本文主要是介绍GEE:指数计算(NDVI、NBR、EVI、NDMI、NDSI、TC、NDFI、EBBI、VCI、BSI、NDBI、GRAY),希望对大家解决编程问题提供一定的参考价值,需要的开发者们随着小编来一起学习吧!
本文记录了,使用 Landsat 光学波段计算指数的方法和代码,包括NDVI、NBR、EVI、NDMI、NDSI、TC变化(绿度、亮度、湿度)、NDFI、EBBI、VCI等指数。
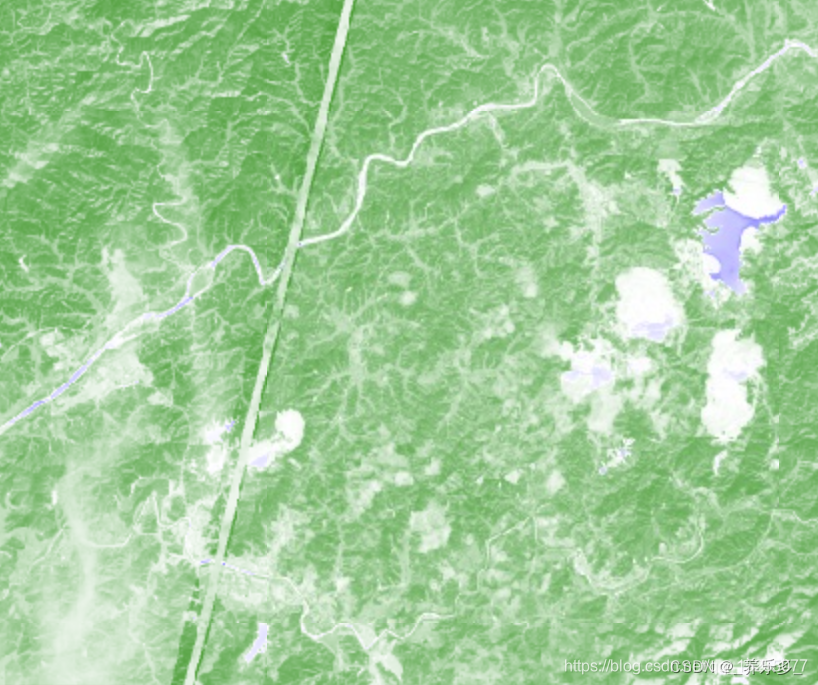
文章目录
- 一、代码框架
- 二、指数计算
- 1.NDVI
- 2.NBR
- 3.EVI
- 4.NDMI
- 5.NDSI
- 6.TC-Transform
- 7.NDFI
- 8.EBBI
- 9.VCI
- 10.BSI
- 11.NDBI
- 12.GRAY
一、代码框架
在GEE平台上使用Landsat影像计算指数需要以下几个步骤:
- 导入Landsat影像;
- 定义指数计算函数;
- 计算指数并将其添加到图层中;
- 将图层添加到地图中。
下面是一个简单的示例代码,演示如何使用GEE平台计算归一化植被指数(NDVI):
//导入研究区边界
var roi = table
Map.centerObject(roi, 10);
Map.addLayer(roi, {color:"black"}, "roi");// Step 1: 导入Landsat影像
var l8 = ee.ImageCollection('LANDSAT/LC08/C01/T1_TOA').filterDate('2019-01-01', '2019-12-31').filterBounds(roi).map(roiClip);//按研究区边界裁剪
function roiClip(image){return image.clip(roi)
}// Step 2: 定义NDVI计算函数
function addNDVI(image) {var ndvi = image.normalizedDifference(['B5', 'B4']).rename('NDVI');return image.addBands(ndvi);
}// Step 3: 计算NDVI并将其添加到图层中
var withNDVI = l8.map(addNDVI);// Step 4: 将图层添加到地图中
// 可视化参数
var viz = {min:-1, max:1, palette:'blue, white, green'};
Map.addLayer(withNDVI.select('NDVI'), viz, 'NDVI');
二、指数计算
以下指数计算中使用到的波段名,基于《GEE:时间序列分析2——将Landsat5、7、8所有影像合成一个影像集合,构建NDVI时间序列》一文中Landsat578波段统一后的名称。
1.NDVI
归一化植被指数
// NDVI
var ndviTransform = function(img){ var ndvi = img.normalizedDifference(['B4', 'B3']) // calculate normalized dif between band 4 and band 3 (B4-B3/B4_B3).multiply(1000) // scale results by 1000.select([0], ['NDVI']) // name the band.set('system:time_start', img.get('system:time_start'));return ndvi;
};
2.NBR
// NBR
var nbrTransform = function(img) {var nbr = img.normalizedDifference(['B4', 'B7']) // calculate normalized difference of B4 and B7. orig was flipped: ['B7', 'B4'].multiply(1000) // scale results by 1000.select([0], ['NBR']) // name the band.set('system:time_start', img.get('system:time_start'));return nbr;
};
3.EVI
// EVI
var eviTransform = function(img) {var evi = img.expression('2.5 * ((NIR - RED) / (NIR + 6 * RED - 7.5 * BLUE + 1))', {'NIR': img.select('B4'),'RED': img.select('B3'),'BLUE': img.select('B1')}).multiply(1000) // scale results by 1000.select([0], ['EVI']) // name the band.set('system:time_start', img.get('system:time_start')); return evi;
};
4.NDMI
// NDMI
var ndmiTransform = function(img) {var ndmi = img.normalizedDifference(['B4', 'B5']) // calculate normalized difference of B4 and B7. orig was flipped: ['B7', 'B4'].multiply(1000) // scale results by 1000.select([0], ['NDMI']) // name the band.set('system:time_start', img.get('system:time_start'));return ndmi;
};
5.NDSI
// NDSI
var ndsiTransform = function(img){ var ndsi = img.normalizedDifference(['B2', 'B5']) // calculate normalized dif between band 4 and band 3 (B4-B3/B4_B3).multiply(1000) // scale results by 1000.select([0], ['NDSI']) // name the band.set('system:time_start', img.get('system:time_start'));return ndsi;
};
6.TC-Transform
// TASSELLED CAP
var tcTransform = function(img){ var b = ee.Image(img).select(["B1", "B2", "B3", "B4", "B5", "B7"]); // select the image bandsvar brt_coeffs = ee.Image.constant([0.2043, 0.4158, 0.5524, 0.5741, 0.3124, 0.2303]); // set brt coeffs - make an image object from a list of values - each of list element represents a bandvar grn_coeffs = ee.Image.constant([-0.1603, -0.2819, -0.4934, 0.7940, -0.0002, -0.1446]); // set grn coeffs - make an image object from a list of values - each of list element represents a bandvar wet_coeffs = ee.Image.constant([0.0315, 0.2021, 0.3102, 0.1594, -0.6806, -0.6109]); // set wet coeffs - make an image object from a list of values - each of list element represents a bandvar sum = ee.Reducer.sum(); // create a sum reducer to be applyed in the next steps of summing the TC-coef-weighted bandsvar brightness = b.multiply(brt_coeffs).reduce(sum); // multiply the image bands by the brt coef and then sum the bandsvar greenness = b.multiply(grn_coeffs).reduce(sum); // multiply the image bands by the grn coef and then sum the bandsvar wetness = b.multiply(wet_coeffs).reduce(sum); // multiply the image bands by the wet coef and then sum the bandsvar angle = (greenness.divide(brightness)).atan().multiply(180/Math.PI).multiply(100);var tc = brightness.addBands(greenness).addBands(wetness).addBands(angle).select([0,1,2,3], ['TCB','TCG','TCW','TCA']) //stack TCG and TCW behind TCB with .addBands, use select() to name the bands.set('system:time_start', img.get('system:time_start'));return tc;
};indexImg = tc.select(['TCB']);
indexImg = tc.select(['TCG']);
indexImg = tc.select(['TCW']);
indexImg = tc.select(['TCA']);
7.NDFI
//Ben added
// NDFI - from CODED utility (original: users/bullocke/coded:coded/miscUtilities)
var ndfiTransform = function(img) {// pre-defined endmembersvar params = ee.Dictionary({'cfThreshold': 0.01, // CLOUD THRESHOLD 'soil': [2000, 3000, 3400, 5800, 6000, 5800],'gv': [500, 900, 400, 6100, 3000, 1000],'npv': [1400, 1700, 2200, 3000, 5500, 3000],'shade': [0, 0, 0, 0, 0, 0],'cloud': [9000, 9600, 8000, 7800, 7200, 6500]});/* Utility function for calculating spectral indices */var gv = params.get('gv');var shade = params.get('shade');var npv = params.get('npv');var soil = params.get('soil');var cloud = params.get('cloud');//var cfThreshold = ee.Image.constant(params.get('cfThreshold'))/* Do spectral unmixing on a single image */var unmixImage = ee.Image(img).unmix([gv, shade, npv, soil, cloud], true,true).rename(['band_0', 'band_1', 'band_2','band_3','band_4']);var newImage = ee.Image(img).addBands(unmixImage);//var mask = newImage.select('band_4').lt(cfThreshold)var ndfi = unmixImage.expression('((GV / (1 - SHADE)) - (NPV + SOIL)) / ((GV / (1 - SHADE)) + NPV + SOIL)', {'GV': unmixImage.select('band_0'),'SHADE': unmixImage.select('band_1'),'NPV': unmixImage.select('band_2'),'SOIL': unmixImage.select('band_3')}); var ndvi = ee.Image(img).normalizedDifference(['B4','B3']).rename('NDVI')var evi = ee.Image(img).expression('float(2.5*(((B4/10000) - (B3/10000)) / ((B4/10000) + (6 * (B3/10000)) - (7.5 * (B1/10000)) + 1)))',{'B4': ee.Image(img).select(['B4']),'B3': ee.Image(img).select(['B3']),'B1': ee.Image(img).select(['B1'])}).rename('EVI'); var toExp = newImage.addBands([ndfi.rename(['NDFI']), ndvi, evi]).select(['band_0','band_1','band_2','band_3','NDFI','NDVI','EVI','B1','B2','B3','B4','B5']).rename(['GV','Shade','NPV','Soil','NDFI','NDVI','EVI','Blue','Green','Red','NIR','SWIR1']); //.updateMask(mask)toExp = toExp.select(['NDFI']).multiply(1000).set('system:time_start', img.get('system:time_start'));return toExp;};
8.EBBI
// calculate EBBI
var ebbi = median1.expression('(SWIR - NIR)/ 10 * sqrt(SWIR + TIRS)',
{
'SWIR':median1.select('B5_median'),
'NIR':median1.select('B4_median'),
'TIRS' : median1.select('B6_median')
}).rename('EBBI');
9.VCI
计算多年来的植被状况指数(the vegetation condition index,VCI)
(代码链接)
var getvci = function(image){// ((NDVI-NDVImin)/(NDVImax-MDVImin))*100var vci = image.subtract(minImage).divide(maxImage.subtract(minImage)).rename('VCI')// return image.addBands(vci) // both output togtherreturn vci // only the vci
}
10.BSI
var addBSI = function(image) {var bsi = image.expression('((RED + SWIR) - (NIR + BLUE)) / ((RED + SWIR) + (NIR + BLUE)) ', {'RED': image.select('B4'), 'BLUE': image.select('B2'),'NIR': image.select('B8'),'SWIR': image.select('B11'),}
).rename('BSI').copyProperties(image,['system:time_start']);return image.addBands(bsi);
};
11.NDBI
归一化建筑指数:RNIR、RMIR分别为影像的近红外、中红外波段,OLI数据的5波段、6波段。
// NDBI
var ndbiTransform = function(img) {var ndbi = img.normalizedDifference(['B5', 'B6']) // calculate normalized difference of B5 and B6. orig was flipped: ['B5', 'B6'].multiply(1000) // scale results by 1000.select([0], ['NDBI']) // name the band.set('system:time_start', img.get('system:time_start'));return ndbi;
};
12.GRAY
var GRAY = imageOriginal.expression('(0.3 * NIR) + (0.59 * R) + (0.11 * G)', {'NIR': imageOriginal.select('B5'),'R': imageOriginal.select('B4'),'G': imageOriginal.select('B3')}).multiply(1000).rename('GRAY');
这篇关于GEE:指数计算(NDVI、NBR、EVI、NDMI、NDSI、TC、NDFI、EBBI、VCI、BSI、NDBI、GRAY)的文章就介绍到这儿,希望我们推荐的文章对编程师们有所帮助!