本文主要是介绍python 四分卫数_NFL 2020预览与Python四分卫,希望对大家解决编程问题提供一定的参考价值,需要的开发者们随着小编来一起学习吧!
python 四分卫数
NFL 2020 season is coming soon. For preview this season, I’m going to visualize some quarterbacks data using 2019 dataset.
NFL 2020赛季即将到来。 为了预览本季,我将使用2019年数据集可视化一些四分卫数据。
1.概述 (1. Overview)
In this article, I’m going to use this dataset as below. Thanks to Mr. Ron Yurko.
在本文中,我将使用以下数据集。 感谢Ron Yurko先生。
There is play-by-play dataset of pre-season, regular season and play-off. I’m going to use only regular season and visualize some quarterback stats. What kind of type? Pocket passer or Mobile QB? How is their performance? How is it when they are in the specific situation such as quarter, down and score behind?
有季前,常规赛和附加赛的逐项比赛数据集。 我将只使用常规赛季并可视化一些四分卫的数据。 什么样的类型? 口袋路人还是手机QB? 他们的表现如何? 当他们处在特定情况下(如四分之一,下降,得分落后)时,情况如何?
OK, Let’s get down to implementation.
好的,让我们开始实施。
2.预处理 (2. Preprocessing)
import pandas as pd
pd.set_option(“max_columns”, 400)
pbp = pd.read_csv(“play_by_play_data/regular_season/reg_pbp_2019.csv”)
roster = pd.read_csv(“roster_data/regular_season/reg_roster_2019.csv”)
Filter with quarterbacks.
用四分卫过滤。
qb = roster[roster.position == “QB”].sort_values(“full_player_name”).reset_index(drop=True)
See the dataframe info of pbp dataset.
查看pbp数据集的数据框信息。
pbp.info()
<class ‘pandas.core.frame.DataFrame’> RangeIndex: 45546 entries, 0 to 45545 Columns: 256 entries, play_id to defensive_extra_point_conv dtypes: float64(130), int64(21), object(105) memory usage: 89.0+ MB
<class'pandas.core.frame.DataFrame'> RangeIndex:45546个条目,0到45545列:256个条目,play_id到defensive_extra_point_conv dtypes:float64(130),int64(21),object(105)内存使用量:89.0+ MB
It’s too large to visualize quarterback data, so narrow down.
它太大而无法可视化四分卫数据,因此请缩小范围。
pbp_custom = pbp[[
“game_id”
,”game_half”
,”qtr”
,”time”
,”posteam”
,”yardline_100"
,”down”
,”ydstogo”
,”two_point_attempt”
,”yards_gained”
,”air_yards”
,”yards_after_catch”
,”play_type”
,”first_down_pass”
,”first_down_rush”
,”qb_hit”
,”rush_attempt”
,”pass_attempt”
,”complete_pass”
,”incomplete_pass”
,”sack”
,”touchdown”
,”interception”
,”pass_touchdown”
,”rush_touchdown”
,”pass_length”
,”pass_location”
,”passer_player_id”
,”passer_player_name”
,”rusher_player_id”
,”rusher_player_name”
]].sort_values(
[
“game_id”
,”game_half”
,”qtr”
,”time”
]
,ascending=[
True
,True
,True
,False
]
)
Aggregate this data as passing stats.
将此数据汇总为通过状态。
#Don’t count sack yards for player’s stats
pbp_custom.loc[pbp_custom.sack == 1, “yards_gained”] = 0#Aggregate by player, quarter and down
qb_pass_stats = pbp_custom[
(pbp_custom.passer_player_id.isin(qb.gsis_id)) #only QB
& (pbp_custom.two_point_attempt == 0) #exclude two-point conversion
].groupby(
[
“passer_player_id”
,”qtr”
,”down”
]
,as_index=False
).agg(
{
“complete_pass”: “sum”
,”yards_gained”: “sum”
,”first_down_pass”: “sum”
,”pass_touchdown”: “sum”
,”incomplete_pass”: “sum”
,”sack”: “sum”
,”interception”: “sum”
}
)#Create new columns
qb_pass_stats[“pass_attempt”] = qb_pass_stats[“complete_pass”] + qb_pass_stats[“incomplete_pass”] + qb_pass_stats[“interception”]
qb_pass_stats[“complete_rate”] = round(
qb_pass_stats[“complete_pass”] / qb_pass_stats[“pass_attempt”]
, 3
) * 100#Aggregate by player
qb_pass_stats_season = qb_pass_stats.groupby(
[“passer_player_id”]
,as_index=False
).agg(
{
“pass_attempt”: “sum”
,“complete_pass”: “sum”
,”yards_gained”: “sum”
,”first_down_pass”: “sum”
,”pass_touchdown”: “sum”
,”incomplete_pass”: “sum”
,”sack”: “sum”
,”interception”: “sum”
}
)#Create new columns
qb_pass_stats_season[“complete_rate”] = round(
qb_pass_stats_season[“complete_pass”] / qb_pass_stats_season[“pass_attempt”]
, 3
) * 100#only who exceed 2000 yards
qb_pass_stats_season = qb_pass_stats_season[qb_pass_stats_season.yards_gained >= 2000]
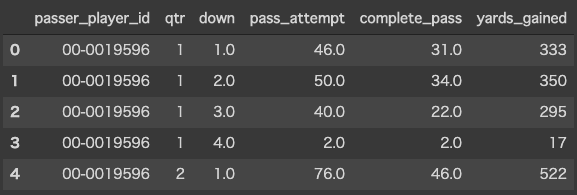
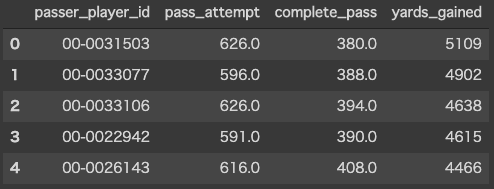
Top is Jameis Winston with 5109 yards.
最高的是5109码的Jameis Winston。
Do the same with rushing. “yards_gained” doesn’t include lateral rush, please note that.
匆匆做同样的事情。 “ yards_gained”不包括横向奔波,请注意。
#Aggregate by player, quarter and down
qb_rush_stats = pbp_custom[
pbp_custom.rusher_player_id.isin(
qb_pass_stats_season.passer_player_id
)].groupby(
[
“rusher_player_id”
,”qtr”
,”down”
]
,as_index=False
).agg(
{
“play_type”: “count”
,”yards_gained”: “sum”
,”first_down_rush”: “sum”
,”rush_touchdown”: “sum”
}
)#Aggregate by player
qb_rush_stats_season = qb_rush_stats.groupby(
[
“rusher_player_id”
]
,as_index=False
).agg(
{
“rush_attempt”: “sum”
,”yards_gained”: “sum”
,”first_down_rush”: “sum”
,”rush_touchdown”: “sum”
}
)
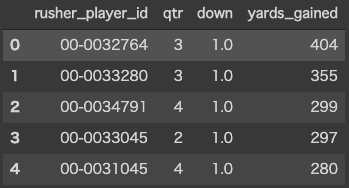
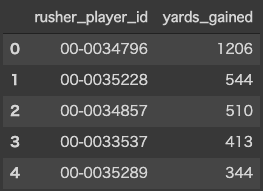
Top is of cource Lamar Jackson with 1206 yards.
顶部是库拉(Lamar Jackson)的1206码码。
Merge passing dataset and rushing dataset, also merge player dataset.
合并通过数据集和紧急数据集,也合并玩家数据集。
#Merge pass stats and rush stats datasets
qb_stats_season = pd.merge(
qb_pass_stats_season
,qb_rush_stats_season
,left_on=”passer_player_id”
,right_on=”rusher_player_id”
,how=”inner”
,suffixes=[“_passing”, “_rushing”]
).sort_values(“yards_gained_passing”, ascending=False)#Merge stats and players datasets
qb_stats_season = pd.merge(
qb_stats_season
,qb
,left_on="passer_player_id"
,right_on="gsis_id"
,how="inner"
)qb_stats_season = qb_stats_season.rename(columns={"passer_player_id": "player_id"})#Create new columns
qb_stats_season["yards_gained"] = qb_stats_season["yards_gained_passing"] + qb_stats_season["yards_gained_rushing"]qb_stats_season["touchdown"] = qb_stats_season["pass_touchdown"] + qb_stats_season["rush_touchdown"]
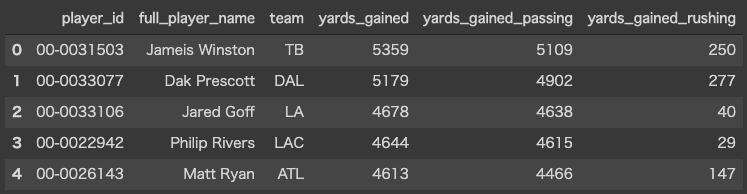
3.可视化 (3. Visualization)
Let’s visualize quarterback playing style. Describe passing yards and rushing yards using scatter plot.
让我们可视化四分卫的比赛风格。 使用散点图描述通过码和冲码。
%matplotlib inline
import matplotlib.pyplot as pltwith plt.rc_context(
{
"axes.edgecolor":"white"
,"xtick.color":"white"
, "ytick.color":"white"
, "figure.facecolor":"white"
}
):
fig = plt.figure(figsize=(15, 12), facecolor="black")
ax = fig.add_subplot(111, facecolor="black")#Plot scatter
s = ax.scatter(
qb_stats_season["yards_gained_passing"]
,qb_stats_season["yards_gained_rushing"]
,s=200
,alpha=0.5
,c=(qb_stats_season["sack"] + qb_stats_season["interception"])
,cmap="bwr"
,marker="D"
)
ax.set_xlabel("Pass Yds", color="white")
ax.set_ylabel("Rush Yds", color="white")
ax.set_xlim(2400, 5200)
ax.set_ylim(-100, 1300)#Plot player name as text
for _, qb_data in qb_stats_season.iterrows():
ax.text(
qb_data.yards_gained_passing
,qb_data.yards_gained_rushing
,qb_data.full_player_name
,verticalalignment="center"
,horizontalalignment="center"
,fontsize=13
,color="white"
)#Colorbar settings
cb = plt.colorbar(s)
cb.set_label("Sack + Interception", color="white", size=20)
cb.outline.set_edgecolor("white")
plt.setp(plt.getp(cb.ax.axes, 'yticklabels'), color="white")plt.title("QB Type", color="white")
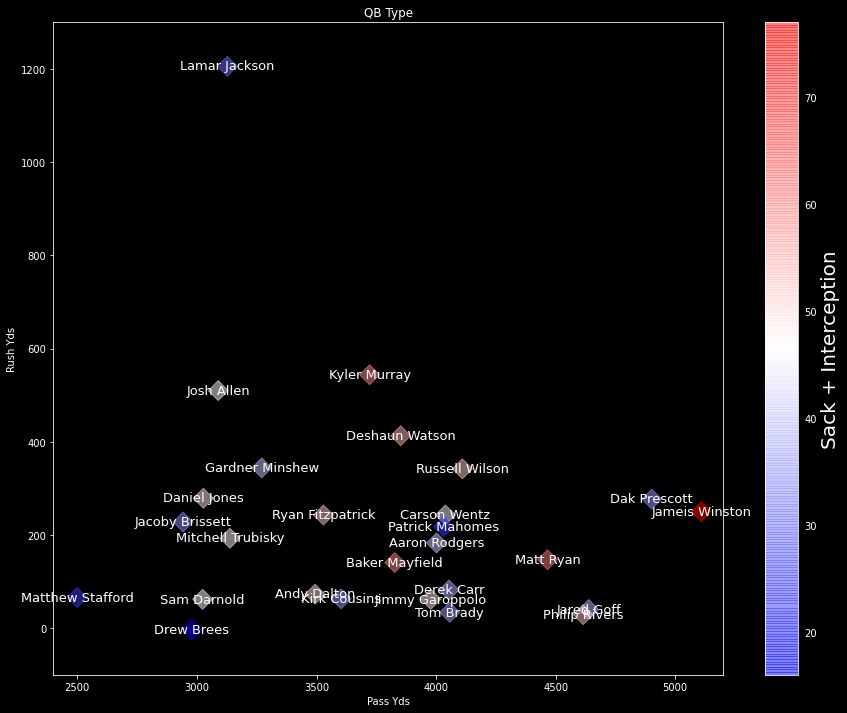
X-axis is passing yards and Y-axis is rushing yards. It’s strange to be defined different scale between x-axis and y-axis, but this is for visibility.
X轴是经过码,Y轴是冲码。 在x轴和y轴之间定义不同的比例很奇怪,但这是为了提高可见性。
I also colored each marker, which is total amount of sack and interception. Red, such as Winston and Murray, is more sacked and intercepted while blue, such as Mahomes and Brees, is less sacked and intercepted.
我还为每个标记着色,这是麻袋和拦截物的总量。 红色(例如Winston和Murray)被解雇和被拦截,而蓝色(例如Mahomes和Brees)被解雇和被拦截。
We can find out:
我们可以找到:
- Winston has the highest passing yards but was more sacked and intercepted. 温斯顿传球码最高,但被解雇和拦截的次数更多。
- Jackson is absolutely mobile QB and was also less sacked and intercepted. 杰克逊绝对是行动QB,也没有那么被解雇和被拦截。
- Mahomes and Brees was much less sacked and intercepted but not many passing yards. Mahomes和Brees被解雇和拦截的次数要少得多,但传球码并不多。
- Murray, Watson and Wilson is good at both? 默里,沃森和威尔逊都擅长吗?
Next, how many yards they gained while they were sacked or intercepted?
接下来,他们被解雇或拦截时获得了多少码?
Calculate yards gained per sacked and intercepted and visualize it using histogram.
计算每个被解雇和拦截的码数,并使用直方图将其可视化。
#Create new column
qb_stats_season[“gained_per_sack_and_interception”] = round(
qb_stats_season[“yards_gained”] / (qb_stats_season[“sack”] + qb_stats_season[“interception”])
,1
)qb_stats_season = qb_stats_season.sort_values(“gained_per_sack_and_interception”, ascending=True).reset_index(drop=True)with plt.rc_context(
{
"axes.edgecolor":"white"
,"xtick.color":"white"
, "ytick.color":"white"
, "figure.facecolor":"white"
}
):
fig = plt.figure(figsize=(10, 10), facecolor=”black”)
ax = fig.add_subplot(111, facecolor=”black”)#Plot horizontal histogram
ax.barh(
qb_stats_season.full_player_name
,qb_stats_season.gained_per_sack_and_interception
,color=”grey”
)#Plot stats as text on histogram
for index, qb_data in qb_stats_season.iterrows():
ax.text(
qb_data.gained_per_sack_and_interception
,index
,str(qb_data.yards_gained) + “ / “ + str(int(qb_data.sack) + int(qb_data.interception))
,color=”white”
,ha=”center”
,va=”right”
)
plt.title(“Never Fail QB Ranks”, color=”white”)
ax.set_xlabel(“Gained / (Sack + Interception)”, color=”white”)
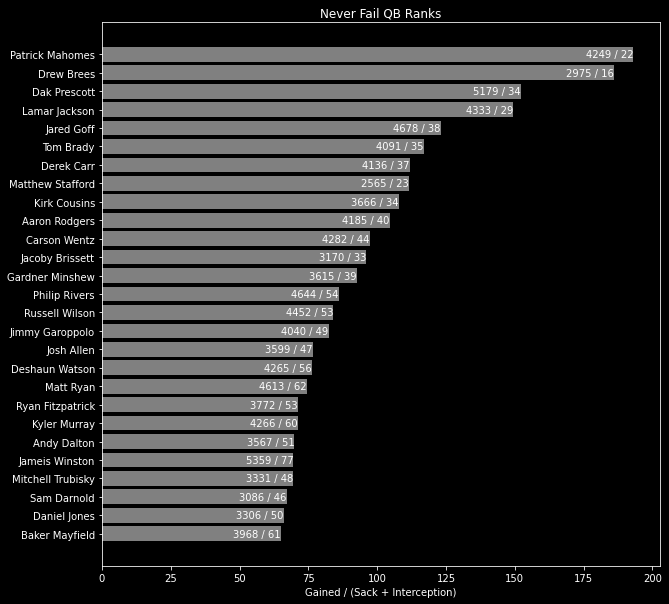
How stable Mahomes is. Brees, Prescott and Jackson are also outstanding. Meanwhile, Winston and Murray has many yards but we can say they are not stable.
Mahomes有多稳定。 布雷斯,普雷斯科特和杰克逊也很出色。 同时,温斯顿(Winston)和穆雷(Murray)有很多码,但是我们可以说它们不稳定。
By the way, how about each quarter? Aggregate data again.
顺便问一下,每个季度怎么样? 再次汇总数据。
qb_pass_stats_qtr = qb_pass_stats.groupby(
[
“passer_player_id”
,”qtr”
]
,as_index=False
).agg(
{
“complete_pass”: “sum”
,”yards_gained”: “sum”
,”first_down_pass”: “sum”
,”pass_touchdown”: “sum”
,”incomplete_pass”: “sum”
,”sack”: “sum”
,”interception”: “sum”
}
)
qb_pass_stats_qtr[“pass_attempt”] = qb_pass_stats_qtr[“complete_pass”] + qb_pass_stats_qtr[“incomplete_pass”] + qb_pass_stats_qtr[“interception”]qb_pass_stats_qtr[“complete_rate”] = round(qb_pass_stats_qtr[“complete_pass”] / qb_pass_stats_qtr[“pass_attempt”], 3) * 100qb_rush_stats_qtr = qb_rush_stats.groupby(
[
"rusher_player_id"
,"qtr"
]
,as_index=False
).agg(
{
"rush_attempt": "sum"
,"yards_gained": "sum"
,"first_down_rush": "sum"
,"rush_touchdown": "sum"
}
)qb_stats_qtr = pd.merge(
qb_pass_stats_qtr
,qb_rush_stats_qtr
,left_on=["passer_player_id","qtr"]
,right_on=["rusher_player_id","qtr"]
,how="inner"
,suffixes=["_passing", "_rushing"]
)qb_stats_qtr = pd.merge(
qb_stats_qtr
,qb
,left_on="passer_player_id"
,right_on="gsis_id"
,how="inner"
)qb_stats_qtr["yards_gained"] = qb_stats_qtr["yards_gained_passing"] + qb_stats_qtr["yards_gained_rushing"]qb_stats_qtr["touchdown"] = qb_stats_qtr["pass_touchdown"] + qb_stats_qtr["rush_touchdown"]qb_stats_qtr = qb_stats_qtr.rename(columns={"passer_player_id": "player_id"})
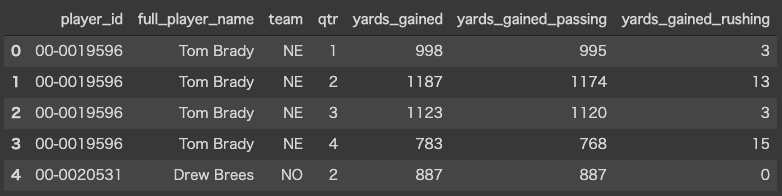
qb_stats_4q = qb_stats_qtr[qb_stats_qtr.qtr == 4].sort_values(“yards_gained”, ascending=False)with plt.rc_context(
{
"axes.edgecolor":"white"
,"xtick.color":"white"
, "ytick.color":"white"
, "figure.facecolor":"white"
}
):
fig = plt.figure(figsize=(15, 5), facecolor=”black”)
ax = fig.add_subplot(111, facecolor=”black”)s = ax.scatter(
qb_stats_4q.yards_gained_passing
,qb_stats_4q.yards_gained_rushing
,s=200
,alpha=0.5
,c=(qb_stats_4q.sack + qb_stats_4q.interception)
,cmap=”bwr”
,marker=”D”
)ax.set_xlabel(“Pass Yds”, color=”white”)
ax.set_ylabel(“Rush Yds”, color=”white”)for _, qb_data in qb_stats_4q.iterrows():
ax.text(
qb_data.yards_gained_passing
,qb_data.yards_gained_rushing
,qb_data.full_player_name
,verticalalignment=”center”
,horizontalalignment=”center”
,fontsize=13
,color=”white”
)cb = plt.colorbar(s)
cb.set_label(“Sack + Interception”, color=”white”, size=20)
cb.outline.set_edgecolor(“white”)
plt.setp(plt.getp(cb.ax.axes, ‘yticklabels’), color=”white”)
plt.title(“QB Type in 4Q”, color=”white”)
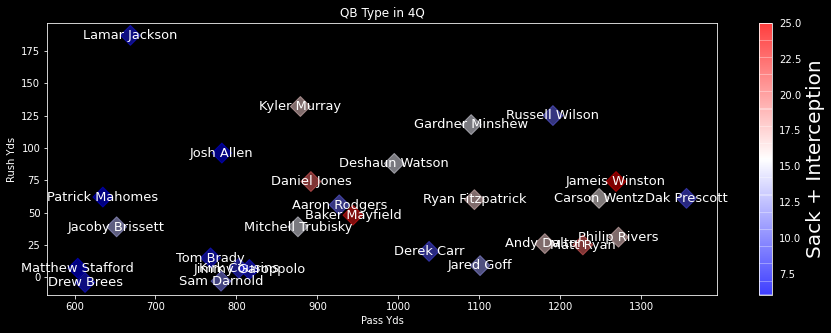
Prescott and Mahomes are in constrast. Compare the gained yards in each quarter. We can also say that most QBs are less sacked and intercepted because of 4Q. (Winston and Mayfield are gambler?)
普雷斯科特(Prescott)和马荷姆斯(Mahomes)持反对意见。 比较每个季度获得的码数。 我们也可以说,由于Q,大多数QB的解雇和拦截较少。 (温斯顿和梅菲尔德是赌徒?)
mahomes_stats_qtr = qb_stats_qtr[qb_stats_qtr.player_id == “00–0033873”]
prescott_stats_qtr = qb_stats_qtr[qb_stats_qtr.player_id == “00–0033077”]with plt.rc_context(
{
"axes.edgecolor":"white"
,"xtick.color":"white"
, "ytick.color":"white"
, "figure.facecolor":"white"
}
):
fig = plt.figure(figsize=(10, 5), facecolor=”black”)
ax_mahomes = fig.add_subplot(121, facecolor=”black”)
ax_prescott = fig.add_subplot(122, facecolor=”black”)#Draw pie chart of Mahomes
wedges, _, _ = ax_mahomes.pie(
mahomes_stats_qtr.yards_gained
,labels=[“1Q”,”2Q”,”3Q”,”4Q”]
,textprops={“color”: “white”}
,wedgeprops={“linewidth”: 3}
,startangle=90
,counterclock=False
,autopct=”%1.1f%%”
)
ax_mahomes.text(
0, 0
,qb_stats_season[“yards_gained”][qb_stats_season.player_id == “00–0033873”].values[0]
,color=”white”
,ha=”center”
,va=”center”
,fontsize=20
)
plt.setp(wedges, width=0.2)#Draw pie chart of Prescott
wedges, _, _ = ax_prescott.pie(
prescott_stats_qtr.yards_gained
,labels=[“1Q”,”2Q”,”3Q”,”4Q”]
,textprops={“color”: “white”}
,wedgeprops={“linewidth”: 3}
,startangle=90
,counterclock=False
,autopct=”%1.1f%%”ax_prescott.text(
0, 0
,qb_stats_season[“yards_gained”][qb_stats_season.player_id == “00–0033077”].values[0]
,color=”white”
,ha=”center”
,va=”center”
,fontsize=20
)
plt.setp(wedges, width=0.2)ax_mahomes.set_title(“Mahomes”, color=”white”)
ax_prescott.set_title(“Prescott”, color=”white”)
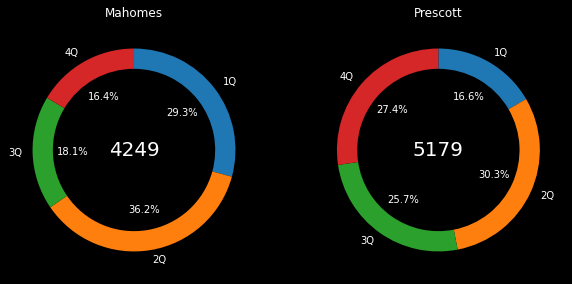
Can we describe Mahomes is “pre-emptive” QB and Prescott is “rising” QB?
我们能否描述Mahomes是“先发制人”的QB而Prescott是“崛起”的QB?
In addition, how about when the team is in adversity (score behind)?
此外,团队何时处于逆境中(得分落后)?
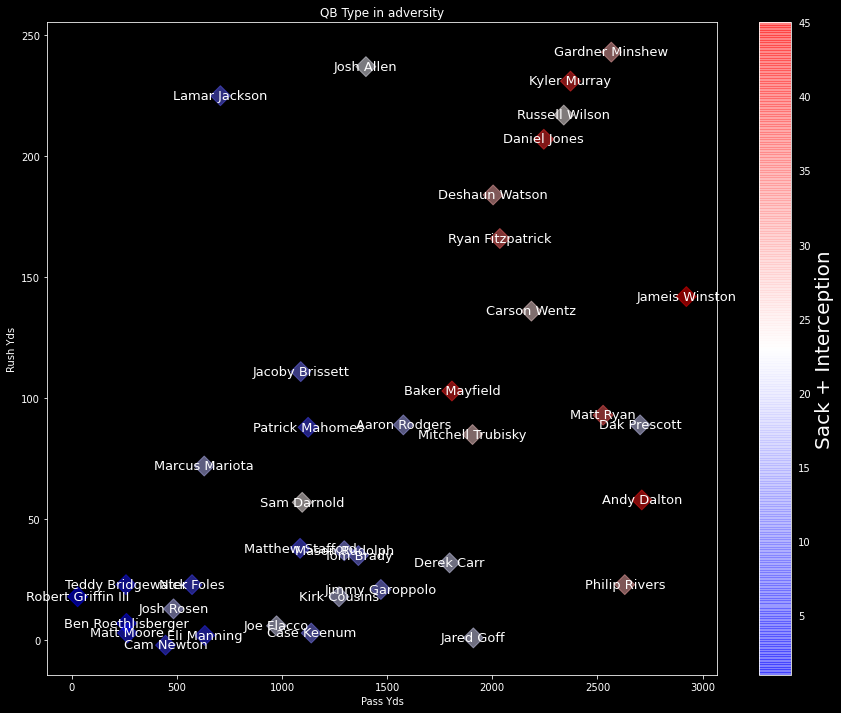
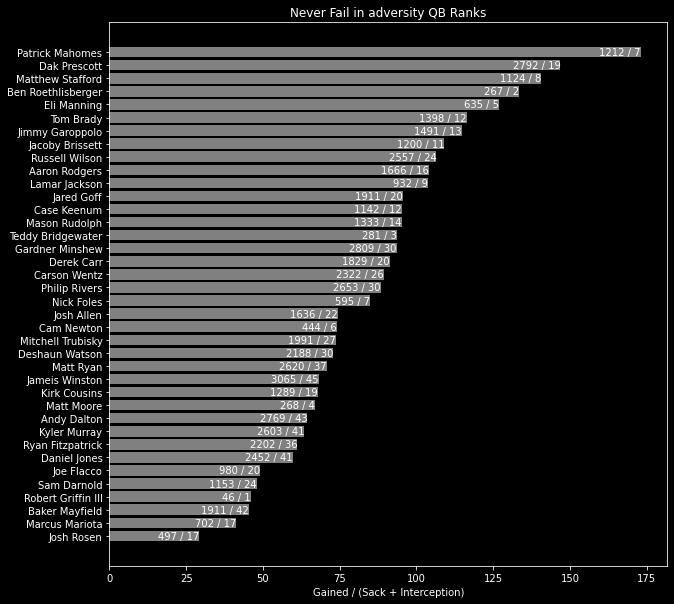
Oh, Mahomes is also outstanding in adversity… Prescott is too. Stafford is 3rd while he is 8th in gross and Garoppolo is 7th while 16th in gross. We can say they are strong in adversity.
哦,Mahomes在逆境中也很出色... Prescott也是。 斯塔福德排名第3,而他排名第8,加洛波罗排名第7,而排名第16。 我们可以说他们在逆境中很强。
I can do as much as I want, but leave off around here. Will Mahomes be MVP again with outstanding stability? Prescott will lead Dallas to Superbowl? How will Winston achieve at Saints alongside Brees? Can Murray and Mayfield improve stability and become the best QB in NFL?
我可以做很多我想做的事,但是不要在这里闲逛。 Mahomes会再次以出色的稳定性成为MVP吗? 普雷斯科特会带领达拉斯进入超级碗吗? 温斯顿将如何与布雷斯一起在圣徒队取得成就? Murray和Mayfield能否提高稳定性并成为NFL中最好的QB?
Thank you for reading!!
谢谢您的阅读!!
翻译自: https://medium.com/the-sports-scientist/nfl-2020-preview-with-python-quarterback-24345b76b97a
python 四分卫数
相关文章:
这篇关于python 四分卫数_NFL 2020预览与Python四分卫的文章就介绍到这儿,希望我们推荐的文章对编程师们有所帮助!