本文主要是介绍IJCAI2023推荐系统相关论文集锦,希望对大家解决编程问题提供一定的参考价值,需要的开发者们随着小编来一起学习吧!
第32届国际人工智能联合会议(International Joint Conference on Artificial Intelligence, 简称为IJCAI)是人工智能领域最顶级的国际学术会议之一,也是CCF-A类会议。今年的IJCAI将于2023年8月19-25日在中国澳门特别行政区举办。在今年的4566篇投稿论文中,有大约15%的论文被接收,其中跟推荐系统相关的论文大约16篇。通过对今年的论文题目进行分析发现,对于图数据的挖掘仍然是主流,所涉及的技术涵盖多模态、注意力、强化学习、联邦学习等技术。
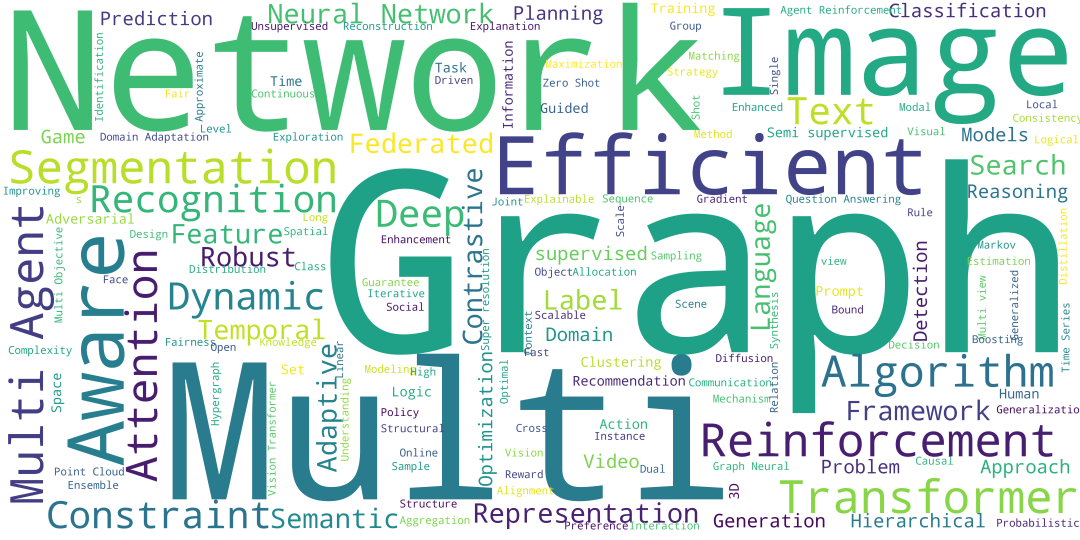
通过对16篇推荐系统相关论文进行总结,其中包含1篇用户行为建模综述类论文[1]。另外Main Track中11篇算法类论文则包含图推荐[2,6,11,12]、对话推荐[3]、序列推荐[4,7]、联邦推荐[5,8]、无偏推荐[10]等。更具体的内容可参考下文的部分摘要内容。
Survey Track
1. A Survey on User Behavior Modeling in Recommender Systems
Zhicheng He, Weiwen Liu, Wei Guo, Jiarui Qin, Yingxue Zhang, Yaochen Hu, Ruiming Tang
https://arxiv.org/abs/2302.11087
User Behavior Modeling (UBM) plays a critical role in user interest learning, which has been extensively used in recommender systems. Crucial interactive patterns between users and items have been exploited, which brings compelling improvements in many recommendation tasks. In this paper, we attempt to provide a thorough survey of this research topic. We start by reviewing the research background of UBM. Then, we provide a systematic taxonomy of existing UBM research works, which can be categorized into four different directions including Conventional UBM, Long-Sequence UBM, Multi-Type UBM, and UBM with Side Information. Within each direction, representative models and their strengths and weaknesses are comprehensively discussed. Besides, we elaborate on the industrial practices of UBM methods with the hope of providing insights into the application value of existing UBM solutions. Finally, we summarize the survey and discuss the future prospects of this field.
Main Track
2. Self-supervised Graph Disentangled Networks for Review-based Recommendation
Yuyang Ren, Haonan Zhang, Qi Li, Luoyi Fu, Xinbing Wang, Chenghu Zhou
https://arxiv.org/abs/2209.01524
User review data is considered as auxiliary information to alleviate the data sparsity problem and improve the quality of learned user/item or interaction representations in review-based recommender systems. However, existing methods usually model user-item interactions in a holistic manner and neglect the entanglement of the latent intents behind them, e.g., price, quality, or appearance, resulting in suboptimal representations and reducing interpretability. In this paper, we propose a Self-supervised Graph Disentangled Networks for review-based recommendation (SGDN), to separately model the user-item interactions based on the latent factors through the textual review data. To this end, we first model the distributions of interactions over latent factors from both semantic information in review data and structural information in user-item graph data, forming several factor graphs. Then a factorized message passing mechanism is designed to learn disentangled user/item and interaction representations on the factor graphs. Finally, we set an intent-aware contrastive learning task to alleviate the sparsity issue and encourage disentanglement through dynamically identifying positive and negative samples based on the learned intent distributions. Empirical results over five benchmark datasets validate the superiority of SGDN over the state-of-the-art methods and the interpretability of learned intent factors.
3. Towards Hierarchical Policy Learning for Conversational Recommendation with Hypergraph-based Reinforcement Learning
Sen Zhao, Wei Wei, Yifan Liu, Ziyang Wang, Wendi Li, Xian-Ling Mao, Shuai Zhu, Minghui Yang, Zujie Wen
https://arxiv.org/abs/2305.02575
Conversational recommendation systems (CRS) aim to timely and proactively acquire user dynamic preferred attributes through conversations for item recommendation. In each turn of CRS, there naturally have two decision-making processes with different roles that influence each other: 1) director, which is to select the follow-up option (i.e., ask or recommend) that is more effective for reducing the action space and acquiring user preferences; and 2) actor, which is to accordingly choose primitive actions (i.e., asked attribute or recommended item) to estimate the effectiveness of the director’s option. However, existing methods heavily rely on a unified decision-making module or heuristic rules, while neglecting to distinguish the roles of different decision procedures, as well as the mutual influences between them. To address this, we propose a novel Director-Actor Hierarchical Conversational Recommender (DAHCR), where the director selects the most effective option, followed by the actor accordingly choosing primitive actions that satisfy user preferences. Specifically, we develop a dynamic hypergraph to model user preferences and introduce an intrinsic motivation to train from weak supervision over the director. Finally, to alleviate the bad effect of model bias on the mutual influence between the director and actor, we model the director’s option by sampling from a categorical distribution. Extensive experiments demonstrate that DAHCR outperforms state-of-the-art methods.
4. Sequential Recommendation with Probabilistic Logical Reasoning
Huanhuan Yuan, Pengpeng Zhao, Xuefeng Xian, Guanfeng Liu, Yanchi Liu, Victor S. Sheng, Lei Zhao
https://arxiv.org/abs/2304.11383
Deep learning and symbolic learning are two frequently employed methods in Sequential Recommendation (SR). Recent neural-symbolic SR models demonstrate their potential to enable SR to be equipped with concurrent perception and cognition capacities. However, neural-symbolic SR remains a challenging problem due to open issues like representing users and items in logical reasoning. In this paper, we combine the Deep Neural Network (DNN) SR models with logical reasoning and propose a general framework named Sequential Recommendation with Probabilistic Logical Reasoning (short for SR-PLR). This framework allows SR-PLR to benefit from both similarity matching and logical reasoning by disentangling feature embedding and logic embedding in the DNN and probabilistic logic network. To better capture the uncertainty and evolution of user tastes, SR-PLR embeds users and items with a probabilistic method and conducts probabilistic logical reasoning on users' interaction patterns. Then the feature and logic representations learned from the DNN and logic network are concatenated to make the prediction. Finally, experiments on various sequential recommendation models demonstrate the effectiveness of the SR-PLR.
5. Federated Probabilistic Preference Distribution Modelling with Compactness Co-Clustering for Privacy-Preserving Multi-Domain Recommendation
Weiming Liu, Chaochao Chen, Xinting Liao, Mengling Hu, Jianwei Yin, Yanchao Tan, Longfei Zheng
With the development of modern internet techniques, Cross-Domain Recommendation (CDR) systems have been widely exploited for tackling the data-sparsity problem. Meanwhile most current CDR models assume that user-item interactions are accessible across different domains. However, such knowledge sharing process will break the privacy protection policy. In this paper, we focus on the Privacy-Preserving Multi-Domain Recommendation problem (PPMDR). The problem is challenging since different domains are sparse and heterogeneous with the privacy protection. To tackle the above issues, we propose Federated Probabilistic Preference Distribution Modelling (FPPDM). FPPDM includes two main components, i.e., local domain modelling component and global server aggregation component with federated learning strategy. The local domain modelling component aims to exploit user/item preference distributions using the rating information in the corresponding domain. The global server aggregation component is set to combine user characteristics across domains. To better extract semantic neighbors information among the users, we further provide compactness co-clustering strategy in FPPDM ++ to cluster the users with similar characteristics. Our empirical studies on benchmark datasets demonstrate that FPPDM/ FPPDM ++ significantly outperforms the state-of-the-art models.
6. Basket Representation Learning by Hypergraph Convolution on Repeated Items for Next-basket Recommendation
Yalin Yu, Enneng Yang, Guibing Guo, Linying Jiang, Xingwei Wang
Basket representation plays an important role in the task of next-basket recommendation. However, existing methods generally adopts pooling operations to learn a basket’s representation, from which two critical issues can be identified. First, they treat a basket as a set of items independent and identically distributed. We find that items occurring in the same basket have much higher correlations than those randomly selected by conducting data analysis on a real dataset. Second, although some works have recognized the importance of items repeatedly purchased in multiple baskets, they ignore the correlations among the repeated items in a same basket, whose importance is shown by our data analysis. In this paper, we propose a novel Basket Representation Learning (BRL) model by leveraging the correlations among intra-basket items. Specifically, we first connect all the items (in a basket) as a hyperedge, where the correlations among different items can be well exploited by hypergraph convolution operations. Meanwhile, we also connect all the repeated items in the same basket as a hyperedge, whereby their correlations can be further strengthened. We generate a negative (positive) view of the basket by data augmentation on repeated (non-repeated) items, and apply contrastive learning to force more agreements on repeated items. Finally, experimental results on three real datasets show that our approach performs better than eight baselines in ranking accuracy.
7. Probabilistic Masked Attention Networks for Explainable Sequential Recommendation
Huiyuan Chen, Kaixiong Zhou, Zhimeng Jiang, Michael Yeh, Xiaoting Li, Menghai Pan, Yan Zheng, Xia Hu, Hao Yang
The recently proposed Transformer-based models are highly powerful for modeling temporal dynamics of user preference in sequential recommendation. Most of variants adopt the Softmax transformation in the self-attention layers to generate dense attention probabilities. However, real-world item sequences are often noisy, containing a mixture of true-positive and false-positive interactions. Such dense attentions inevitably assign probability mass to noisy or irrelevant items, leading to sub-optimal performance and poor explainability. To tackle these issues, we propose a Probabilistic Masked Attention Network (PMAN) to identify the sparse pattern of attentions, which is more desirable for pruning noisy items in sequential recommendation. Specifically, we employ a probabilistic mask to achieve sparse attentions under a constrained optimization framework. As such, PMAN allows to select which information is critical to be retained or dropped in a data-driven fashion. Experimental studies on real-world benchmark datasets show that PMAN is able to improve the performance of Transformers significantly, and the performance gain becomes larger for more noisy sequences. Our code and data are available in: https://anonymous.4open.science/r/PMAN_Rec-E72E.
8. Dual Personalization on Federated Recommendation
Chunxu Zhang, Guodong Long, Tianyi Zhou, Peng Yan, Zijian Zhang, Chengqi Zhang, Bo Yang
https://openreview.net/forum?id=8VvQ4SpvZVi
Federated recommendation is a new Internet service architecture that aims to provide privacy-preserving recommendation services in federated settings. Existing solutions are used to combine distributed recommendation algorithms and privacy-preserving mechanisms. Thus it inherently takes the form of heavyweight models at the server and hinders the deployment of on-device intelligent models to end-users. This paper proposes a novel Personalized Federated Recommendation (PFedRec) framework to learn many user-specific lightweight models to be deployed on smart devices rather than a heavyweight model on a server. Moreover, we propose a new dual personalization mechanism to effectively learn fine-grained personalization on both users and items. The overall learning process is formulated into a unified federated optimization framework. Specifically, unlike previous methods that share exactly the same item embeddings across users in a federated system, dual personalization allows mild finetuning of item embeddings for each user to generate user-specific views for item representations which can be integrated into existing federated recommendation methods to gain improvements immediately. Experiments on multiple benchmark datasets have demonstrated the effectiveness of PFedRec and the dual personalization mechanism. Moreover, we provide visualizations and in-depth analysis of the personalization techniques in item embedding, which shed novel insights on the design of recommender systems in federated settings. The code is available.
9. Curriculum Multi-Level Learning for Imbalanced Live-Stream Recommendation
Shuodian Yu, Junqi Jin, Li Ma, Xiaofeng Gao, Xiaopeng WU, Haiyang Xu, Jian Xu
In large-scale live-stream recommendation, streamers are classified into different levels based on their popularity and other metrics for marketing. Several top streamers at the head level occupy a considerable amount of exposure, resulting in an unbalanced data distribution. A unified model for all levels without consideration of imbalance issue can be biased towards head streamers and neglect the conflicts between levels. The lack of inter-level streamer correlations and intra-level streamer characteristics modeling imposes obstacles to estimating the user behaviors. To tackle these challenges, we propose a curriculum multi-level learning framework for imbalanced recommendation. We separate model parameters into shared and level-specific ones to explore the generality among all levels and discrepancy for each level respectively. The level-aware gradient descent and a curriculum sampling scheduler are designed to capture the de-biased commonalities from all levels as the shared parameters. During the specific parameters training, the hardness-aware learning rate and an adaptor are proposed to dynamically balance the training process. Finally, shared and specific parameters are combined to be the final model weights and learned in a cooperative training framework. Extensive experiments on a live-stream production dataset demonstrate the superiority of the proposed framework.
10. Discriminative-Invariant Representation Learning for Unbiased Recommendation
Hang Pan, Jiawei Chen, Fuli Feng, Wentao Shi, junkang Wu, Xiangnan He
Selection bias hinders recommendation models from learning unbiased user preference. Recent works empirically reveal that pursuing invariant user and item representation across biased and unbiased data is crucial for counteracting selection bias. However, our theoretical analysis reveals that simply optimizing representation invariance is insufficient for addressing the selection bias — recommendation performance is bounded by both representation invariance and discriminability. Worse still, current invariant representation learning methods in recommendation neglect even hurt the representation discriminability due to data sparsity and label shift. In this light, we propose a new Discriminative-Invariant Representation Learning framework for unbiased recommendation, which incorporates label-conditional clustering and prior-guided contrasting into conventional invariant representation learning to mitigate the impact of data sparsity and label shift, respectively. We conduct extensive experiments on three real-world datasets, validating the rationality and effectiveness of the proposed framework.
11. Denoised Self-Augmented Learning for Social Recommendation
Tianle Wang, Chao Huang, Lianghao Xia
Social recommendation has been increasingly investigated in a broad spectrum of online applications (e.g., e-commerce, online streaming) to leverage social information for help user-item interaction modeling. Recently, Self-Supervised Learning (SSL) has been outstandingly successful in alleviating data sparsity with the augmented learning tasks. Inspired by this, recent attempts bring the benefits of SSL into social recommendation by supplementing the main supervised task with social-aware self-supervised signals. However, social information is unavoidably noisy for characterizing user preference, due to the ubiquitous presence of interest-irrelevant social connections, e.g., colleagues or classmates who do not share many common interests. To rectify this, we propose a new social recommender with a Denoised Cross-view Self-Augmented Learning paradigm (DSAL). It not only preserves the helpful social relations for enhancing user-item interaction modeling, but also allows the personalized cross-view knowledge transfer with adaptive semantic alignment in embedding space. Experimental results on various recommendation benchmarks verify the advantages of our DSAL over state-of-the-art methods.
12. Intent-aware Recommendation via Disentangled Graph Contrastive Learning
Yuling Wang, Xiao Wang, Xiangzhou Huang, yanhua yu, Haoyang Li, Mengdi Zhang, Zirui Guo, Wei Wu
Graph neural network (GNN) based recommender systems have become one of the mainstream trends due to the powerful learning ability from user behavior data. Understanding the user intents from behavior data is the key to recommender systems, which poses two basic requirements for GNN-based recommender systems. One is how to learn complex and diverse intents especially when the user behavior is usually inadequate in reality. The other is different behaviors have different intent distributions, so how to establish their relations for a more explainable recommender system. In this paper, we present the Intent-aware Recommendation via Disentangled Graph Contrastive Learning (IDCL), which simultaneously learns interpretable intents and behavior distributions over those intents. Specifically, we first model the user behavior data as a user-item-concept graph, and design a GNN based behavior disentangling module to learn the different intents. Then we propose the intent-wise contrastive learning to enhance the intent disentangling and meanwhile infer the behavior distributions. Finally, the coding rate reduction regularization is introduced to make the behaviors of different intents orthogonal. Extensive experiments demonstrate the effectiveness of IDCL in terms of substantial improvement and the interpretability.
Special Track on AI for Good
13. GreenFlow: A Computation Allocation Framework for Building Environmentally Sound Recommendation System
Xingyu Lu, Zhining Liu, Yanchu Guan, Hongxuan Zhang, Chenyi Zhuang, Ma Wenqi, Yize Tan, Jinjie Gu, Guannan Zhang
14. Toward Job Recommendation For All
Guillaume Bied, Solal Nathan, Elia Perennes, Morgane Hoffmann, Philippe Caillou, Bruno Crépon, Christophe Gaillac, Michele Sebag
15. Keeping people active and healthy at home using a Reinforcement Learning-based fitness recommendation framework
Elias Tragos, Diarmuid O’Reilly-Morgan, JAMES GERACI, Bichen Shi, Barry Smyth, Cailbhe Doherty, Aonghus Lawlor, Neil Hurley
Demonstrations Track
16. SupervisorBot: NLP-Annotated Real-Time Recommendations of Psychotherapy Treatment Strategies with Deep Reinforcement Learning
Baihan Lin, Guillermo Cecchi, Djallel Bouneffouf
更多论文可参考官网链接:
https://ijcai-23.org/main-track-accepted-papers/
这篇关于IJCAI2023推荐系统相关论文集锦的文章就介绍到这儿,希望我们推荐的文章对编程师们有所帮助!