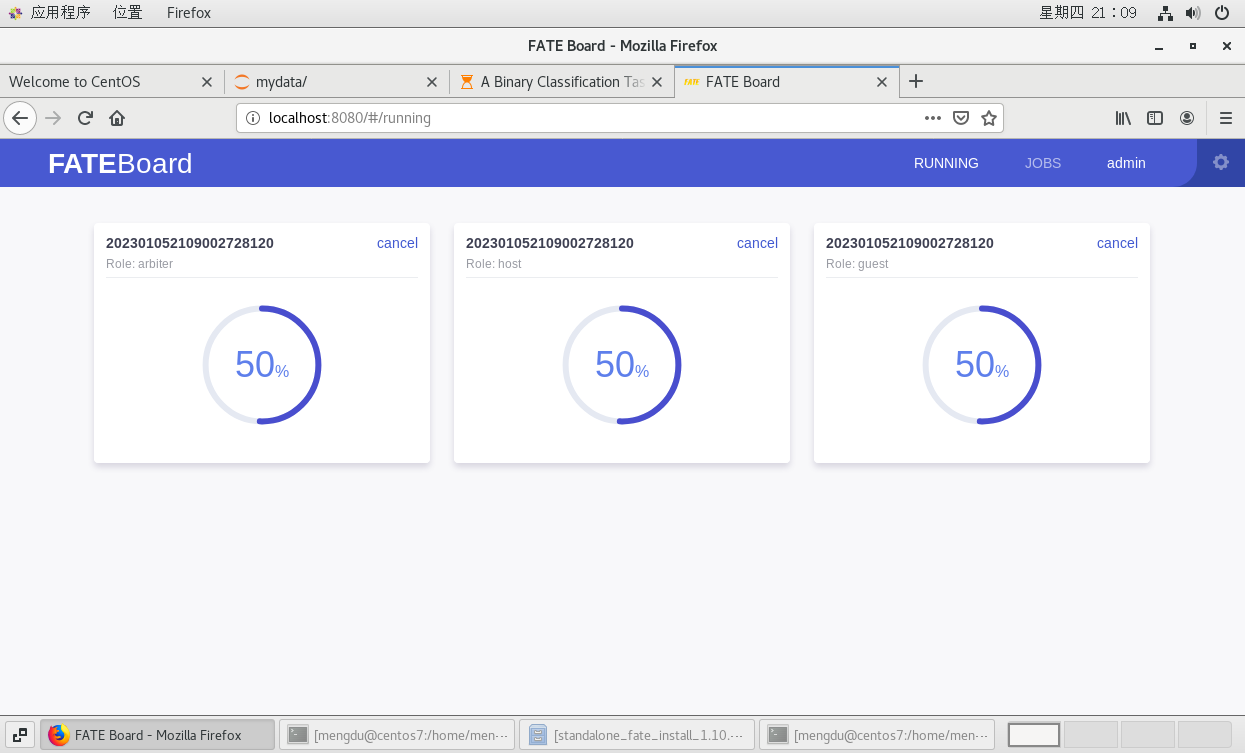
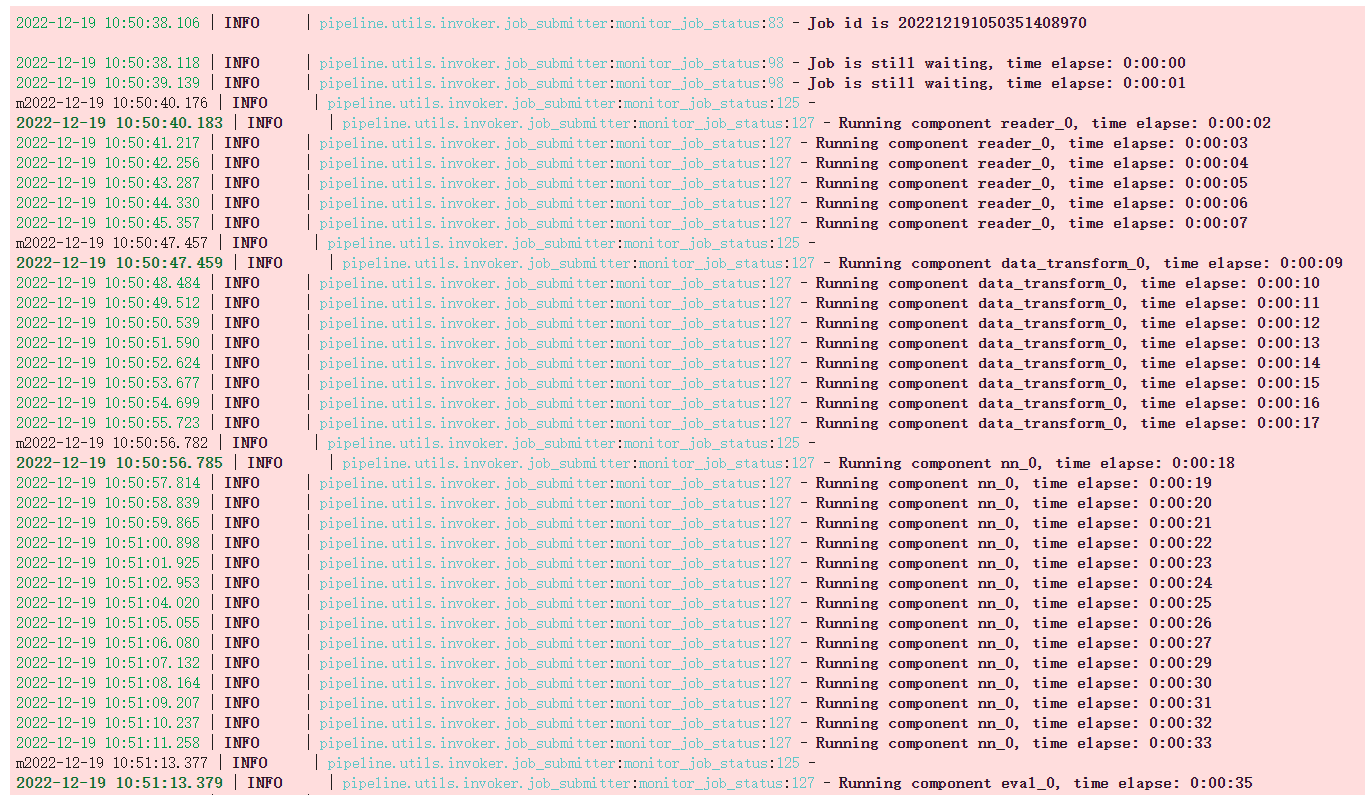
获取组件输出
# get predict scores
pipeline.get_component('nn_0').get_output_data()
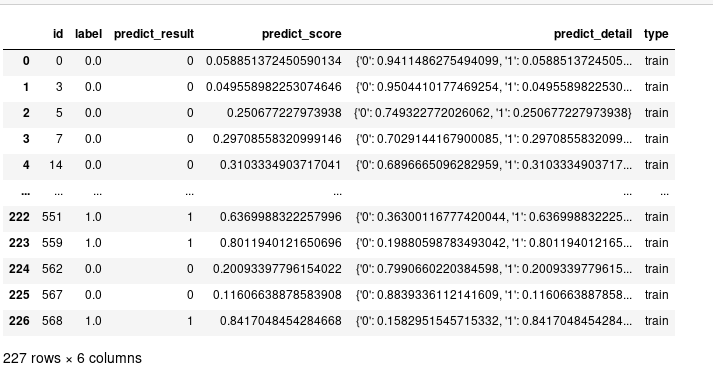
# get summary
pipeline.get_component('nn_0').get_summary()
{'best_epoch': 2,'loss_history': [0.8317702709315632, 0.683187778825802, 0.5690162255375396],'metrics_summary': {'train': {'auc': [0.732987012987013,0.9094372294372294,0.9561904761904763],'ks': [0.4153246753246753, 0.6851948051948051, 0.7908225108225109]}},'need_stop': False}
TrainerParam trainer parameter and trainer
在这个版本中,Homo NN的训练逻辑和联邦聚合逻辑都在Trainer类中实现。fedavg_trainer是FATE Homo NN的默认训练器,它实现了标准的fedavg算法。TrainerParam的功能是:
使用trainer_name=“{模块名称}”指定要使用的trainer。trainer位于federatedml.nn.homo.trainer目录中,因此您可以自定义自己的trainer。本教程将有一章专门介绍如何定制trainer
其余参数将传递给trainer的__init__()接口
我们可以在FATE中检查fedavg_trainer的参数,这些可用参数可以填写在TrainerParam中。
from federatedml.nn.homo.trainer.fedavg_trainer import FedAVGTrainer
查看FedAVGTrainer的文档以了解可用参数。提交任务时,可以使用TrainerParam传递这些参数
print(FedAVGTrainer.__doc__)
Parameters----------epochs: int >0, epochs to trainbatch_size: int, -1 means full batchsecure_aggregate: bool, default is True, whether to use secure aggregation. if enabled, will add random number mask to local models. These random number masks will eventually cancel out to get 0.weighted_aggregation: bool, whether add weight to each local model when doing aggregation. if True, According to origin paper, weight of a client is: n_local / n_global, where n_local is the sample number locally and n_global is the sample number of all clients.if False, simply averaging these models.early_stop: None, 'diff' or 'abs'. if None, disable early stop; if 'diff', use the loss difference between two epochs as early stop condition, if differences < tol, stop training ; if 'abs', if loss < tol,stop training tol: float, tol value for early stopaggregate_every_n_epoch: None or int. if None, aggregate model on the end of every epoch, if int, aggregate every n epochs.cuda: bool, use cuda or notpin_memory: bool, for pytorch DataLoadershuffle: bool, for pytorch DataLoaderdata_loader_worker: int, for pytorch DataLoader, number of workers when loading datavalidation_freqs: None or int. if int, validate your model and send validate results to fate-board every n epoch. if is binary classification task, will use metrics 'auc', 'ks', 'gain', 'lift', 'precision'. if is multi classification task, will use metrics 'precision', 'recall', 'accuracy'. if is regression task, will use metrics 'mse', 'mae', 'rmse', 'explained_variance', 'r2_score'checkpoint_save_freqs: save model every n epoch, if None, will not save checkpoint.task_type: str, 'auto', 'binary', 'multi', 'regression'. this option decides the return format of this trainer, and the evaluation type when running validation. if auto, will automatically infer your task type from labels and predict results.
到目前为止,我们已经对Homo NN有了基本的了解,并利用它完成了基本的建模任务。此外,Homo NN提供了为更高级的用例定制模型、数据集和训练器的能力。有关详细信息,请参阅提供的其他教程
这篇关于FATE —— 二.1.1 Homo-NN二进制分类任务的文章就介绍到这儿,希望我们推荐的文章对编程师们有所帮助!