本文主要是介绍Brian2 学习笔记(1)-Introduction to Brian part 1: Neurons,希望对大家解决编程问题提供一定的参考价值,需要的开发者们随着小编来一起学习吧!
原址链接:http://brian2.readthedocs.io/en/stable/introduction/index.html
第一次更新:2018年6月2日
Brian是一款面向SNN(spiking neural network)的模拟器。模拟器主体使用python语言,具有简单易学、灵活和可扩展的功能,旨在降低模拟计算的时间。
注:模拟器还有一些其他重要的特性,例如运用多核甚至计算集群的能力以及模拟内容向集群的映射简便性。这也是目前Brian模拟器最欠缺的特性(做的较好的是NEST,它是面向计算神经科学的,模型复杂度低,规模可以很大,重视对网络结构的探索;NEST is a simulator for spiking neural network models that focuses on the dynamics, size and structure of neural systems rather than on the exact morphology of individual neurons, suitable for Models of information processing, Models of network activity dynamics, Models of learning and plasticity )。Brian2还有一个弱势是每次模拟编译器都需要对模型进行分析和编译,尤其对于大规模网络的模拟是不利的。
推荐学习的方法是:安装;学习tutorial;运行例程;学习User guide;尝试自行编写或者修改模拟文件;学习完整的Documents。
引用:
[1] Goodman DF and Brette R (2009). The Brian simulator. Front Neurosci doi:10.3389/neuro.01.026.2009
[2] Stimberg M, Goodman DFM, Benichoux V, Brette R (2014). Equation-oriented specification of neural models for simulations. Frontiers Neuroinf, doi: 10.3389/fninf.2014.00006.
User guide
Units
基本单位
IS最基本的七个单位:安培amp/ampere, 千克kilogram/kilogramme, 秒second, 米metre/meter, 摩尔(6.022e236.022e23)mole/mol, 开尔文(温度)kelvin, 以及坎德拉candela.
其他常用单位: 库伦coulomb, 法拉farad, 克gram/gramme, 赫兹hertz, 焦耳joule, 升liter/ litre, 摩尔质量molar, 帕斯卡pascal, 欧姆ohm, 西蒙子siemens, 伏volt, 瓦特watt, together with 前缀prefixed versions (e.g. msiemens = 0.001*siemens) using the prefixes p, n, u, m, k, M, G, T。两个例外千克没有前缀, 米‘meter’可以加 “centi” 前缀, i.e. cmetre/cmeter。
给一个数据加上单位的方法是‘乘以’一个单位,例如‘*ms’。去除单位变为纯数字的方法有三个,最常用的是‘除以’,例如‘/ms’;第二种方法是用numpy的数列函数‘asarray’或者‘array’,例如‘asarray(speed)’;第三种方法是在变量后加‘_’,例如:
print(M.v_[0])
- 1
温度
使用基本单位制中的kelvin,在brian2中已经去掉了celsius的单位。
常数
The brian2.units.constants package provides a range of physical constants that can be useful for detailed biological models. Brian provides the following constants:
import
(重要)
from brian2 import *
- 1
A simple model
Let’s start by defining a simple neuron model. In Brian, all models are defined by systems of differential equations. Here’s a simple example of what that looks like:
tau = 10*ms
eqs = '''
dv/dt = (1-v)/tau : 1
'''
In Python, the notation '''
is used to begin and end a multi-line string. So the equations are just a string with one line per equation. The equations are formatted with standard mathematical notation, with one addition. At the end of a line you write : unit
where unit
is the SI unit of that variable. Note that this is not the unit of the two sides of the equation (which would be 1/second
), but the unit of the variable defined by the equation, i.e. in this case vv.
Now let’s use this definition to create a neuron.
G = NeuronGroup(1, eqs)
In Brian, you only create groups of neurons, using the class NeuronGroup
. The first two arguments when you create one of these objects are the number of neurons (in this case, 1) and the defining differential equations.
Let’s see what happens if we didn’t put the variable tau
in the equation:
eqs = '''
dv/dt = 1-v : 1
'''
G = NeuronGroup(1, eqs)
run(100*ms)
run(时间)表模拟运行时间
run(100*ms)
Now let’s go back to the good equations and actually run the simulation.
start_scope()tau = 10*ms
eqs = '''
dv/dt = (1-v)/tau : 1
'''G = NeuronGroup(1, eqs)
run(100*ms)
INFO No numerical integration method specified for group 'neurongroup', using method 'exact' (took 0.04s). [brian2.stateupdaters.base.method_choice]
First off, ignore that start_scope()
at the top of the cell. You’ll see that in each cell in this tutorial where we run a simulation. All it does is make sure that any Brian objects created before the function is called aren’t included in the next run of the simulation.
Secondly, you’ll see that there is an “INFO” message about not specifying the numerical integration method. This is harmless and just to let you know what method we chose, but we’ll fix it in the next cell by specifying the method explicitly.
So, what has happened here? Well, the command run(100*ms)
runs the simulation for 100 ms. We can see that this has worked by printing the value of the variable v
before and after the simulation.
start_scope()G = NeuronGroup(1, eqs, method='exact')
print('Before v = %s' % G.v[0])
run(100*ms)
print('After v = %s' % G.v[0])
Before v = 0.0
After v = 0.99995460007
By default, all variables start with the value 0. Since the differential equation is dv/dt=(1-v)/tau
we would expect after a while that v
would tend towards the value 1, which is just what we see. Specifically, we’d expect v
to have the value 1-exp(-t/tau)
. Let’s see if that’s right.
print('Expected value of v = %s' % (1-exp(-100*ms/tau)))
Expected value of v = 0.99995460007
Good news, the simulation gives the value we’d expect!
Now let’s take a look at a graph of how the variable v
evolves over time.
start_scope()G = NeuronGroup(1, eqs, method='exact')
M = StateMonitor(G, 'v', record=True)run(30*ms)plot(M.t/ms, M.v[0])
xlabel('Time (ms)')
ylabel('v');
plt.show()
注:
import numpy as np
import matplotlib.pyplot as plt
是画图的必需导入!!!
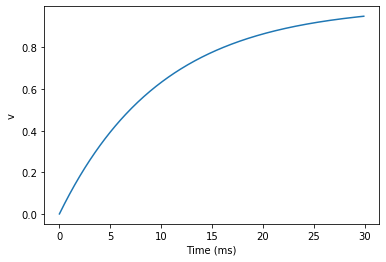
This time we only ran the simulation for 30 ms so that we can see the behaviour better. It looks like it’s behaving as expected, but let’s just check that analytically by plotting the expected behaviour on top.
start_scope()G = NeuronGroup(1, eqs, method='exact')
M = StateMonitor(G, 'v', record=0)run(30*ms)plot(M.t/ms, M.v[0], 'C0', label='Brian')
plot(M.t/ms, 1-exp(-M.t/tau), 'C1--',label='Analytic')
xlabel('Time (ms)')
ylabel('v')
legend();
plt.show()
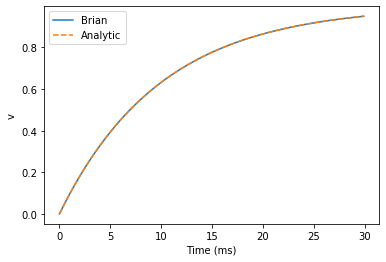
As you can see, the blue (Brian) and dashed orange (analytic solution) lines coincide.
In this example, we used the object StateMonitor
object. This is used to record the values of a neuron variable while the simulation runs. The first two arguments are the group to record from, and the variable you want to record from. We also specify record=0
. This means that we record all values for neuron 0. We have to specify which neurons we want to record because in large simulations with many neurons it usually uses up too much RAM to record the values of all neurons.
Now try modifying the equations and parameters and see what happens in the cell below.
start_scope()tau = 10*ms
eqs = '''
dv/dt = (sin(2*pi*100*Hz*t)-v)/tau : 1
'''# Change to Euler method because exact integrator doesn't work here
G = NeuronGroup(1, eqs, method='euler')
M = StateMonitor(G, 'v', record=0)G.v = 5 # initial valuerun(60*ms)plot(M.t/ms, M.v[0])
xlabel('Time (ms)')
ylabel('v');
plt.show()
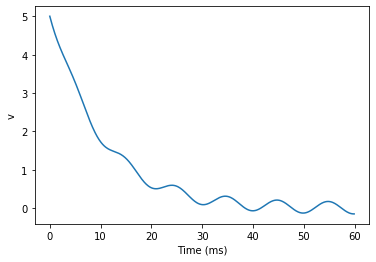
Adding spikes
So far we haven’t done anything neuronal, just played around with differential equations. Now let’s start adding spiking behaviour.
start_scope()tau = 10*ms
eqs = '''
dv/dt = (1-v)/tau : 1
'''G = NeuronGroup(1, eqs, threshold='v>0.8', reset='v = 0', method='exact')M = StateMonitor(G, 'v', record=0)
run(50*ms)
plot(M.t/ms, M.v[0])
xlabel('Time (ms)')
ylabel('v');
plt.show()
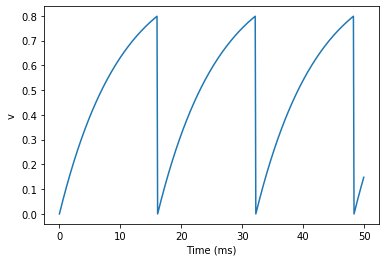
We’ve added two new keywords to the NeuronGroup
declaration: threshold='v>0.8'
and reset='v = 0'
. What this means is that when v>0.8
we fire a spike, and immediately reset v = 0
after the spike. We can put any expression and series of statements as these strings.
As you can see, at the beginning the behaviour is the same as before until v
crosses the threshold v>0.8
at which point you see it reset to 0. You can’t see it in this figure, but internally Brian has registered this event as a spike. Let’s have a look at that.
start_scope()G = NeuronGroup(1, eqs, threshold='v>0.8', reset='v = 0', method='exact')spikemon = SpikeMonitor(G)run(50*ms)print('Spike times: %s' % spikemon.t[:])
Spike times: [ 16. 32.1 48.2] ms
The SpikeMonitor
object takes the group whose spikes you want to record as its argument and stores the spike times in the variable t
. Let’s plot those spikes on top of the other figure to see that it’s getting it right.
start_scope()G = NeuronGroup(1, eqs, threshold='v>0.8', reset='v = 0', method='exact')statemon = StateMonitor(G, 'v', record=0)
spikemon = SpikeMonitor(G)run(50*ms)plot(statemon.t/ms, statemon.v[0])
for t in spikemon.t:axvline(t/ms, ls='--', c='C1', lw=3)
xlabel('Time (ms)')
ylabel('v');
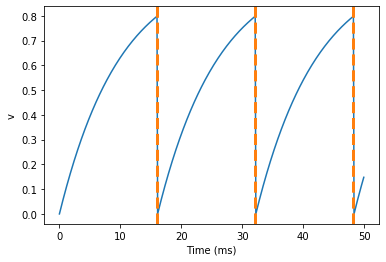
Here we’ve used the axvline
command from matplotlib
to draw an orange, dashed vertical line at the time of each spike recorded by the SpikeMonitor
.
Now try changing the strings for threshold
and reset
in the cell above to see what happens.
Refractoriness
A common feature of neuron models is refractoriness. This means that after the neuron fires a spike it becomes refractory for a certain duration and cannot fire another spike until this period is over. Here’s how we do that in Brian.
start_scope()tau = 10*ms
eqs = '''
dv/dt = (1-v)/tau : 1 (unless refractory)
'''G = NeuronGroup(1, eqs, threshold='v>0.8', reset='v = 0', refractory=5*ms, method='exact')statemon = StateMonitor(G, 'v', record=0)
spikemon = SpikeMonitor(G)run(50*ms)plot(statemon.t/ms, statemon.v[0])
for t in spikemon.t:axvline(t/ms, ls='--', c='C1', lw=3)
xlabel('Time (ms)')
ylabel('v');
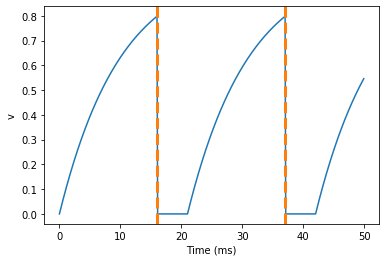
As you can see in this figure, after the first spike, v
stays at 0 for around 5 ms before it resumes its normal behaviour. To do this, we’ve done two things. Firstly, we’ve added the keywordrefractory=5*ms
to the NeuronGroup
declaration. On its own, this only means that the neuron cannot spike in this period (see below), but doesn’t change how v
behaves. In order to make v
stay constant during the refractory period, we have to add (unless refractory)
to the end of the definition of v
in the differential equations. What this means is that the differential equation determines the behaviour of v
unless it’s refractory in which case it is switched off.
Here’s what would happen if we didn’t include (unless refractory)
. Note that we’ve also decreased the value of tau
and increased the length of the refractory period to make the behaviour clearer.
start_scope()tau = 5*ms
eqs = '''
dv/dt = (1-v)/tau : 1
'''G = NeuronGroup(1, eqs, threshold='v>0.8', reset='v = 0', refractory=15*ms, method='exact')statemon = StateMonitor(G, 'v', record=0)
spikemon = SpikeMonitor(G)run(50*ms)plot(statemon.t/ms, statemon.v[0])
for t in spikemon.t:axvline(t/ms, ls='--', c='C1', lw=3)
axhline(0.8, ls=':', c='C2', lw=3)
xlabel('Time (ms)')
ylabel('v')
print("Spike times: %s" % spikemon.t[:])
Spike times: [ 8. 23.1 38.2] ms
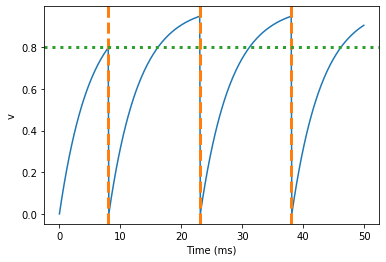
So what’s going on here? The behaviour for the first spike is the same: v
rises to 0.8 and then the neuron fires a spike at time 8 ms before immediately resetting to 0. Since the refractory period is now 15 ms this means that the neuron won’t be able to spike again until time 8 + 15 = 23 ms. Immediately after the first spike, the value of v
now instantly starts to rise because we didn’t specify (unless refractory)
in the definition of dv/dt
. However, once it reaches the value 0.8 (the dashed green line) at time roughly 8 ms it doesn’t fire a spike even though the threshold is v>0.8
. This is because the neuron is still refractory until time 23 ms, at which point it fires a spike.
Note that you can do more complicated and interesting things with refractoriness. See the full documentation for more details about how it works.
Multiple neurons
So far we’ve only been working with a single neuron. Let’s do something interesting with multiple neurons.
start_scope()N = 100
tau = 10*ms
eqs = '''
dv/dt = (2-v)/tau : 1
'''G = NeuronGroup(N, eqs, threshold='v>1', reset='v=0', method='exact')
G.v = 'rand()'spikemon = SpikeMonitor(G)run(50*ms)plot(spikemon.t/ms, spikemon.i, '.k')
xlabel('Time (ms)')
ylabel('Neuron index');
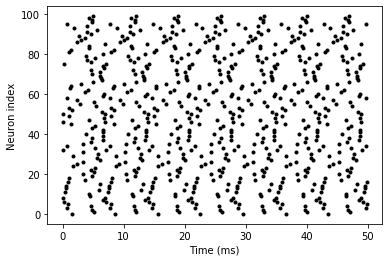
This shows a few changes. Firstly, we’ve got a new variable N
determining the number of neurons. Secondly, we added the statement G.v = 'rand()'
before the run. What this does is initialise each neuron with a different uniform random value between 0 and 1. We’ve done this just so each neuron will do something a bit different. The other big change is how we plot the data in the end.
As well as the variable spikemon.t
with the times of all the spikes, we’ve also used the variable spikemon.i
which gives the corresponding neuron index for each spike, and plotted a single black dot with time on the x-axis and neuron index on the y-value. This is the standard “raster plot” used in neuroscience.
Parameters
To make these multiple neurons do something more interesting, let’s introduce per-neuron parameters that don’t have a differential equation attached to them.
start_scope()N = 100
tau = 10*ms
v0_max = 3.
duration = 1000*mseqs = '''
dv/dt = (v0-v)/tau : 1 (unless refractory)
v0 : 1
'''G = NeuronGroup(N, eqs, threshold='v>1', reset='v=0', refractory=5*ms, method='exact')
M = SpikeMonitor(G)G.v0 = 'i*v0_max/(N-1)'run(duration)figure(figsize=(12,4))
subplot(121)
plot(M.t/ms, M.i, '.k')
xlabel('Time (ms)')
ylabel('Neuron index')
subplot(122)
plot(G.v0, M.count/duration)
xlabel('v0')
ylabel('Firing rate (sp/s)');
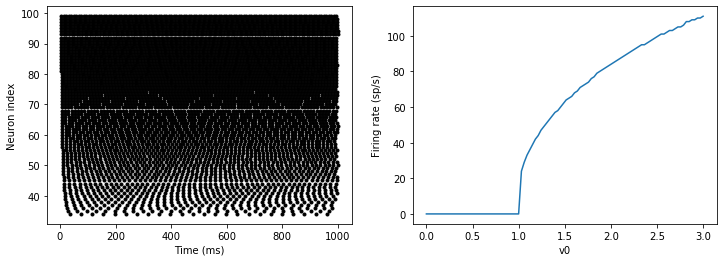
The line v0 : 1
declares a new per-neuron parameter v0
with units 1
(i.e. dimensionless).
The line G.v0 = 'i*v0_max/(N-1)'
initialises the value of v0 for each neuron varying from 0 up to v0_max
. The symbol i
when it appears in strings like this refers to the neuron index.
So in this example, we’re driving the neuron towards the value v0
exponentially, but when v
crosses v>1
, it fires a spike and resets. The effect is that the rate at which it fires spikes will be related to the value of v0
. For v0<1
it will never fire a spike, and as v0
gets larger it will fire spikes at a higher rate. The right hand plot shows the firing rate as a function of the value of v0
. This is the I-f curve of this neuron model.
Note that in the plot we’ve used the count
variable of the SpikeMonitor
: this is an array of the number of spikes each neuron in the group fired. Dividing this by the duration of the run gives the firing rate.
Stochastic neurons
Often when making models of neurons, we include a random element to model the effect of various forms of neural noise. In Brian, we can do this by using the symbol xi
in differential equations. Strictly speaking, this symbol is a “stochastic differential” but you can sort of thinking of it as just a Gaussian random variable with mean 0 and standard deviation 1. We do have to take into account the way stochastic differentials scale with time, which is why we multiply it bytau**-0.5
in the equations below (see a textbook on stochastic differential equations for more details). Note that we also changed the method
keyword argument to use 'euler'
(which stands for the Euler-Maruyama method); the 'exact'
method that we used earlier is not applicable to stochastic differential equations.
start_scope()N = 100
tau = 10*ms
v0_max = 3.
duration = 1000*ms
sigma = 0.2eqs = '''
dv/dt = (v0-v)/tau+sigma*xi*tau**-0.5 : 1 (unless refractory)
v0 : 1
'''G = NeuronGroup(N, eqs, threshold='v>1', reset='v=0', refractory=5*ms, method='euler')
M = SpikeMonitor(G)G.v0 = 'i*v0_max/(N-1)'run(duration)figure(figsize=(12,4))
subplot(121)
plot(M.t/ms, M.i, '.k')
xlabel('Time (ms)')
ylabel('Neuron index')
subplot(122)
plot(G.v0, M.count/duration)
xlabel('v0')
ylabel('Firing rate (sp/s)');
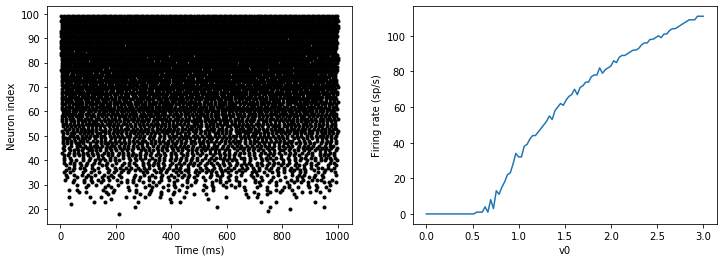
That’s the same figure as in the previous section but with some noise added. Note how the curve has changed shape: instead of a sharp jump from firing at rate 0 to firing at a positive rate, it now increases in a sigmoidal fashion. This is because no matter how small the driving force the randomness may cause it to fire a spike.
End of tutorial
That’s the end of this part of the tutorial. The cell below has another example. See if you can work out what it is doing and why. Try adding a StateMonitor
to record the values of the variables for one of the neurons to help you understand it.
You could also try out the things you’ve learned in this cell.
Once you’re done with that you can move on to the next tutorial on Synapses.
start_scope()N = 1000
tau = 10*ms
vr = -70*mV
vt0 = -50*mV
delta_vt0 = 5*mV
tau_t = 100*ms
sigma = 0.5*(vt0-vr)
v_drive = 2*(vt0-vr)
duration = 100*mseqs = '''
dv/dt = (v_drive+vr-v)/tau + sigma*xi*tau**-0.5 : volt
dvt/dt = (vt0-vt)/tau_t : volt
'''reset = '''
v = vr
vt += delta_vt0
'''G = NeuronGroup(N, eqs, threshold='v>vt', reset=reset, refractory=5*ms, method='euler')
spikemon = SpikeMonitor(G)G.v = 'rand()*(vt0-vr)+vr'
G.vt = vt0run(duration)_ = hist(spikemon.t/ms, 100, histtype='stepfilled', facecolor='k', weights=ones(len(spikemon))/(N*defaultclock.dt))
xlabel('Time (ms)')
ylabel('Instantaneous firing rate (sp/s)');
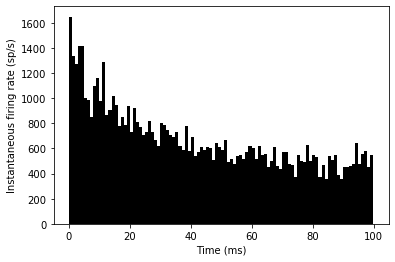
这篇关于Brian2 学习笔记(1)-Introduction to Brian part 1: Neurons的文章就介绍到这儿,希望我们推荐的文章对编程师们有所帮助!